A Comprehensive List of Data Mining Software: Exploring Various Options
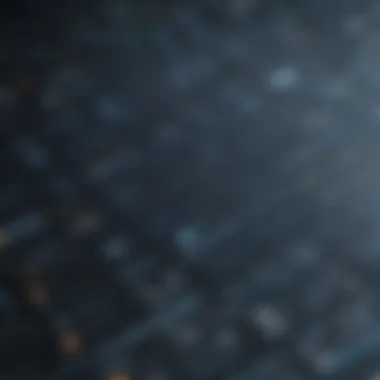
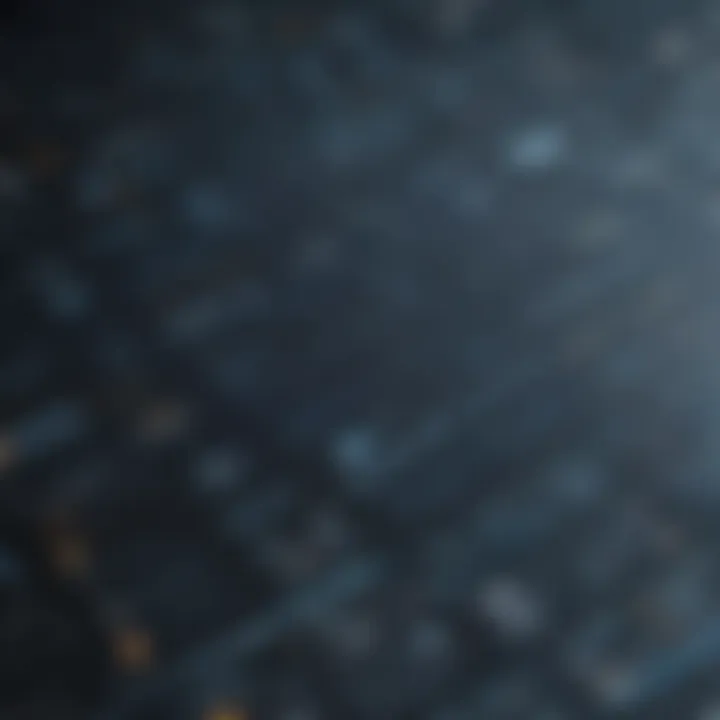
Industry Overview
Data mining software plays a pivotal role in today's information-driven world, offering powerful tools to extract valuable insights from vast datasets. As businesses strive to leverage data for strategic decision-making, the demand for innovative data mining software continues to rise. Emerging technologies like artificial intelligence and machine learning are reshaping the landscape, propelling the industry forward with advanced analytical capabilities.
Top Software Options
In the realm of data mining software, several leading providers stand out for their comprehensive features and robust performance. From established platforms like IBM Watson and SAS to newer entrants such as RapidMiner and KNIME, each software option brings unique strengths to the table. A detailed review and comparison of these top solutions will shed light on the key features, functionalities, and pricing structures, empowering users to make informed choices based on their specific requirements.
Selection Criteria
When navigating the multitude of data mining software options available, it is crucial to consider several factors to ensure the chosen solution aligns with your business needs. From scalability and predictive analytics capabilities to user-friendliness and cost-effectiveness, evaluating these critical factors can streamline the selection process. By understanding common mistakes to avoid and learning how to determine the right fit for your organization, you can make a well-informed decision that drives success.
Implementation Strategies
Implementing data mining software effectively is as vital as selecting the right solution. By embracing best practices for seamless implementation, organizations can maximize the benefits of their chosen software. This includes strategies for integration with existing systems and processes, as well as investing in comprehensive training and support resources. Ensuring a smooth deployment process is essential for optimizing the functionality and usability of the software within your business environment.
Future Trends
Looking ahead, the future of data mining software promises exciting advancements and innovations that will shape the industry landscape. From cutting-edge technologies like deep learning and natural language processing to emerging trends in data visualization and cloud computing, staying abreast of these developments is key to future-proofing your technology stack. By anticipating future trends and proactively adapting to industry shifts, businesses can position themselves for sustained growth and competitiveness in a rapidly evolving digital era.
