The Ultimate Guide to Top Data Analytics Software
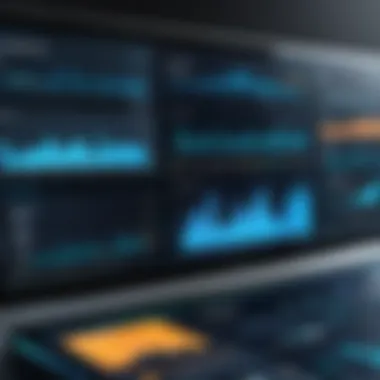
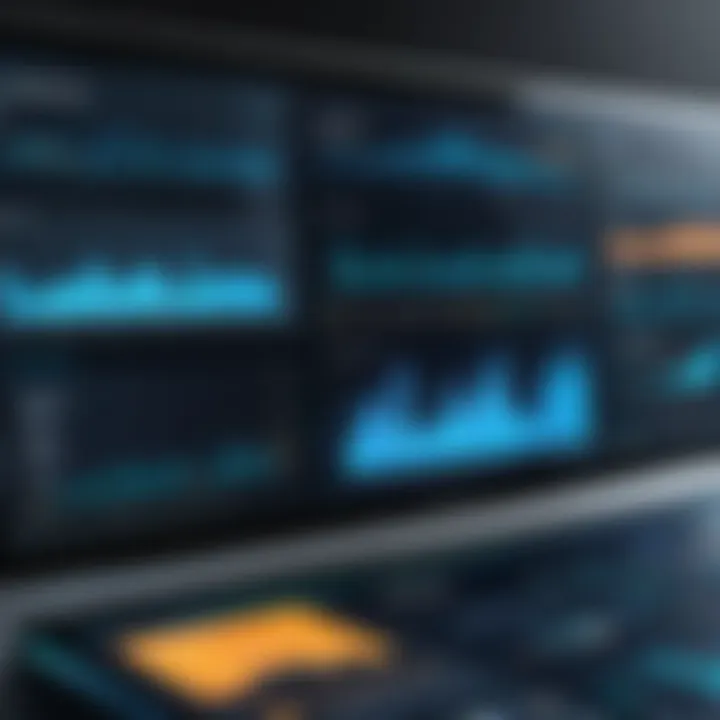
Intro
In recent years, the realm of data analytics has evolved tremendously. The exponential growth of data generated from various sources necessitates robust software to extract meaningful insights. Selecting the right data analytics software is crucial for professionals and organizations aiming to harness the power of data effectively. This guide seeks to illuminate the key features, benefits, and latest offerings in the data analytics software market, enabling informed decisions for businesses, IT professionals, and entrepreneurs alike.
Industry Overview
The data analytics software market is vast, characterized by rapid growth and innovation. As enterprises recognize the significant value of data insights, the demand for advanced analytics tools has surged. Here are several notable aspects of the current landscape.
Current Trends in the Industry-specific Software Market
More businesses are adopting data-driven decisions. Key trends include:
- Increased focus on real-time analytics.
- Growth of self-service analytics to enable non-technical users.
- Importance of cloud-based solutions for scalability and accessibility.
Key Challenges Faced by Buyers in the Industry
Despite the growth in available tools, buyers often face challenges such as:
- Difficulty in identifying the right solution fit for their unique needs.
- Overwhelming choices leading to decision fatigue.
- Integration hurdles with existing systems.
Emerging Technologies Impacting the Industry
Technologies reshaping data analytics include:
- Artificial Intelligence for predictive analytics.
- Machine Learning for enhanced data pattern recognition.
- Augmented Analytics for automated data preparation and insight discovery.
Top Software Options
Exploring leading software options is essential for optimizing analytics efforts.
Review of Leading Software Providers in the Industry
Some notable providers include:
- Tableau – Known for its powerful visualization capabilities.
- Microsoft Power BI – Offers seamless integration with other Microsoft products.
- QlikView – Provides in-memory data processing for swift analysis.
Feature Comparison of Top Software Solutions
A comprehensive look at related features might entail:
- Data visualization tools to present insights clearly.
- User-friendly dashboards for easy navigation.
- Support for multiple data sources for comprehensive analysis.
