Exploring Erwin Modeling for Effective Data Management
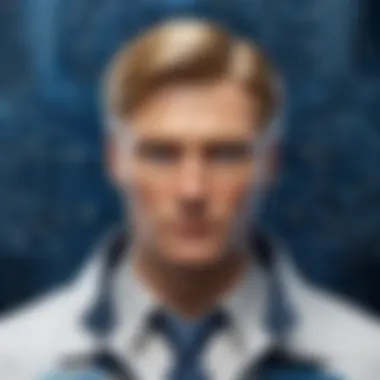
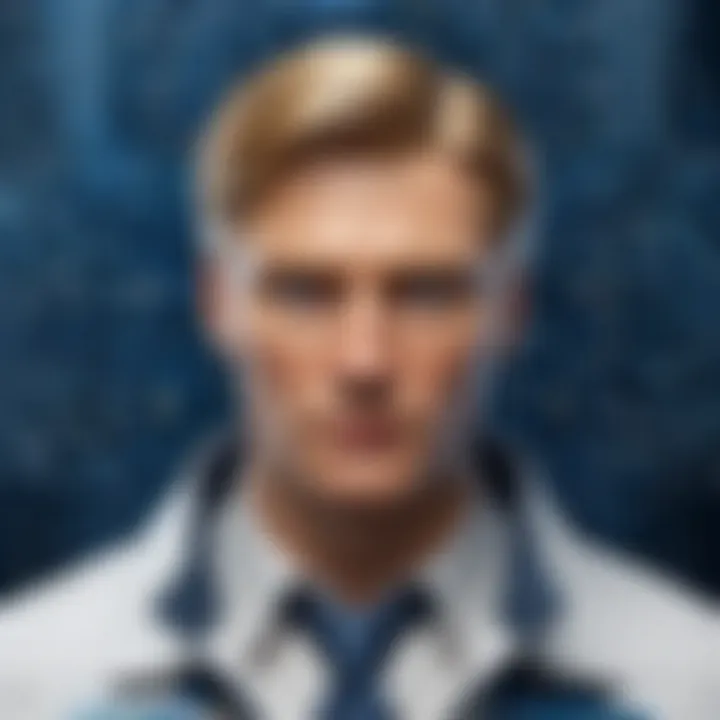
Intro
In an era where data reigns supreme, the methodologies we employ to manage and understand that data have never been more critical. The intricacies of data modeling present a backbone to effective data governance, and among the myriad tools available, Erwin Modeling stands out as a prominent option that epitomizes regulation and structure. This article takes you on a journey through the depths of Erwin Modeling, examining its significance, features, and real-world applications tailored for professionals across various sectors. Whether you are a seasoned IT professional, decision-maker, or entrepreneur, this guide aims to enhance your comprehension of data management through Erwin tools.
Industry Overview
The ever-evolving software market is shaped by various forces, including technological advancements, changing consumer needs, and regulatory developments. Organizations now face higher demands for data management solutions that can handle significant volumes of data while ensuring accuracy and reliability.
Current Trends in the Industry-specific Software Market
Recent trends show a marked increase in the appetite for comprehensive data solutions that not only address the immediate needs for data governance but also align with broader business strategies.
- Cloud Adoption: Organizations are migrating to cloud-based solutions, facilitating flexibility and scalability.
- Data Democratization: There's a push toward making data stewardship a collective responsibility within organizations, thereby enabling more employees to work with data effectively.
- Real-Time Analytics: Businesses require real-time insights, necessitating tools that can provide immediate access to relevant data.
Key Challenges Faced by Buyers in the Industry
Despite the advancements in technology, buyers encounter several hurdles when selecting appropriate software. These include:
- Complex Integration: Aligning new tools with existing systems often presents technical challenges.
- Cost Management: Budget constraints can complicate the selection process, especially when evaluating premium solutions.
- Talent Scarcity: Finding capable staff to manage and utilize data tools remains a significant barrier for many organizations.
Emerging Technologies Impacting the Industry
As we look forward, emerging technologies are beginning to leave their mark on data management practices. Innovations such as Artificial Intelligence (AI) and Machine Learning (ML) are reshaping how data is analyzed and utilized. Furthermore, tools integrating these technologies enable predictive analytics, establishing a more robust framework for informed decision-making.
Top Software Options
When delving into software options that enhance data management, especially with a focus on tools like Erwin, it is essential to understand the competitive landscape.
Review of Leading Software Providers in the Industry
Some of the major players in the data management domain include:
- Erwin: Renowned for its data modeling capabilities and governance features.
- IBM InfoSphere: Offers comprehensive data integration and management solutions.