Introduction
Data mining software plays a crucial role in today's data-driven world. It serves as a powerful tool for extracting valuable insights from vast amounts of data, aiding in decision-making processes across various industries. Understanding the nuances of different data mining software is essential for businesses aiming to optimize their analytical capabilities and stay ahead in a competitive market landscape. This article delves deep into the realm of data mining software, offering a comprehensive overview of both established platforms and emerging tools, providing readers with a nuanced understanding of the key features and functionalities of each software.
Understanding Data Mining Software
Definition of Data Mining Software
Data mining software refers to a set of tools and techniques used to extract patterns and knowledge from large datasets. Its primary function is to uncover hidden trends, relationships, and anomalies within data to facilitate informed decision-making. The essence of data mining software lies in its ability to process and interpret complex datasets, enabling businesses to derive actionable insights from seemingly disparate information sources. The unique characteristic of data mining software lies in its advanced algorithms and data processing capabilities, making it a valuable asset for organizations seeking to enhance their analytical capacities.
Importance in Data Analysis
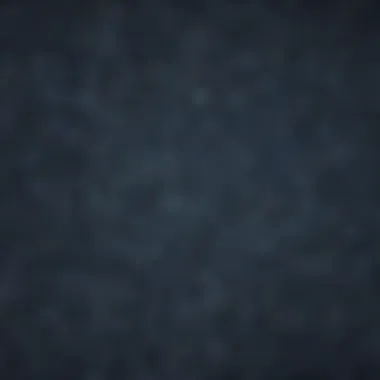
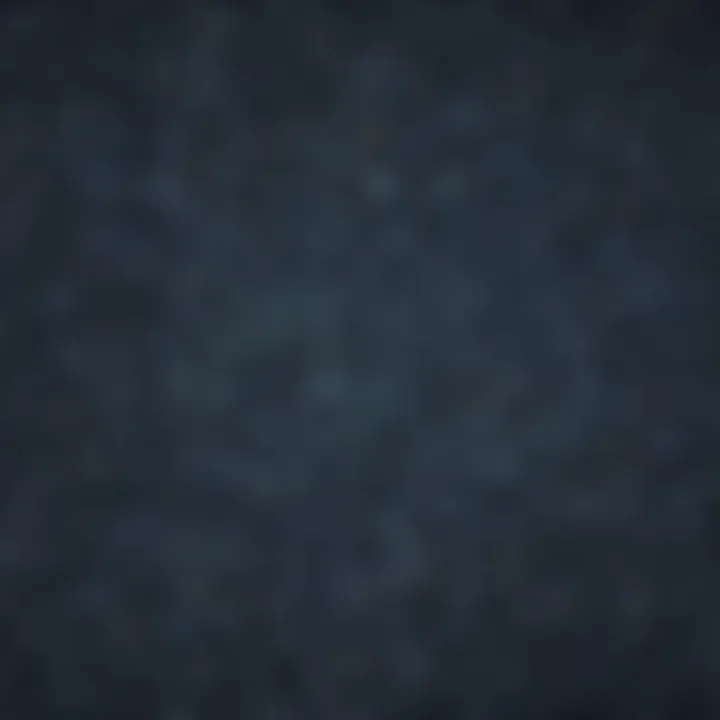
The significance of data mining software in data analysis cannot be overstated. By employing sophisticated algorithms and statistical models, data mining software allows analysts to discover valuable patterns and relationships within data, thus empowering them to make informed decisions. The key benefit of data mining software lies in its ability to transform raw data into actionable intelligence, driving strategic initiatives and forecasting future trends. While data mining software offers unparalleled opportunities for data exploration and analysis, its complexity may pose challenges for users unfamiliar with advanced statistical methods. As such, a deep understanding of data mining software is vital for maximizing its potential in the realm of data analysis.
Scope of the Article
Objective of Compiling Software List
The main objective of compiling this software list is to equip readers with a comprehensive overview of the diverse data mining tools available in the market. By curating a range of established platforms and emerging tools, this list aims to elucidate the distinct features and functionalities of each software, enabling readers to make informed decisions based on their specific requirements. The software list serves as a valuable resource for organizations seeking to implement robust data mining solutions tailored to their unique analytical needs.
