Top Data Analysis Programs for Better Decision-Making
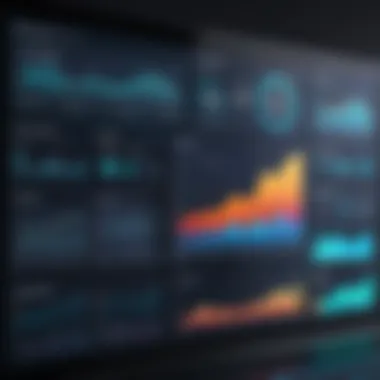
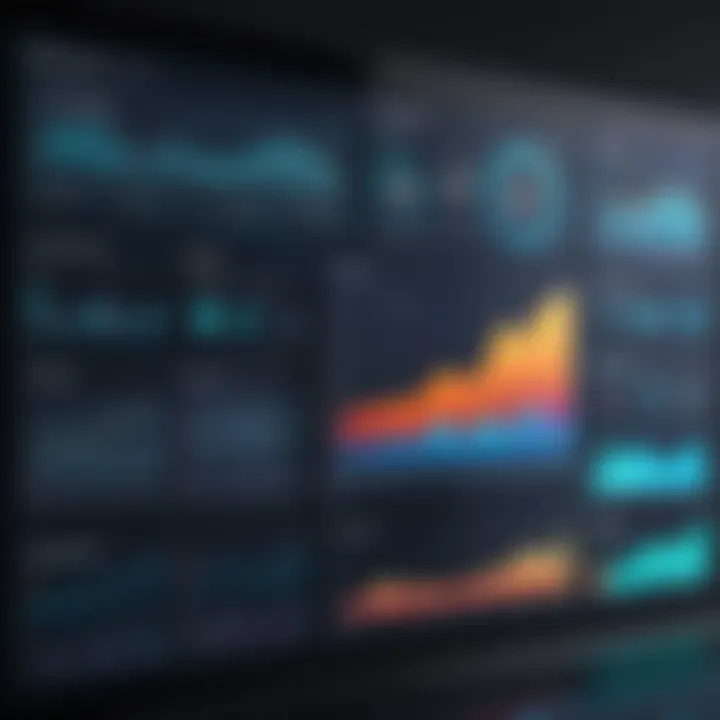
Intro
Data is the lifeblood of decision making in today's organizational landscape. With organizations increasingly driven by analytics, the choice of data analysis programs has never been more crucial. The ongoing evolution within the realm of data analysis tools is fascinating and essential for professionals, from marketing gurus to scientific researchers, who rely on data to provide insight and inform strategies. The right software can mean the difference between clarity and confusion, informed decisions and shot-in-the-dark guesses. Thus, navigating the labyrinth of available options is vital for success.
This guide aims to serve as a trusty compass for organizations seeking to enhance their analytical capabilities. We will explore the features, usability, and reasonably glaring gaps that can work in your favor or against it, while introducing a selection of top-tier software programs. Let’s embark on this journey to empower your data-driven outcomes.
Foreword to Data Analysis Programs
In the modern landscape of decision-making, data analysis has become an indispensable tool for organizations and professionals alike. Understanding data is not just about crunching numbers; it's about gaining valuable insights that drive strategic choices, enhance productivity, and ultimately shape the future of businesses. By leveraging the right data analysis programs, one can transform raw data into actionable intelligence that informs everything from marketing strategies to risk management.
Importance of Data Analysis in Today’s World
Data is often described as the new oil. Just like oil powered the industrial age, data fuels the digital age. It allows businesses to understand their customers, predict trends, and optimize their operations. Companies that effectively harness data analysis can stay a step ahead of competitors, ultimately leading to increased market share.
For instance, consider a retail company that utilizes data analytics to track shopping habits. By identifying patterns in consumer behavior, such as peak shopping times or popular products, the company can tailor its marketing strategies and improve inventory management. This not only enhances customer satisfaction but can also significantly impact the bottom line.
"In today's world, data analysis isn't just beneficial; it's crucial for survival in competitive markets."
Furthermore, the integration of data analysis into day-to-day operations can empower teams across various departments. From sales to customer service, having access to relevant data allows for quick, informed decisions that align with the overall business strategy. In short, the implications of effective data analysis reach far beyond just numbers; they can dictate the trajectory of an organization.