Pricing Structures of Different Software Options
Pricing can vary significantly. Some offer subscription models, while others have perpetual licenses. Users must assess costs against their budget constraints and required functionalities.
Selection Criteria
Choosing the right software requires keen evaluation of several factors.
Important Factors to Consider When Choosing Software
Key considerations include:
- Scalability to accommodate future growth.
- Usability for non-technical team members.
- Integration capabilities for existing tools and platforms.
Common Mistakes to Avoid During the Selection Process
Avoid pitfalls such as:
- Focusing solely on features rather than on how they meet business goals.
- Neglecting the importance of vendor support and updates.
- Underestimating the training required for effective use.
How to Determine the Right Fit for Your Business Needs
Assessment of specific organizational needs is critical. Conducting a thorough needs analysis can reveal essential functionalities that should be prioritized in software selection.
Implementation Strategies
Once software is selected, effective implementation is crucial for success.
Best Practices for Smooth Implementation of Software Solutions
- Develop a timeline with clear milestones.
- Engage stakeholders at every step to ensure buy-in.
- Allocate adequate resources for implementation tasks.
Integration with Existing Systems and Processes
Smooth integration can minimize disruptions. Evaluation of current systems will reveal how the new solution can fit seamlessly in existing workflows.
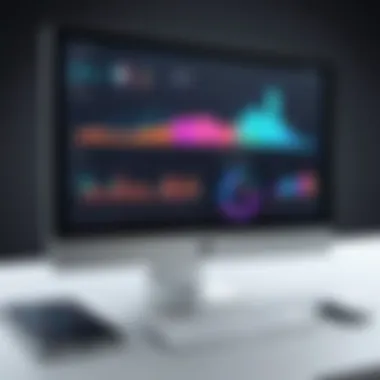
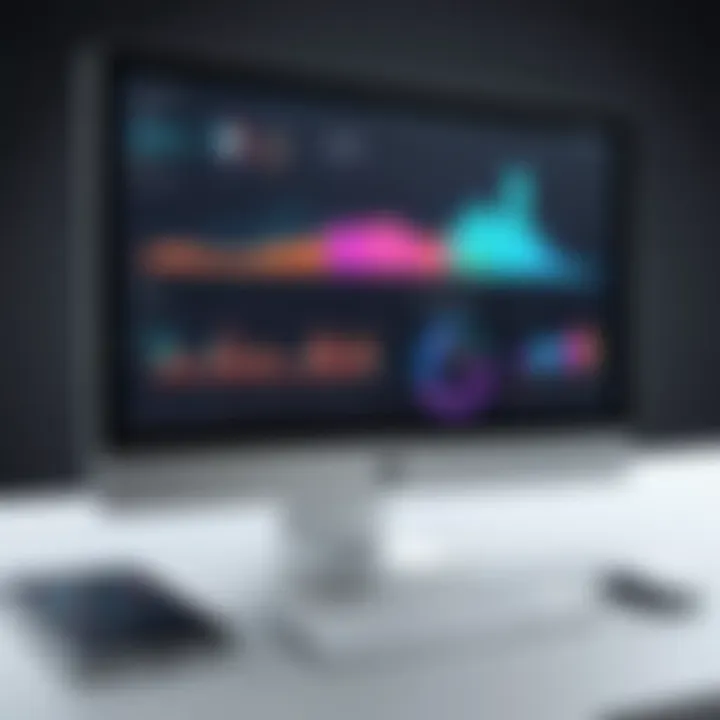
Training and Support Resources for Successful Deployment
Providing training resources is essential for user adoption. Vendors often offer tutorials, webinars, and documentation to support users during the early stages.
Future Trends
Understanding future trends can guide your investment in analytics tools.
Predictions for the Future of the Industry-specific Software Landscape
The emphasis will likely shift towards automation and real-time insights. Analytics will become more ubiquitous across all sectors.
Upcoming Technologies Shaping the Industry
Emerging technologies like quantum computing promise enhanced analysis capabilities. As they integrate into mainstream software, they could redefine efficiency in data analytics.
Recommendations for Future-Proofing Your Technology Stack
To remain competitive, businesses should:
- Regularly update and review their analytics capabilities.