Target Audience
This article caters to a broad spectrum of professionals, including decision-makers, IT professionals, and entrepreneurs, who are vested in the realm of data analysis and business intelligence. The content is tailored to address the needs of individuals seeking to enhance their understanding of data mining software and its implications for data-driven decision-making. Whether you are a seasoned data analyst or a budding entrepreneur looking to leverage data insights for strategic growth, this article offers valuable insights into the world of data mining software.
Popular Data Mining Software
Data mining software plays a pivotal role in the realm of data analysis, facilitating the extraction of valuable insights from vast datasets. In this article, the focus is on delving deep into the diverse landscape of popular data mining software options available in the market. From well-established platforms to up-and-coming tools, this section aims to provide a nuanced understanding of the features and functionalities that define each software. By showcasing the specific elements, benefits, and considerations of popular data mining software, readers can gain a comprehensive overview to aid in their decision-making process.
Established Platforms
Microsoft SQL Server Analysis Services
Microsoft SQL Server Analysis Services stands out as a stalwart in the realm of data mining software. Its robust capabilities in handling complex data analysis tasks make it a favored choice for organizations aiming to derive actionable insights from their datasets. The key characteristic of Microsoft SQL Server Analysis Services lies in its parallel processing capabilities, which enhance scalability and performance significantly. However, one notable detriment is its relatively steep learning curve for novice users. Despite this, its integration capabilities and data integration support make it a valuable asset in the data mining software landscape.
IBM SPSS Modeler
IBM SPSS Modeler is another heavyweight in the data mining software arena, distinguished by its user-friendly interface and extensive array of machine learning algorithms. The key characteristic of IBM SPSS Modeler is its comprehensive suite of classification and clustering algorithms, empowering users to uncover patterns and trends within their data effortlessly. One of its standout features is the seamless integration with other IBM software products, streamlining data analysis workflows. While IBM SPSS Modeler excels in predictive analytics, its pricing structure can be a deterrent for smaller enterprises seeking cost-effective solutions.
Oracle Data Mining
Oracle Data Mining emerges as a formidable contender among established data mining platforms, known for its prowess in handling large datasets with efficiency. The key characteristic of Oracle Data Mining lies in its robust regression and association rule mining algorithms, enabling users to extract valuable insights for making informed decisions. Its seamless integration with Oracle's database management system fosters a cohesive data analysis environment. However, the dependency on Oracle's ecosystem and licensing costs may pose challenges for organizations with budget constraints.
Emerging Tools
RapidMiner
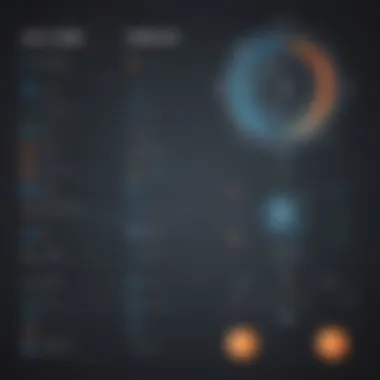
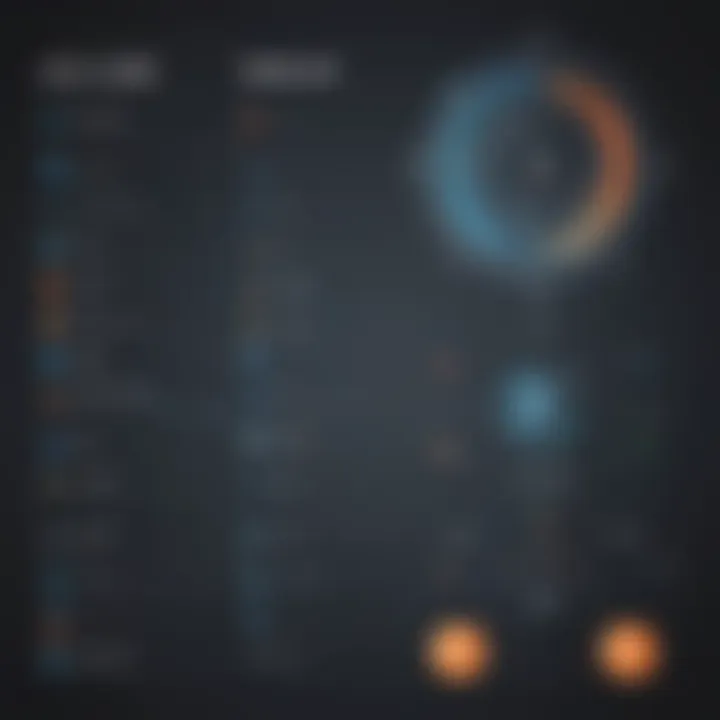
RapidMiner stands out as a versatile and intuitive data mining tool, catering to the needs of both novice and advanced users. The key characteristic of RapidMiner is its user-friendly interface and drag-and-drop functionality, simplifying the process of building machine learning models. Its unique feature lies in the extensive library of pre-built templates and workflows, streamlining the data analysis process. While RapidMiner excels in data preparation and modeling, its pricing structure based on usage may not align with the budgetary constraints of all organizations.