Criteria for Evaluating Data Analysis Software
Choosing the right data analysis software can feel like looking for a needle in a haystack. There are myriad options available, each with its unique features, strengths, and weaknesses. Thus, identifying the appropriate software involves evaluating a set of specific criteria:
- Usability: Software should be user-friendly, allowing easy navigation without extensive training. If the learning curve is steep, it might deter team members from using it effectively.
- Functionality: Does it provide the necessary tools for your analytical needs? Consider features such as data visualization, integration capabilities, and statistical analysis functions.
- Scalability: As the organization grows, so do its data needs. The software should be able to handle increasing data volumes and complexity.
- Cost: Compare pricing against the value provided. Sometimes, the most expensive option isn’t necessarily the best for your specific use-case.
- Support and Community: Consider the level of customer service available and the community support around the software. A responsive support system can make all the difference in troubleshooting.
Navigating these considerations effectively can aid decision-makers in selecting an analysis program that aligns with their organization's goals. With a plethora of tools available, it’s sensible to approach the selection process systematically, ensuring that it meets the diverse needs of stakeholders and accelerates data-driven decision-making.
Overview of Leading Data Analysis Programs
In the vast sea of data that organizations navigate today, understanding the landscape of data analysis programs is essential. These tools serve not only as methodologies for processing numbers but as catalysts for insights that drive strategic decisions. By analyzing trends, patterns, and anomalies, data analysis software enables businesses to make informed choices, ultimately impacting their bottom line. This section provides a thorough overview of the current leading data analysis programs, reflecting the diverse needs of users ranging from novice analysts to seasoned data scientists. Choosing the right program isn't just a technical decision—it can determine the trajectory of a project.
Commercial Software Solutions
Commercial software has long been the backbone of data analysis in the corporate world. Programs like Microsoft Excel and SAS provide extensive functionalities tailored to a plethora of analytical needs. Businesses in regulated industries often gravitate towards these well-established solutions due to their reliability and customer support. These commercial offerings typically include:
- User-friendly interfaces that simplify complex tasks
- Robust customer service and support, making troubleshooting easier
- Regular updates that enhance performance and introduce new features
However, while commercial solutions can come with hefty price tags, the investment often pays off through improved productivity and data accuracy. Companies have reported that the accessibility and functionality of these software programs empower them to turn raw data into actionable insights with minimal fuss. As businesses strive to maintain their competitive edge, the reliability of commercial data analysis tools remains a top consideration for decision-makers.
Open Source Alternatives
For those seeking flexibility and customization, open-source software provides an enticing alternative. Programs like R and Python, paired with libraries such as NumPy and Pandas, offer extensive capabilities at little to no cost. The beauty of open-source tools lies in their adaptability; experienced users can tailor the software to better fit their specific analytical needs.
- Benefits of Open Source Solutions Include:
- Lower costs compared to commercial software
- A vast community of developers contributing to constant improvements
- Highly flexible with the ability to integrate various coding packages
Nevertheless, organizations might face challenges, such as the steep learning curve associated with software like R, especially for those who lack a coding background. Consequently, while the flexibility of open-source programs is undeniable, decision-makers must consider the expertise available within their teams before opting for these solutions.
Cloud-Based Platforms
With the rise of remote work, cloud-based data analysis tools have surged in popularity. Platforms like Google Data Studio and Microsoft Power BI are at the forefront of this transition. They provide real-time data collaboration that few traditional solutions can match. The ability to access analytical tools from virtually anywhere enhances not just individual productivity but team-based data efforts.This flexibility increases engagement and ensures timely decision-making.
- Key Features of Cloud-Based Platforms:
- Accessibility from any device with an internet connection
- Collaboration features that support teamwork on shared data analysis
- Regular updates without requiring users to manage installations
Despite these advantages, cloud solutions also come with considerations, such as data security and potential dependency on internet access. Decision-makers must weigh these factors against the productivity gains to determine whether to fully embrace this modern approach.
In summary, understanding the realm of data analysis programs from commercial and open-source solutions to cloud offerings is crucial. Each category presents unique strengths tailored to different organizational needs and challenges.