- Oracle Data Modeler: Provides a robust environment for creating logical and physical data designs.
Feature Comparison of Top Software Solutions
Choosing a tool isn't just about brand recognition. Key features to consider include:
- Data modeling capabilities
- Collaborative functionalities
- Integration capabilities with other systems
- Reporting and analytics features
Here’s a quick glance:
| Feature | Erwin | IBM InfoSphere | Oracle Data Modeler | | Data Visualization | Yes | Yes | Yes | | Collaboration Tools | Yes | Limited | No | | Cost-effectiveness | Moderate | High | Moderate |
Pricing Structures of Different Software Options
When considering the financial aspect, potential buyers should weigh their options based on:
- Licensing Fees: One-time fees or subscription models vary greatly.
- Additional Costs: Training and support can add up quickly.
For example, while Erwin follows a subscription-based model with tiers based on user count, Oracle often leans towards one-time licensing which can be substantial.
Selection Criteria
Choosing the right software is a meticulous process, laden with critical considerations.
Important Factors to Consider When Choosing Software
- Scalability: Can it grow with your organization’s needs?
- User-Friendliness: Is it intuitive enough for your team?
- Support Services: How reliable is the vendor's support?
Common Mistakes to Avoid During the Selection Process
- Not involving end users in the selection process can cause discontent.
- Underestimating training requirements often leads to poor adoption.
- Focusing solely on cost instead of value.
How to Determine the Right Fit for Your Business Needs
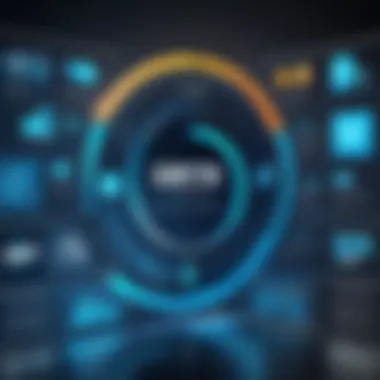
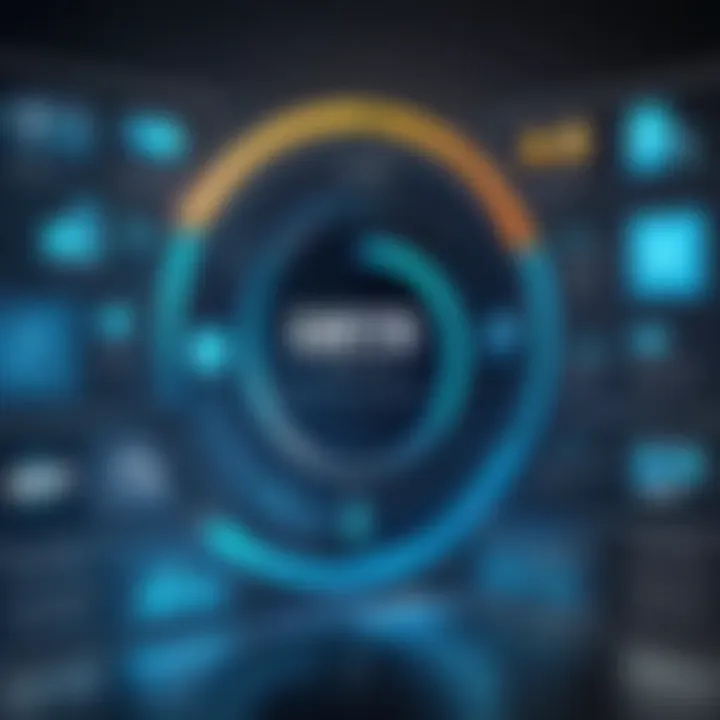
Consider your organization’s specific requirements, culture, and existing infrastructure. This alignment plays a pivotal role in ensuring that the selected tool doesn't just serve immediate needs, but also complements long-term goals.
Implementation Strategies
Once the right software is chosen, the next step revolves around effective implementation.
Best Practices for Smooth Implementation of Software Solutions
- Form a dedicated team for project management.
- Set clear objectives and timelines.
- Regularly communicate with stakeholders.
Integration with Existing Systems and Processes
Integration can be a daunting task. Ensure compatibility with current systems while optimizing workflows to minimize disruption.
Training and Support Resources for Successful Deployment
Investing time in training sessions helps users acclimate to new tools. Utilizing vendor resources or investing in a training consultant can enhance this transition phase.
Future Trends
Looking ahead, the industry-specific software landscape is poised for transformation.
Predictions for the Future of the Industry-specific Software Landscape
As businesses evolve, we can anticipate an increase in AI-driven data solutions that enhance operational efficiency and decision-making processes.