Weka
Weka, a popular open-source data mining tool, garners attention for its extensive collection of machine learning algorithms and data preprocessing tools. The key characteristic of Weka is its flexibility and adaptability, allowing users to experiment with diverse algorithms and techniques. Its unique feature lies in the visualization tools that aid in interpreting the results of data mining algorithms effectively. Despite its strengths in algorithmic diversity, Weka's reliance on Java programming may pose challenges for users accustomed to other programming languages.
KNIME
KNIME emerges as a robust and scalable data analytics platform, offering a wide range of functionalities for data preprocessing and analysis. The key characteristic of KNIME is its integration capabilities with various data sources and tools, promoting a unified approach to data processing. Its unique feature lies in the availability of ready-to-use components and extensions, enhancing the extensibility of the platform. While KNIME excels in workflow automation and collaboration, its advanced features may require a learning curve for users transitioning from simpler data mining tools.
Key Features Comparison
In the realm of data mining software exploration, the comparison of key features holds paramount importance. By analyzing scalability and performance, decision-makers, IT professionals, and entrepreneurs can discern the most suitable tools for their needs. Scalability refers to the software's ability to handle large volumes of data efficiently, crucial in modern data-driven environments. Performance, on the other hand, evaluates how effectively the software processes and analyzes data, impacting the speed and accuracy of results. Assessing parallel processing capabilities is essential as it allows for simultaneous data processing, optimizing performance and reducing processing times significantly. Data integration support plays a pivotal role in ensuring seamless compatibility with various data sources, enhancing the software's adaptability and utility.
Scalability and Performance
Parallel Processing Capabilities
When delving into parallel processing capabilities, we unveil a core aspect that drives efficiency and effectiveness in data mining software. The ability to process multiple tasks simultaneously enhances the software's performance and speed of data analysis. This feature is particularly beneficial for handling vast datasets, enabling quick insights extraction and streamlined workflows. The unique advantage of parallel processing lies in its ability to divide tasks into smaller sub-tasks that can be executed concurrently, harnessing the full computing power of the system. While advantageous in enhancing speed and efficiency, complexities in managing parallel processes may arise, necessitating robust system resources for optimal functioning.
Data Integration Support
Within the realm of data integration support, the software's capability to seamlessly incorporate diverse data sources emerges as a catalyst for comprehensive analysis. Ensuring harmonious data interaction across different platforms and formats is crucial for generating holistic insights. The key characteristic of data integration support lies in its ability to unify disparate data sets, creating a unified ecosystem for analysis. This feature is a popular choice for organizations seeking to maximize the value of their data assets by amalgamating information from various sources. While advantageous in enabling comprehensive analyses, intricate data mapping and transformation processes may pose challenges, requiring meticulous attention to detail.
Machine Learning Algorithms
In the landscape of data mining software, machine learning algorithms play a pivotal role in driving analytical capabilities. The utilization of classification and clustering algorithms empowers users to categorize and group data points effectively, enabling pattern recognition and data segregation. These algorithms offer a reliable method for organizing data into meaningful clusters and classes, facilitating advanced analysis and prediction. The unique feature of classification and clustering algorithms lies in their unsupervised learning approach, allowing the software to identify patterns autonomously. Regression and association rule mining further enhance analytical capabilities by enabling predictive modeling and identifying significant relationships within the data set. By leveraging regression and association rule mining, users can uncover valuable insights and correlations, enhancing decision-making processes.