- Invest in scalable platforms that can grow with their needs.
- Stay informed about industry advancements and be agile in adopting new solutions.
By strategically choosing and implementing data analytics software, businesses can greatly enhance their decision-making capabilities and operational efficiencies.
Prelims to Data Analytics Software
Data analytics software plays a crucial role in the modern digital landscape. As businesses increasingly depend on data-driven decision-making, understanding this software's scope and functionality is vital. This section illustrates how analytics software transforms raw data into actionable insights.
Defining Data Analytics Software
Data analytics software refers to tools and applications that allow users to collect, process, and analyze data. These tools can range from simple spreadsheet functions to advanced algorithms and machine learning models. The primary aim of such software is to generate insights that drive business improvement. Features typically include data integration, statistical analysis, and reporting capabilities. As organizations navigate vast datasets, defining the software becomes crucial for operational effectiveness.
Importance of Data Analytics in Business
The role of data analytics in business cannot be overstated. It enables organizations to identify patterns, trends, and anomalies which may not be apparent through conventional reviews.
- Improved Decision Making: Data analytics empowers decision-makers by presenting them with well-structured information. This leads to better strategies and operational efficiencies.
- Customer Insights: Understanding customer behavior through analytics helps tailor offerings to meet their needs, enhancing customer satisfaction and loyalty.
- Competitive Advantage: Businesses that adopt advanced analytics gain an edge over competitors. The ability to swiftly analyze market trends and adapt accordingly fosters agility.
"In an era of information overload, the ability to distill meaningful insights from data is invaluable for any organization."
In summary, as we explore the landscape of data analytics software, it becomes clear that its definition and importance are foundational to selecting the appropriate tools for business needs.
Criteria for Selecting Data Analytics Software
Selecting the right data analytics software is crucial for businesses aiming to leverage data effectively. Quality tools can transform raw data into meaningful insights, drive decision-making, and ultimately enhance overall performance. When evaluating software options, several criteria come into play. These criteria help in narrowing down choices based on individual business needs, user preferences, and specific scenarios.
Key Features to Consider
Data Integration Capabilities
Data Integration Capabilities is one of the most significant factors in choosing analytics software. This feature enables the software to gather data from various sources, making it indispensable for organizations with diverse data streams. The ability to connect seamlessly with databases, APIs, and other software options ensures a holistic view of organizational data.
A key characteristic of strong data integration capabilities is versatility. Software that supports different types of data formats and sources can cater to unique business environments effectively. For example, tools like Tableau offer robust integration options, allowing users to pull data from multiple channels easily. This flexibility provides businesses with the agility to adapt, ensuring they make decisions based on the most comprehensive data sets.
User-Friendly Interface
The User-Friendly Interface is another critical aspect to consider. Even the most powerful analytics software will fall short if users find it challenging to navigate. A simplified interface can significantly improve productivity by allowing users to focus on analysis rather than struggling with the tool itself. An important characteristic here is intuitive design. Software that provides clear menus, tooltips, and a logical flow of information can dramatically reduce the learning curve. This ease of use fosters quicker adoption among team members, especially in organizations with varying levels of technical expertise.
Visualization Tools
Visualization Tools are essential for presenting data insights clearly and engagingly. The ability to convert complex datasets into charts, graphs, and dashboards aids in comprehension and communication of findings to stakeholders. A beneficial characteristic of effective visualization tools is customization. Many software programs, like Microsoft Power BI, offer extensive customization options, allowing users to tailor visuals to their specific needs. This adaptability can make insights more relatable to different audiences but requires a certain level of expertise in design.
Scalability and Flexibility
Scalability and Flexibility refer to how well the software can grow with your business needs. As organizations expand, their data analytics needs evolve. Important considerations include whether the software can handle growing datasets and adapt to new analytics tasks. Choosing scalable software ensures that businesses will not need to switch solutions frequently, which can be both time-consuming and costly. Flexibility allows tools to support various analytics methods, like predictive modeling or real-time data analysis. This adaptability means users can experiment and explore various analytics approaches as their needs change.