Upcoming Technologies Shaping the Industry
Blockchain technology may also have implications for data governance, offering transparency and enhanced security.
Recommendations for Future-Proofing Your Technology Stack
Staying adaptable by monitoring industry changes and regularly updating software can ensure longevity and relevance in your technology stack. Furthermore, fostering a culture of continuous learning among employees will allow organizations to leverage advancements effectively.
In summary, having an understanding of Erwin Modeling and data management’s holistic approach is indispensable for professionals aiming to harness the full potential of their data.
Preamble to Data Modeling
Data modeling stands at the core of any effective data management strategy. It serves as a blueprint for organizations, helping them to visualize and organize their data in a manner that is both efficient and meaningful. This process not only streamlines operations but also enhances data quality, improves accessibility, and ultimately drives better decision-making across departments.
Definition and Importance of Data Modeling
Data modeling can be described as the practice of creating a data model to visually represent the information and relationships within various systems. A well-structured model can encapsulate how different data entities interact, which makes it easier for teams to understand their data landscape. Here are some key points that highlight its importance:
- Clarity: Data models provide a clear definition of data elements which helps the teams to understand the components of the system.
- Efficiency: By establishing a logical framework, data modeling allows for efficient data retrieval and management.
- Error Reduction: A well-conceived data model minimizes the risk of redundancy and inconsistency, leading to more accurate data overall.
- Foundation for Development: Models lay the groundwork for database design and system development, essential for project success.
In essence, data modeling is not just a technical task but a fundamental practice that backs business intelligence initiatives. With a keen understanding of data schemas and relationships, organizations can derive actionable insights that drive growth and innovation.
Challenges in Data Management
Despite its significance, data management comes with its own set of challenges. Here are some of the hurdles organizations often face:
- Data Silos: Information often resides in isolated pockets, making it difficult to gain a holistic view of the data landscape.
- Quality Issues: Inaccurate or outdated data can lead to poor decision-making, frustrating various stakeholders who rely on consistent data quality.
- Complex Systems: The increasing complexity of IT systems poses challenges for integration and management, creating barriers to efficient data flow.
- Compliance: With ever-evolving regulations on data usage and privacy, organizations must stay vigilant in maintaining compliance while managing their data.
*"Data management isn't just about technology; it's about fostering a culture of data-driven decision-making.
Overview of Erwin Modeling
Understanding Erwin Modeling is crucial for anyone looking to grasp the intricacies of data management in today’s fast-paced digital landscape. The essence of this tool lies in its ability to streamline data architecture, enhancing both governance and usability across various sectors. With organizations dealing with an ever-growing amount of data, having a structured approach to data modeling becomes more than just an option—it’s a necessity.
History and Evolution of Erwin Software
The journey of Erwin Software dates back to the late 1980s, when it emerged as a pioneering solution aimed at addressing data management challenges. Initially designed for relational database modeling, it quickly gained traction among database professionals. Over the years, Erwin has evolved tremendously, adapting to the changing technological landscape.
From its early days, Erwin began to incorporate features that addressed the demands of both technical users and business stakeholders. By the early '90s, data analysts recognized Erwin Modeler as a key tool for enabling data governance and compliance. This evolution continued with regular updates, introducing functionalities like data lineage tracing and metadata management.
The release of Erwin Data Modeler in the 2000s marked a pivotal shift. It provided extensive support for data visualization, which made navigating complex models more intuitive. Today, Erwin remains not only relevant but a leading choice for organizations looking to enhance their information architecture.