Classification and Clustering Algorithms
Addressing the intricacies of classification and clustering algorithms reveals their significant contribution to data mining software functionality. These algorithms excel in discerning patterns within data sets, facilitating the organization and segmentation of information based on similarities and relationships. The key characteristic of classification and clustering algorithms is their ability to categorize data points into distinct groups, aiding in data interpretation and exploration. This feature is a popular choice for organizations seeking to identify trends and behaviors within large datasets efficiently. While advantageous in organizational structuring, algorithmic biases and data noise might affect the accuracy of results, necessitating thorough validation procedures.
Regression and Association Rule Mining
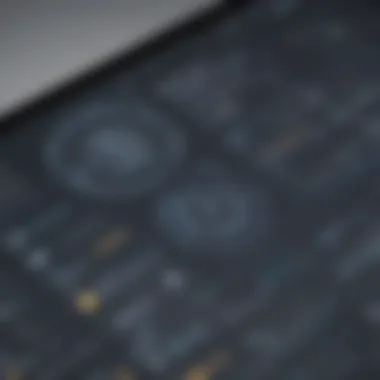
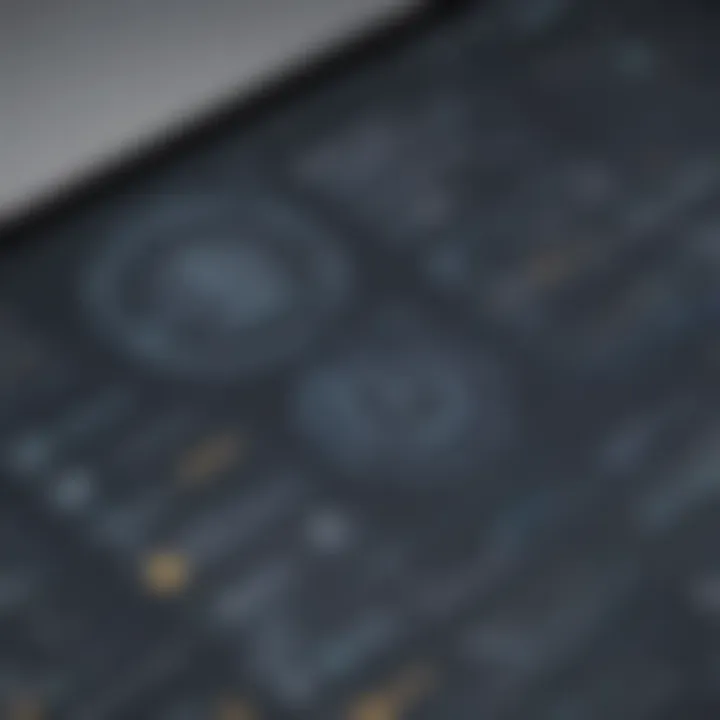
Within the realm of regression and association rule mining, the software's capacity for predictive modeling and rule discovery shines brightly. Regression algorithms enable users to predict future trends and outcomes based on historical data patterns, offering valuable insights for decision-making processes. Association rule mining, on the other hand, uncovers significant relationships and dependencies within the data, unveiling hidden patterns and associations. The unique feature of regression and association rule mining lies in their ability to extract meaningful information from complex data sets, aiding in strategic decision-making. While advantageous in predictive analytics, the complexity of algorithm selection and interpreting results accurately may pose challenges, requiring domain expertise for optimal utilization.
Factors to Consider When Choosing Data Mining Software
In the realm of data mining software selection, the consideration of various factors plays a pivotal role in determining the effectiveness and suitability of a solution. Acknowledging the importance of this aspect within the scope of this article allows us to delve deeper into the intricacies of decision-making processes associated with software selection. Factors like cost, licensing agreements, integration capabilities, and other significant aspects contribute significantly to the overall success of a data mining endeavor, making them vital components to explore and understand thoroughly.