Cost Considerations
Cost Considerations play a pivotal role in software selection. Budgets vary across organizations, and understanding the cost vs. value proposition is essential. Hidden costs related to implementation, training, and ongoing support can add up quickly. When evaluating cost, look beyond initial pricing. Factors such as subscription fees, maintenance costs, and scalability-related expenses should also be assessed. Implementing a cost-benefit analysis can help businesses select software that fits their budget while delivering appropriate value.
Top Data Analytics Software in the Market
In today's data-driven world, selecting the best data analytics software is critical for organizations aiming to extract meaningful insights from their data. The right tool can enhance decision-making, drive operational efficiencies, and ultimately improve business outcomes. Understanding various software options allows businesses to align their analytical capabilities with their specific needs. This section delves into the leading data analytics software currently available, analyzing their unique strengths and weaknesses.
Overview of Leading Software Options
Several leading software solutions stand out in the data analytics market. Each offers distinct features and benefits that cater to different organizational needs. When evaluating these options, consider factors such as ease of use, integration capabilities, and overall functionality. Popular platforms include Tableau, Microsoft Power BI, and SAS. These tools lead in adoption rates among businesses, primarily due to their ability to simplify complex data processes.
- Tableau: Known for its advanced visualization capabilities, Tableau provides users with an intuitive interface that allows for rapid dashboard creation.
- Microsoft Power BI: This tool excels in cost-effectiveness and seamless integration with other Microsoft products, making it ideal for many organizations already using the Microsoft ecosystem.
- SAS: Renowned for its robust statistical analysis features, SAS offers powerful analytics capabilities suitable for industries where data handling complexity is high.
Detailed Analysis of Popular Tools
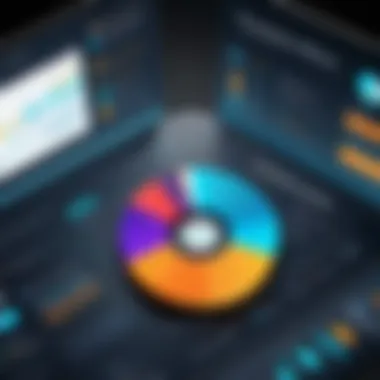
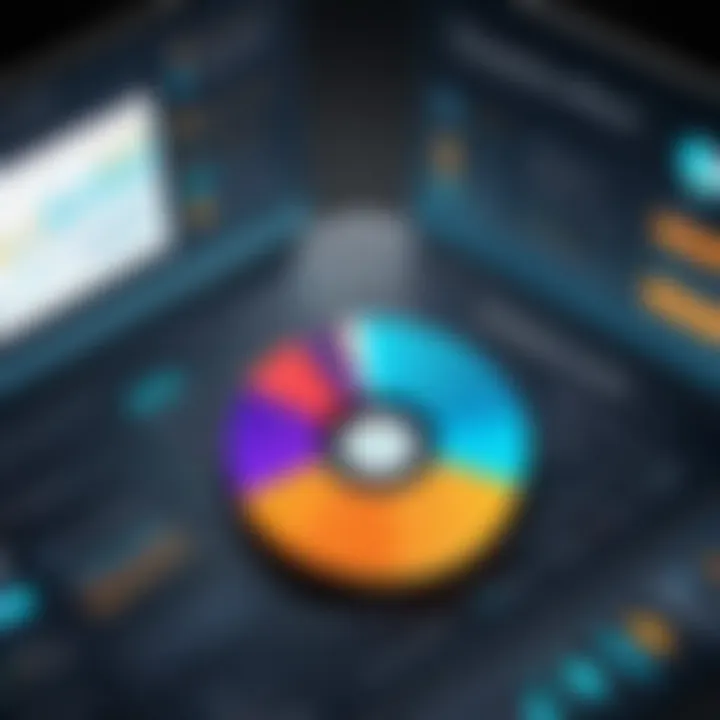
Tool A: Tableau Features and Benefits
Tableau stands out for its dynamic data visualization capabilities. Its primary strength lies in enabling users to create rich, interactive dashboards quickly. Users benefit from a drag-and-drop interface, which requires minimal programming knowledge. The software’s unique feature is the ability to connect to various data sources, including cloud and on-premise options, making it highly versatile.
- Benefits: Tableau allows organizations to visualize large amounts of data effectively.