In-Depth Analysis of Top Commercial Programs
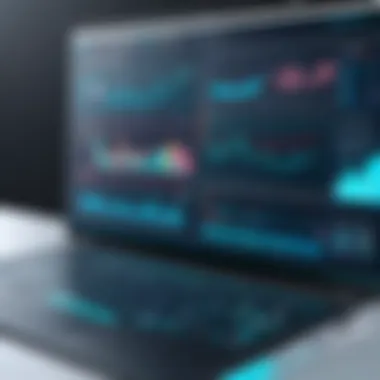
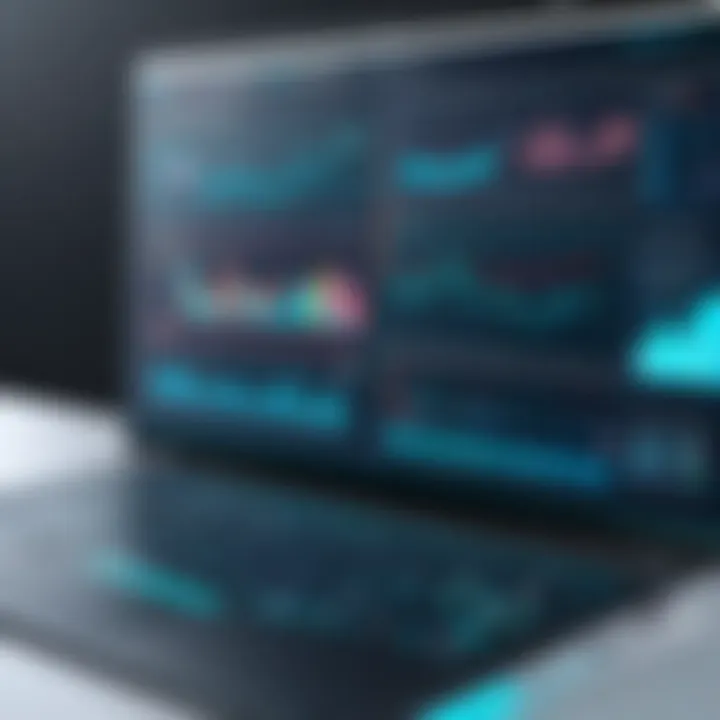
Data analysis programs have become crucial in extracting meaningful insights from the plethora of data generated daily. Diving into an in-depth analysis of top commercial programs shines a light not only on their features and capabilities but also on their practical applications in various industries. This analysis reveals how each program serves different analytical needs, which can help decision-makers make informed choices based on their unique situations.
The commercial software landscape is riddled with options, and understanding what each brings to the table is vital for organizations aiming to leverage data for strategic advantage. This section will dissect leading programs like Microsoft Excel, Tableau, SAS, and IBM SPSS, revealing their unique strengths and the specific considerations one should be aware of when selecting the right tool.
Microsoft Excel: The Ubiquitous Choice
Microsoft Excel remains a stalwart in the data analysis realm. Its familiarity and ease of use have cemented its status as the go-to tool for many professionals, making it seem almost inevitable in discussing data programs. Excel facilitates basic data entry, calculations, and simple graphing functions, which can be elaborated upon with advanced features like PivotTables or macros.
However, there’s more to Excel than just being user-friendly. Its widespread availability ensures that most teams have at least a fundamental proficiency in it. Gartner calls it "the world’s most popular business intelligence tool," and it’s hard to argue against that. With its support for various data sources through Power Query, Excel can seamlessly integrate with databases, making it a versatile option despite its limitations in handling very large datasets compared to more specialized programs.
"For those who want a foundation to build upon, Excel is a reliable starting point, but expanding knowledge to more specialized software can yield greater insights."
Tableau: Visual Analytics at its Best
Next, if one were to highlight a program that thrives on visualization, Tableau would undoubtedly take the crown. Tableau transforms complex data into colorful, easy-to-understand visualizations that tell a story in a glance. Its drag-and-drop functionality makes it accessible to users, reducing the learning curve significantly.