Cost and Licensing
Open Source vs. Commercial Solutions
Delving into the debate between open-source and commercial solutions in the data mining software domain uncovers a myriad of considerations that can influence decision-making processes. While open-source solutions offer cost-efficiency and flexibility, commercial options often come with robust support and advanced features tailored to specific industry requirements. Deciphering the unique features and advantages of each model becomes crucial in aligning software choices with organizational goals and budget constraints. Understanding the nuances between open source and commercial solutions equips decision-makers with the knowledge needed to make informed and strategic choices that align with their data mining objectives.
Subscription Models
The evolution of subscription-based models in the data mining software landscape has introduced a new dimension to software acquisition and utilization. Subscriptions offer a flexible and scalable approach to accessing cutting-edge software functionalities without hefty upfront investments. By exploring the distinctive characteristics and implications of subscription models, organizations can leverage this financial model to optimize software utilization, streamline budget allocation, and ensure long-term compatibility with evolving business needs. Subscriptions present a strategic advantage for decision-makers aiming to balance cost efficiency with technological innovation in their data mining software selection process.
Integration Capabilities
Compatibility with Existing Systems
Assessing the compatibility of data mining software with existing systems unveils crucial insights into the seamless integration potential and operational efficiency gains that organizations can achieve. Ensuring that the selected software aligns with current infrastructure, data formats, and operational protocols becomes essential for minimizing disruptions and optimizing workflow continuity. The ability of a software solution to integrate effortlessly with existing systems empowers organizations to harness the full potential of their data resources while enhancing productivity and performance levels across operational domains.
API Support
The presence of robust Application Programming Interface (API) support in data mining software signifies advanced data interaction capabilities and interoperability with external applications or systems. API support enables seamless data exchange, automation of processes, and enhanced connectivity, fostering a cohesive ecosystem of data-driven functionalities. Evaluating the unique features and advantages of API support in software selections enables organizations to future-proof their data mining endeavors and adapt to evolving technological landscapes with agility and efficiency.
Conclusion
In the realm of data mining software, the conclusion serves as the crucial culmination of all discussions and analyses. This section encapsulates the essence of the article, providing a comprehensive recap of the key insights unearthed in the exploration of various software options. By distilling a wealth of information, the conclusion aims to guide readers towards making well-informed decisions tailored to their unique requirements and preferences. It acts as a compass, steering individuals through the maze of data mining software, towards a clear understanding of the landscape and the considerations that matter most in the selection process.
Final Thoughts on Data Mining Software
Importance in Decision-Making
Importance in decision-making within the context of data mining software underscores a fundamental aspect that influences business strategies and analytical outcomes. In this article, the significance of utilizing data mining software as a linchpin in the decision-making process is underscored. This aspect not only streamlines the extraction of actionable insights but also enhances the precision and relevance of decisions made based on data-driven intelligence. The pivotal characteristic of this importance lies in its ability to transform raw data into valuable, strategic information that drives organizational success and competitiveness in the modern landscape. Businesses that harness the power of data mining software for decision-making typically experience improved operational efficiency, enhanced predictive analytics capabilities, and a more agile response to market dynamics. While the advantages of leveraging data mining software for decision-making are abundant, it is essential to note potential considerations such as data security, model accuracy, and the interpretability of results to wield this tool effectively in the decision-making realm.
Continuous Evolution in the Field
The notion of continuous evolution in the field of data mining software embodies a dynamic trajectory characterized by ongoing advancements, innovations, and adaptability. Within the scope of this article, the perpetual evolution of data mining software underscores its responsiveness to emerging technological trends, evolving business needs, and expanding analytics requirements. This dynamic nature ensures that data mining software remains at the forefront of transformative technologies, integrating novel algorithms, processing capabilities, and integrations to meet the evolving demands of modern enterprises. The key characteristic of this continuous evolution lies in its ability to future-proof organizations, enabling them to stay ahead of the curve and capitalize on cutting-edge analytics capabilities. By embracing continuous innovation, businesses can harness the latest tools, techniques, and methods offered by data mining software, paving the way for sustained growth, competitive advantage, and data-driven excellence. Despite its numerous advantages, the evolving nature of data mining software necessitates a proactive approach to adaptation, regular upskilling of personnel, and a strategic roadmap for integrating new features seamlessly into existing workflows.