- Drawbacks: However, it can come at a higher cost, which might be a consideration for smaller organizations.
Tool B: Microsoft Power BI Features and Benefits
Microsoft Power BI is celebrated for its integration with other Microsoft tools. The software is cost-effective and provides powerful data analysis capabilities. Its dashboard features allow users to monitor performance metrics in real time, which is essential for timely decision-making. A unique feature of Power BI is its ability to share insights across teams easily, ensuring consistent data visibility.
- Benefits: The software is very user-friendly and competitive in pricing.
- Drawbacks: Some advanced analytical functions may lack compared to higher-priced alternatives like Tableau.
Tool C: SAS Features and Benefits
SAS is regarded for its comprehensive analytical capabilities. Its predominant characteristic is strength in statistical analysis and predictive modelling. Many organizations in finance and healthcare prefer SAS for its robustness in handling large datasets. The software’s unique feature is its focus on advanced analytics and deep learning, which can provide actionable insights that drive strategic initiatives.
- Benefits: SAS is a powerful tool for organizations where data sophistication is a priority.
- Drawbacks: The complexity of the software might require significant training for new users and can involve a hefty investment.
Final Note: Each of these leading software options has strengths that cater to different types of organizations and use cases. The selection should align with both budget constraints and long-term analytical goals.
Data Visualization in Analytics Software
Data visualization plays a crucial role in the domain of analytics software. This practice allows complex data to be transformed into visually compelling formats that simplify understanding and interpretation. The significance of data visualization is evident as it enhances decision-making processes, provides insight into patterns, and highlights trends. Moreover, effective visualization brings out the story behind the data, which is often lost in raw numbers. Analytics software that incorporates robust visualization tools enables users to make sense of vast amounts of information quickly and effectively, thereby enhancing productivity.
Importance of Visualization in Data Analysis
The importance of visualization in data analysis cannot be overstated. It facilitates the simplification of large datasets into digestible formats. This allows decision-makers to grasp concepts quickly without being overwhelmed by numbers. Furthermore, visualization enhances productivity by aiding in faster comprehension of the intricacies involved in the data.
Effective data visualization not only conveys information but also enables users to derive actionable insights from their analyses.
A strong visual representation can reveal trends and correlations that might not be apparent through text-based data reporting. Analysts and businesses benefit when they can spot anomalies or outliers quickly, driving timely decision-making.
Types of Visualization Tools Available
Several types of visualization tools are common in data analytics software. Each has its specific purpose and advantages, making it important to choose the right one based on the needs of the analysis.
Charts and Graphs
Charts and graphs provide a foundational aspect of data visualization. They translate numbers into graphical representations, making comparisons between variables easy and intuitive. Their key characteristic is clarity; they condense information effectively, which is vital for presentations or reports where time is limited.
The unique feature of charts and graphs is their versatility. Whether it is a pie chart for showcasing composition or a bar graph for comparison, various forms cater to different data types. The advantage of using charts and graphs is they can quickly communicate insights to diverse audiences. However, when the dataset is too complex, they can oversimplify, potentially leading to misinterpretations.
Dashboards
Dashboards have become increasingly popular in data analytics software due to their ability to provide real-time data visualizations in one consolidated view. They display key performance indicators (KPIs) and crucial metrics at a glance, allowing users to monitor data continuously. This tool’s key characteristic is interactivity, giving users the option to drill down into specifics.
The unique feature of dashboards lies in their customizability. Users can tailor the dashboard to their specific requirements, displaying relevant metrics that align with their goals. The advantage of using dashboards is their capacity to consolidate diverse data sources. The downside, however, is that they may become cluttered or overwhelming if too many metrics are presented, which could hinder effective analysis.
Heat Maps
Heat maps provide an advanced visualization method for representing data density and correlations. They use color gradients to indicate which areas are dense with data points. The key characteristic that makes heat maps valuable is their ability to provide a quick visual indication of where significant activity or results are occurring in the dataset.
The unique feature of heat maps is their capacity to illustrate data trends spatially, allowing analysis in contexts such as geographical locations or within applications. The advantage of heat maps is their efficiency in highlighting patterns at a glance. However, they may require a background explanation to comprehend fully, as viewers may misinterpret the color gradients if they do not understand them.