In addition to being visually appealing, Tableau caters to various business needs. Use it to create dashboards that summarize performance metrics or perform detailed analysis with queries tailored for specific operational requirements. The ability to connect a multitude of data sources—from spreadsheets to cloud databases—ensures that whatever your data needs may be, Tableau has you covered.
SAS: Advanced Statistical Analysis
When it comes to comprehensive statistical analysis, SAS stands in a league of its own. This program is well-known for its powerful analytics, data management capabilities, and advanced statistical procedures. Organizations often rely on SAS for predictive analytics, ensuring that decisions are data-backed.
Moreover, SAS's extensive range of tools for statistical modeling, forecasting, and data integration makes it suitable for industries like healthcare, finance, and manufacturing. It can handle vast datasets, making insights actionable amidst complexities. However, a common criticism involves its cost and the steeper learning curve compared to its more user-friendly counterparts.
IBM SPSS: Robust Predictive Analytics
IBM SPSS is synonymous with predictive analytics. It’s extensively used in academia, government, and business sectors alike for its strong statistical capabilities. SPSS specializes in complex multivariate analysis, offering users a suite that includes regression analysis, cluster analysis, and even option for machine learning techniques.
The software embodies a user-friendly interface that still manages to accommodate the more advanced analytical needs without overwhelming the user, which can certainly be appreciated by such diverse user groups. Though it may not offer the same level of visualization prowess as Tableau, its robust statistical abilities make it a core asset in any analyst's toolkit.
Exploring Open Source Data Analysis Tools
Open source data analysis tools have become indispensable in the modern analytical landscape. These programs not only present a cost-effective alternative to their commercial counterparts but also foster a community-driven approach to software development. This means that users can benefit from a constant stream of updates, enhancements, and a wealth of resources shared among users. The flexibility of open source tools allows organizations and individuals to tailor solutions to their specific needs, enhancing the effectiveness of their data analysis processes.
R: The Statistician's Secret Weapon
R is renowned for its extensive statistcal capabilities and is often regarded as a go-to tool for statisticians and data analysts. It's not just about raw data crunching; R shines in data visualization and advanced statistical modeling. One of its most lauded features is the ability to create high-quality graphs and charts, a crucial aspect of data analysis for communicating results effectively. R is also supported by a massive repository of packages—over 15,000 available on CRAN—allowing users to extend its functionality for virtually any analytical need.
Python and Data Analysis Libraries
Python has emerged as one of the most popular programming languages for data analysis, thanks in large part to its versatility and the wealth of libraries that enable data manipulation, visualization, and analysis. Below are three key libraries that have solidified Python's standing in this domain.
NumPy
NumPy is the backbone of numerical computing in Python. It provides support for arrays and matrices, as well as a collection of mathematical functions. This library is invaluable for anyone delving into data analysis or scientific computing. Its speed and performance, due to vectorization, enable users to conduct complex calculations with ease and efficiency. One standout feature is the ability to perform operations on entire arrays without writing explicit loops, which often leads to cleaner and more readable code. While NumPy is generally regarded as a powerful tool, its learning curve can be steep for beginners who may find the initial setup a bit daunting.
Pandas
Pandas is another cornerstone of Python's data analysis capabilities, designed specifically for data manipulation and analysis. It introduces two primary data structures—Series and DataFrames, making it remarkably easy to handle and analyze structured data. One of its most compelling aspects is the ability to work with time series data, enabling financial analysts and researchers to perform analyses that track trends over time. Moreover, its rich set of functions for merging, reshaping, and cleaning datasets is a game changer for anyone dealing with messy data. However, it can take some time to fully grasp the intricacies of indexing and data selection, which can lead to occasional confusion among novice users.
Matplotlib
When it comes to data visualization, Matplotlib stands out as a critical tool. This plotting library offers extensive customization options for generating a wide range of static, animated, and interactive plots in Python. Matplotlib enables analysts to create publication-quality graphs, facilitating data storytelling through visuals. With its simple interface, users can start generating graphs and visualize their data almost immediately. However, the detailed customization can sometimes be overwhelming, as it requires a good understanding of various parameters to fine-tune the appearance of the plots.