Integration with Other Tools and Platforms
Integration with other tools and platforms is a critical consideration when evaluating data analytics software. Businesses operate within complex ecosystems that include various software systems, databases, and applications. The ability of analytics software to connect seamlessly with these existing tools can significantly influence its utility and effectiveness.
Effective integration allows for smooth data flow between different applications, ensuring that analytics platforms can access the most up-to-date information. This improves decision-making, as analytics insights reflect real-time data rather than outdated information.
APIs and Connectivity
Application Programming Interfaces (APIs) play a vital role in enabling connectivity between different software solutions. APIs act as intermediaries that allow various software systems to communicate with one another. When selecting data analytics software, businesses must assess the robustness of its API capabilities.
Benefits of strong API connectivity include:
- Enhanced Data Access: APIs facilitate the extraction of data from different sources in real time, ensuring that analytics can provide accurate and timely insights.
- Custom Integration Options: With flexible APIs, businesses can build customized connections to suit their unique needs, allowing for tailored data flows that enhance the analytics process.
- Scalability: As businesses grow or change, their technology needs may evolve. APIs allow for adjustments without requiring a full overhaul of existing systems.
Compatibility with Existing Systems
The compatibility of data analytics software with existing systems is another vital consideration. Many organizations have invested heavily in technology stacks over the years. Thus, any new software must work harmoniously with the tools already in place.
To evaluate compatibility, consider the following factors:
- Supported Data Formats: Ensure that the analytics software can handle the data formats used by your organization, such as CSV, JSON, or SQL databases.
- Integration with Legacy Systems: Some businesses still rely on legacy systems. The chosen analytics software should offer tools that can bridge modern technologies with older systems efficiently.
- User Training and Transition: If the analytics software requires extensive modifications to existing processes, a transition plan that includes user training becomes necessary to facilitate smooth adaptation.
Integrating data analytics software with existing tools is not just about connectivity; it is about creating a cohesive data ecosystem that supports strategic decision-making.
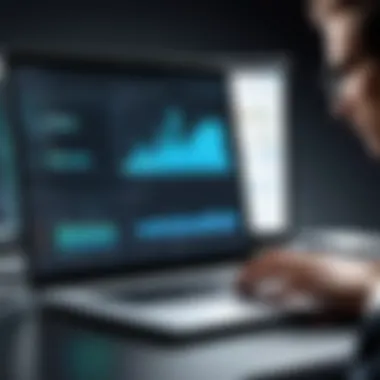
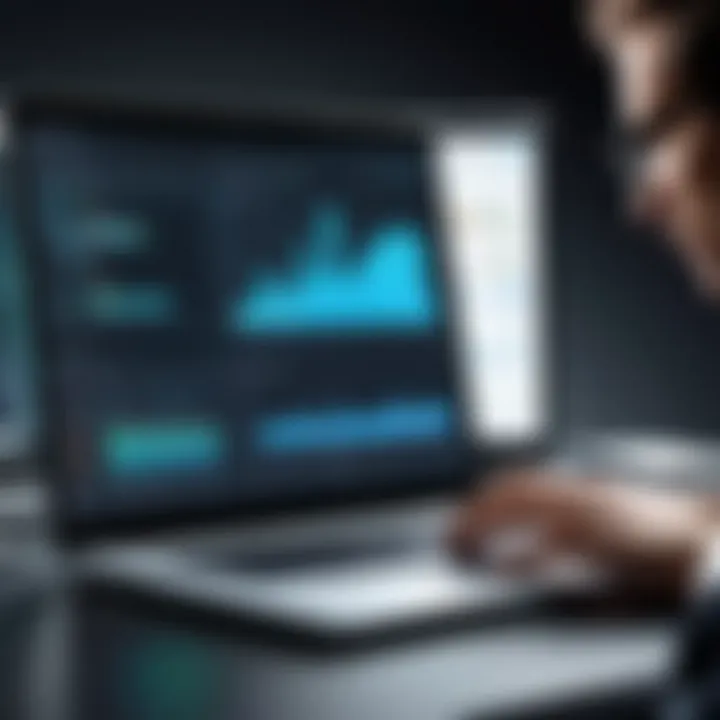
Thoroughly evaluating integration capabilities ensures that businesses do not just adopt advanced analytics software but also derive genuine value from it through effective data utilization.
Challenges in Data Analytics Implementation
Data analytics implementation is crucial for organizations aiming to derive valuable insights from their data. However, navigating the landscape of data analytics comes with its own set of challenges. Addressing these obstacles enables companies to leverage analytics effectively, ensuring a smooth transition and optimal results.
Significance of Identifying Challenges
Recognizing the challenges in data analytics is the first step in formulating an effective strategy. By understanding potential pitfalls, organizations can adopt preventive measures to mitigate risks, leading to successful analytics initiatives. This section delves into critical issues like data quality and user adoption, providing insight into how to overcome these hurdles for better operational efficiency.
Data Quality Issues
Data quality stands as a fundamental aspect of data analytics. High-quality data is essential for producing credible insights. Poor data quality can lead to erroneous conclusions, affecting decision-making processes. Here are some critical factors that underscore the importance of addressing data quality:
- Source Accuracy: Ensuring the accuracy of data sources is vital. Data collected from unreliable sources can result in misleading outcomes. Organizations should prioritize verification processes to establish data credibility.
- Data Completeness: Incomplete data can skew analysis and lead to poor decision-making. Organizations must implement regular audits to identify and fill gaps in data.
- Consistency: Data should remain consistent across various systems and sources. Discrepancies often arise when different departments interpret data differently, complicating the analysis. Standardization is essential to ensure uniformity in data interpretation.
- Timeliness: Market conditions can change rapidly. Therefore, outdated data can hinder responsiveness. Companies should develop strategies to ensure data is regularly updated and relevant.
By addressing data quality issues, organizations can ensure that their analytics efforts yield accurate and actionable insights, ultimately enhancing their competitive advantage.
User Adoption and Training
The implementation of data analytics tools requires not only the right technology but also the adoption by users. If team members do not embrace these tools, the benefits of analytics may remain unrealized.
- Understanding Tool Usage: Employees must fully understand how to utilize analytics tools effectively. This requires comprehensive training programs that can demystify complex features and functionalities.
- Creating a Data-Driven Culture: Fostering a culture that values data can significantly enhance user engagement with analytics tools. When decision-makers promote data-driven approaches, it encourages team members to rely on data insights.
- Continuous Support and Learning: As tools evolve, ongoing education is vital. Organizations should invest in continuous training sessions to keep the workforce updated on new features and best practices.
"Investing in user training ensures that employees feel more confident and competent, directly impacting the effectiveness of data analytics initiatives."
The challenge of user adoption and training is not merely a technical one; it is inherently linked to organizational culture. To navigate this challenge successfully, companies must emphasize communication, transparency, and accessibility in their training methods.
Case Studies of Successful Data Analytics Implementation
Case studies are a powerful tool in understanding the real-world applications of data analytics software. They provide insight into how specific tools can be used effectively within various industries. By analyzing these cases, businesses can identify best practices, learn from successes and failures, and adapt their strategies accordingly.
Using concrete examples helps to illustrate the potential impact of data analytics. These case studies can serve as models for decision-makers, IT professionals, and entrepreneurs who are evaluating the benefits of these tools. They showcase not only the software capabilities but also practical outcomes resulting from implemented strategies.
Industry-Specific Applications
Different industries may leverage data analytics in unique ways. For example, in the healthcare sector, data analytics can improve patient outcomes through predictive modeling and resource optimization. Hospitals can analyze patient data to predict admission rates. This enables better staffing decisions and improves the allocation of medical resources.
In retail, companies like Target utilize data analytics to enhance customer experience. By analyzing purchasing data, Target can tailor marketing strategies, leading to increased customer loyalty and sales. The insights gained from understanding customer behavior are invaluable to stay competitive in a saturated market.
Additionally, in financial services, firms frequently use data analytics for fraud detection. By employing algorithms that analyze transaction patterns, they can quickly identify unusual activities. This not only protects customers but also saves the institutions from significant financial losses.