Apache OpenOffice Calc: A Worthy Competitor
Another significant player in the open source realm is Apache OpenOffice Calc. Primarily viewed as a spreadsheet program, Calc provides a surprisingly robust suite of tools for data analysis. Similar in function to Microsoft Excel, it allows users to perform data calculations, create graphs, and manage databases with ease. Its familiarity makes it accessible for those who might be hesitant to dive into more complex programming environments. However, while it may lack some advanced features found in other specialized data analysis tools, Calc offers a solid foundation for basic to intermediate level data analysis tasks, making it a worthy option for those on a budget.
In summary, exploring open source data analysis tools opens up a wealth of possibilities. Whether one opts for R's analytics prowess or Python's rich libraries, these tools provide the flexibility and community support needed to tackle today’s analytical challenges.
Evaluating Cloud-Based Data Analysis Solutions
In today’s fast-paced digital landscape, cloud-based data analysis solutions have become indispensable for organizations aiming to harness the power of data. These platforms not only offer extensive functionalities but also provide various advantages such as accessibility, scalability, and cost-effectiveness. As businesses increasingly rely on data for decision-making, understanding the nuances of these tools is paramount. Selecting the right cloud-based solution can have significant implications for operational efficiency and strategic insight. Thus, evaluating such programs requires a systematic approach to thoroughly analyze their capabilities and ease of use.
When diving into the cloud-based realm, professionals must consider several specific elements and benefits:
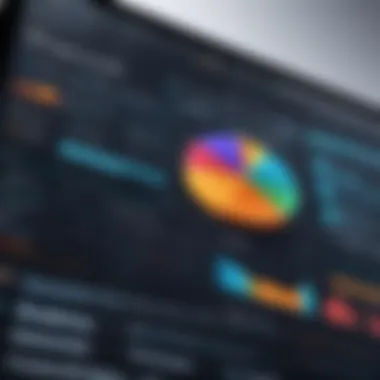
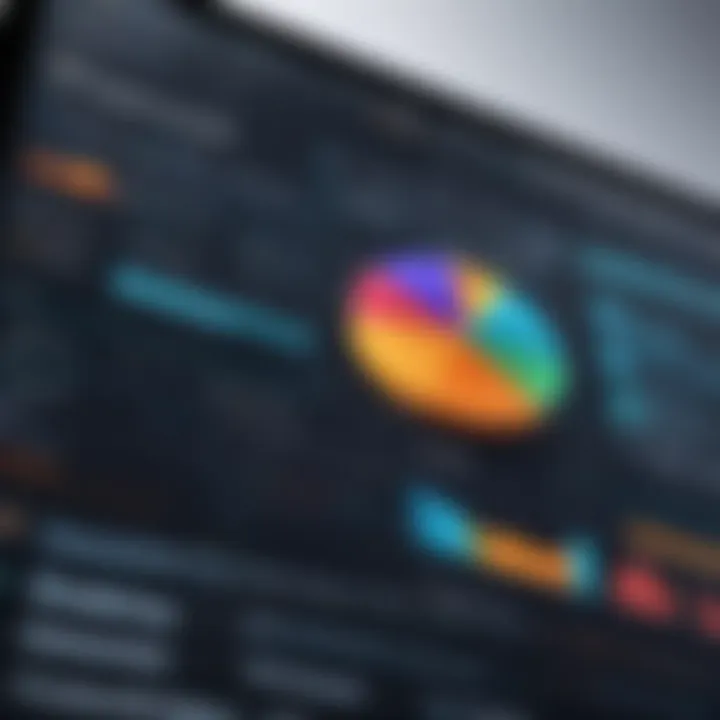
- Accessibility: Cloud solutions allow users to access data from anywhere, anytime. This flexibility enables collaboration across teams and locations, streamlining workflows and ensuring timely insights.
- Scalability: Businesses can easily scale their data analysis capabilities up or down, aligning with their evolving needs without heavy investments in infrastructure.
- Cost-Effectiveness: Many cloud-based options operate on subscription models, which can mitigate upfront costs compared to traditional software licenses.
- Data Security and Compliance: It's crucial to evaluate how well a platform protects user data and adheres to industry regulations, especially with increasing concerns about cybersecurity.
- User Support and Community: A strong support network and active user communities can greatly enhance the overall user experience.
Ultimately, as organizations weigh their choices, understanding the specific features and values that these cloud-based solutions offer becomes essential.
Google Data Studio: The Collaborative Tool
Good ol' Google Data Studio has come to be recognized as a major player in the cloud analytics arena. This tool allows teams to work together like a well-oiled machine, crafting dashboards and reports that visualize data with finesse. Its integration with other Google services is a cherry on top, making data flows smoother for those already invested in the Google ecosystem.
Key features include:
- Drag-and-Drop Interface: Simplifies the design process — no coding skill needed!
- Real-Time Collaboration: Multiple users can work on a report simultaneously.
- Data Connections: Direct connections to a variety of sources, including Google Sheets and Google Analytics.
The cost? Zero dollars. It's free for users, but those awfully comfortable with performance might hit a snag with larger datasets.
Microsoft Power BI: Business Insights Simplified
Moving on to Microsoft Power BI, this program is like having a powerful analytical guru at your fingertips. Designed for business analytics, it helps organizations convert raw data into meaningful insights. The interplay with Microsoft Office products fosters a familiar environment, making it easier for users.
Its standout capabilities are:
- Interactive Dashboards: Users can create stunning displays with live data updates.
- Robust Analytics: Tools for deep-dive analyses, helping to uncover trends.
- Integration with Azure: Further enhances capabilities, especially for users already on Microsoft’s cloud.
However, the learning curve can be a bit steep for first-time users.
AWS QuickSight: Scalable Analytics in the Cloud
AWS QuickSight serves as Amazon's answer to cloud analytics. This service prides itself on delivering fast insights from any dataset. It’s particularly praised for its scalability, which means businesses can grow their analysis capabilities right along with their data needs.
Highpoints include:
- Pay-Per-Session Pricing: Ensures costs align with usage — no more paying for what you don't use.
- Built-In Machine Learning: Integrated ML features for advanced analytics without needing a specialist.
- Secure Sharing: Data visualization can be securely shared across the organization without compromising sensitive information.
One consideration, though, is its initial setup. Navigating AWS can be a bit labyrinthine.
Looker: Data-Driven Decision-Making
Lastly, Looker is a notable cloud-based solution that emphasizes data-driven decision-making. This sleek platform looks to reshape how businesses approach their data by providing a unified platform for analytics and business intelligence. Its unique modeling language is a standout feature, allowing for customized data manipulations suited to organizational needs.
Highlights include:
- Unified Data Platform: Ensures various data sources can be seamlessly analyzed together.
- User-Friendly Interface: Offers an intuitive layout, making it easier for teams to dive into insights without prior training.
- Modern Architecture: Built for the cloud, which means it can evolve quickly as the market changes.
However, it may not be a fit for smaller organizations where its pricing might take a bigger bite out of the budget.
Ultimately, evaluating cloud-based data analysis solutions is not just about selecting a product but aligning it with organizational goals and data strategies. Understanding each tool's unique offerings will empower decision-makers to make informed, strategic choices.
Considerations for Choosing the Right Program
When it comes to selecting data analysis software, making an informed decision is paramount. The right program can not only streamline your workflow but also elevate the quality of insights you can derive from your data. However, navigating the crowded landscape of options requires a systematic approach—hence the significance of considering various factors before committing to a choice.
Assessing User Requirements
Understanding user requirements is the cornerstone of effective software selection. What features are essential for your team? Do you need advanced statistical tools, or is data visualization more critical? Different use cases demand different functionalities. For instance, if your team is mostly analyzing financial data, software with strong forecasting capabilities, like IBM SPSS, may serve you well. On the other hand, for marketing analytics, a user-friendly platform like Google Data Studio might do the trick—its strengths lie in intuitive visualizations and ease of sharing.
It’s helpful to engage with the actual users who will be working with the software. Conduct surveys or hold focus groups to determine their needs and preferences. Furthermore, create a checklist of must-have features to ensure no vital aspect is overlooked.
Integration with Existing Systems
Another critical consideration is ensuring seamless integration with your current systems. Many organizations use a mishmash of software tools—from CRM platforms to various databases. If new software cannot play nicely with what you already have, it can lead to data silos and inefficiencies.
When evaluating potential programs, ask yourself:
- How well does the program integrate with existing tools?