Lessons Learned from Case Studies
Analyzing outcomes from case studies reveals important lessons for business leaders. For instance:
- Implementation is Critical: Even the best data analytics tools can fail if they are not implemented properly. Organizations must ensure staff are trained and that workflows are adapted to integrate new technologies.
- Realistic Expectations: Setting measurable goals is essential. Many organizations experience initial setbacks before achieving success. Understanding this process can help in managing expectations.
- Continuous Improvement: Data analytics is not a one-time set up. Industries learn from ongoing analysis, and firms need to adapt as technology and market demands evolve.
"Businesses must be prepared to iterate on their analytics strategies, not just set them in stone."
Future Trends in Data Analytics Software
The landscape of data analytics software is constantly evolving. Understanding future trends can aid businesses in making informed decisions. Staying ahead in technology can provide a competitive edge. The integration of emerging technologies and the evolution of market dynamics play a crucial role in this landscape.
Emerging Technologies in Analytics
Emerging technologies are reshaping data analytics. Innovations such as artificial intelligence (AI) and machine learning are at the forefront. They enable faster data processing and deeper insights. Here are several key technologies to watch:
- AI-Driven Analytics: Tools that incorporate AI can automate data analysis. This reduces time spent on manual data processing.
- Natural Language Processing (NLP): NLP allows users to interact with data through simple language queries. This makes analytics accessible to non-technical users.
- Real-Time Data Processing: Technologies that allow for real-time analytics are increasingly important. Businesses can make quicker and more informed decisions based on current data.
- Cloud Computing: The rise of cloud platforms enables scalable analytics solutions. Companies can store and access large datasets efficiently.
Emerging technologies like AI and machine learning are not just trends; they are critical tools for business growth.
These advancements bring both opportunities and challenges. Companies must ensure proper integration with their existing systems. Training staff to utilize these new tools effectively is essential for success.
Predictions for the Market
The future of data analytics software is influenced by several predicted trends. These are relevant for decision-makers and IT professionals as they plan for the future.
- Increased Adoption of Self-Service Analytics: More businesses are shifting towards self-service platforms. These tools empower users to generate their insights without relying heavily on IT.
- Focus on Data Governance and Compliance: As data regulations become stricter, businesses will invest more in compliance. Software solutions that offer built-in data governance will see greater demand.
- Personalization of User Experiences: The demand for customized analytics experiences is growing. Software that tailors dashboards and reports to individual user needs will stand out.
- Expansion of Predictive Analytics: Companies will increasingly depend on predictive models to anticipate future trends. The ability to forecast will drive strategic planning and operational efficiency.
In summary, the future of data analytics software holds significant potential. Companies embracing these trends can enhance their analytics capabilities and achieve better outcomes in their business strategies.
Epilogue
In the fast-paced world of data analytics, understanding the nuances of selecting the right software is crucial. This article has explored the key components that businesses must consider when integrating data analytics tools into their operations. Having clear criteria helps organizations to make informed decisions tailored to their specific needs. Each software option comes with its own set of features, advantages, and potential drawbacks. The goal should always be to align these tools with business objectives.
Key Takeaways
- Understanding Needs: Before choosing a data analytics software, evaluate the specific needs of your team. Identify the types of analysis you frequently conduct and the insights you hope to gain.
- Scalability Matters: As businesses grow, their data needs may evolve. Choose software that can adapt and scale without requiring a complete overhaul in the future.
- Integration is Key: Look for tools with robust integration capabilities. This ensures that your analytics software can work seamlessly with existing systems and data sources.
- Cost Considerations: Budget constraints are a reality for most organizations. Find software solutions that provide the best value without compromising essential features.
"Selecting the right data analytics software can transform how organizations interpret their data and make strategic decisions."
Final Thoughts on Selection Strategy
Developing a strategy for selection goes beyond merely browsing available options. It involves a thorough understanding of what each software can deliver and how it aligns with your organization’s goals. Establish a collaborative decision-making process that includes stakeholders from different departments. This ensures all angles are considered.
Regularly revisit your analytics tools and strategies as technology evolves. What may suffice today could lack the efficiency or capability tomorrow. Ultimately, the objective is to harness data effectively, ensuring your organization remains competitive and insightful in its decision-making.