- Are there APIs available for custom integrations?
- Will training be necessary for a smooth transition?
For example, Microsoft Power BI is known for its compatibility with other Microsoft products, but the same might not hold true for less mainstream software. Remember, the goal is to achieve a cohesive ecosystem that allows for the free flow of information across platforms.
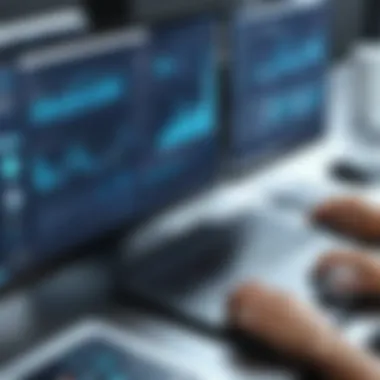
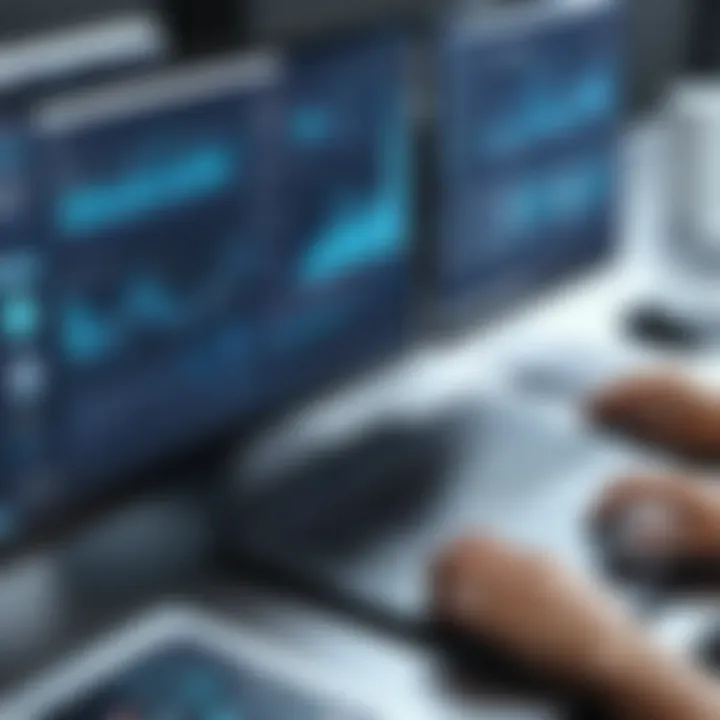
Cost vs. Benefit Analysis
Finally, a meticulous cost versus benefit analysis often makes the difference between a sound investment and a waste of resources. While it might be tempting to opt for the cheapest option available, doing so may backfire in the long run. Software that comes with a higher price tag may offer robust features and support that cheaper alternatives lack.
Consider these aspects in your analysis:
- Licensing costs: Is it subscription-based or a one-time purchase?
- Hidden fees: Are there additional costs for support or updates?
- ROI: How much improvement in productivity or accuracy do you expect?
In short, weigh the overall value offered by a program against its pricing structure. Doing this thoughtfully will ensure that you're not caught between the devil and the deep blue sea when budgeting for new software.
"The key to making sound software choices lies not just in the features, but in a thorough understanding of your specific needs and existing workflows."
Future Trends in Data Analysis Software
The landscape of data analysis is continually morphing, driven by tech advancements and varying user needs. The emphasis on future trends isn’t just for those curious about what’s next; it’s essential for decision-makers and IT professionals to stay ahead of the curve. Understanding these emerging trends helps organizations not only choose the right tools but also prepares them for the challenges and opportunities ahead that may influence their strategy.
Machine Learning Influence
Machine learning has come a long way from being just a buzzword. It's weaving itself into every nook and cranny of data analysis programs. These systems are developing the ability to learn from data patterns without explicit programming, making them more adept at making predictions. By automating complex analytical tasks, businesses can save time and resources.
Moreover, tools like Python, combined with libraries such as TensorFlow and Scikit-learn, are enabling powerhouses of analytics like Google and Facebook to refine their predictive models. In the realm of practical application, this means that programs can quickly highlight anomalies or trends in huge datasets, something that was once a labor-intensive task.
To illustrate, consider a retail company using machine learning algorithms to analyze customer purchasing patterns. This technology can identify seasonal trends or forecast demand for specific products. The insights gained can lead to smarter inventory management and better marketing strategies, ultimately enhancing the bottom line.
Enhanced Data Visualization Techniques
As data proliferates, the ability to visualize this information is growing increasingly important. Enhanced data visualization techniques help clarify complex information, helping decision-makers digest critical insights at a glance. Tools like Tableau and Microsoft Power BI are on the cutting edge of this movement, adopting features that not only present statistics but tell compelling stories.
Key aspects of these visualization techniques include:
- Interactivity: Users can click, hover, and drill down into the data to extract more meaningful insights.
- Real-Time Updates: Solutions that reflect real-time data ensure that decisions are based on the freshest intelligence available.
- Integration with AI: Some programs now employ artificial intelligence to recommend charts or formats that best represent the underlying data.
"Effective visualization transforms raw data into insight. It’s not just about making things pretty; it’s about making it understandable."
Such advancements in visualization technologies facilitate greater engagement with data, leading to more informed decision-making processes.
Focusing on User Experience and Accessibility
User experience (UX) and accessibility have become focal points in the development of data analysis software. As teams become increasingly diverse, ensuring that tools are accessible to all members—regardless of their technical expertise—is vital. Programs that prioritize usability allow non-technical staff to derive valuable insights without depending heavily on specialized knowledge.
Some considerations for enhancing user experience and accessibility might embrace:
- Intuitive Interfaces: Simplified designs that minimize complexity encourage user engagement and reduce the learning curve.
- Mobile Compatibility: As work becomes more flexible, having mobile-friendly interfaces enables users to access critical analytics on-the-go.
- Customizable Dashboards: Users can tailor their workspace to fit their specific needs, focusing on metrics that matter most to them easily.
By making data analysis tools more accessible, organizations can democratize data insights across various departments. When everyone can engage with data, the organization as a whole benefits from more collaborative and informed decision-making.
End and Recommendations
In the constantly evolving landscape of data analysis, wrapping up with thoughtful conclusions and actionable recommendations is vital. The choices surrounding data analysis programs can be bewildering, given the myriad of options available. A structured conclusion helps distill the insights gleaned throughout this guide, offering a roadmap to navigate the cluttered field.
Navigating Software Choices
When it comes to selecting a data analysis program, a clear-eyed assessment based on specific needs is crucial. Understandably, each business is unique. Factors like data volume, the complexity of analysis required, and user expertise should steer your path. For instance, if fast, user-friendly visuals are at the top of your list, Tableau might be the golden goose. Conversely, if deep statistical analysis is your bread and butter, SAS could hit the sweet spot.
A few considerations to keep in mind:
- User Experience: The interface should make sense to your team, as a steep learning curve can drive productivity down.
- Compatibility: Ensure the software meshes well with your existing systems—no one wants to add stress at integration time.
- Scalability: Can the chosen solution grow alongside your data needs? Flexibility can be a game-changer.
In the end, identifying the right alignment between user needs and program capabilities is essential for maximizing data-driven outcomes.
Keeping Pace with Evolving Technologies
Technology is like a fast river—always flowing and often unpredictable. Staying updated on the latest trends in data analysis can help ensure that your organization doesn't get swept away. Emerging technologies like machine learning and advanced data visualization techniques promise to enhance analytical capabilities significantly.
One important aspect is the integration of machine learning algorithms into data analysis software. This enables predictive analytics that can provide valuable foresight for businesses, turning historical data into actionable insights for future decision-making. The benefit? It helps businesses stay one step ahead in a competitive market.
Moreover, user experience and accessibility remain at the forefront of software development. Vendors are increasingly focusing on intuitive designs that allow even the non-technical users to tap into sophisticated analytical power. Hence, leveraging software that caters to a broader audience can foster a data-driven culture within your organization.
"Embracing change and evolving technologies lays the groundwork for the future of data-driven success."
Ultimately, keeping a watchful eye on these trends is not just a matter of preference but a necessity in today’s data-centric world. Staying informed allows organizations to pivot when needed and remain leaders in their industries.