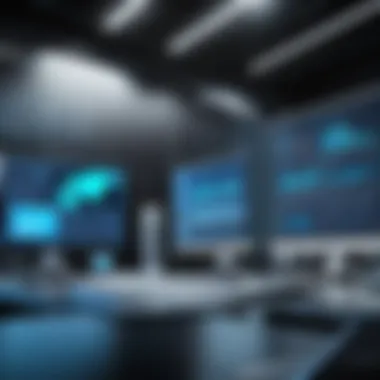
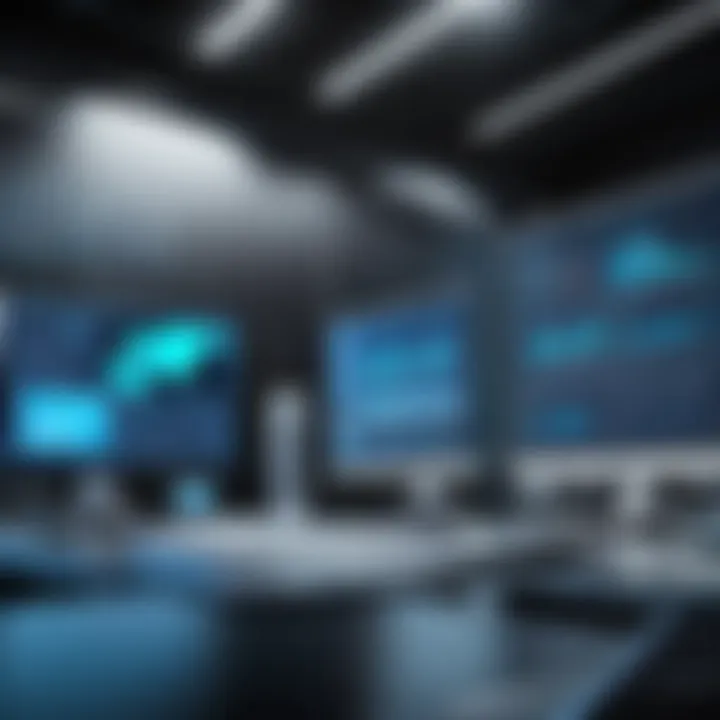
Core Features of Erwin Modeling Tools
Erwin Modeling tools are packed with features that cater to the needs of diverse users. The standout offerings include:
- Graphical Interface: The user-friendly interface allows for easy creation and visualization of data models, making it accessible for both novice and experienced users.
- Collaboration Tools: Real-time collaboration features ensure that teams can work together seamlessly, regardless of their physical location.
- Advanced Metadata Management: This enhances the ability to track data lineage and understand the data lifecycle, facilitating better governance.
- Support for Multiple Models: Whether it's conceptual, logical, or physical data models, Erwin provides the capability to handle them all, ensuring flexibility based on project needs.
These features not only save time but also enhance accuracy in data management, making Erwin a vital asset for businesses.
Integration with Other Technologies
The versatility of Erwin does not stop at its core features. Its ability to integrate with various technologies adds another layer of functionality that enhances its appeal. Whether it's integrating with cloud platforms, ERP systems, or data warehousing solutions, Erwin ensures that organizations can maintain a cohesive data management strategy.
Some notable integrations include:
- Cloud Services: With the growing preference for cloud-based solutions, Erwin's ability to blend with platforms like AWS and Microsoft Azure simplifies migration and management processes.
- Business Intelligence Tools: Integrating with tools such as Tableau and Power BI further leverages Erwin's capabilities, allowing for richer data insights.
- Data Governance solutions: Compatibility with tools designed for governance, security, and compliance forms a crucial part of comprehensive data management strategies.
"The strength of Erwin lies in its flexibility and adaptability, allowing users to create solutions tailored specifically to their organization’s unique data landscape."
This integrative approach makes Erwin a preferred choice for organizations across various industries, unlocking the potential to establish a robust and agile data architecture.
Types of Data Models Supported by Erwin
In the realm of data management, data models serve as the backbone of the architecture, shaping how information is structured and utilized. Erwin Modeling excels in this area by providing diverse types of data models that cater to various needs within organizations. Understanding these models is crucial, as they allow professionals to visualize data needs, establish relationships, and implement strategy effectively.
Conceptual Data Models
Conceptual Data Models are like the blueprints of data architecture. They focus on high-level entities and the relationships between them without delving into technicalities. This type of model is essential for understanding the overall structure of the data landscape, making it accessible to stakeholders who may not be data experts.
It allows organizations to map out the critical components that affect business operations such as customers, products, and transactions.
By using a Conceptual Data Model, teams can identify:
- Key entities that matter to the business.
- Important relationships that affect data flow.
- Business rules governing the data elements.
This model serves as a communication tool among stakeholders, facilitating discussions around data requirements and strategies.
Logical Data Models
Once the conceptual framework is set, Logical Data Models come into play. These models introduce a level of detail that addresses how data will interact within the system. They specify the attributes of each entity and define the relationships more clearly, without getting bogged down by implementation specifics.
A Logical Data Model is vital for:
- Outlining data attributes: Each entity will be further detailed with attributes that describe its properties.
- Defining relationships: This includes specifying one-to-one, one-to-many, or many-to-many relations, crucial for understanding data connectivity.
- Standardizing definitions: It ensures that all parties involved have a common understanding of data definitions.
As you can see, this model paves the way for creating a structured, coherent data management strategy that enhances the ability of teams to prepare for implementation.
Physical Data Models
The Physical Data Model is where theory meets reality. This model translates logical designs into actual database structures, incorporating the specifics necessary for database management systems. It considers factors like indexing, physical storage requirements, and other database optimization strategies.
In implementing a Physical Data Model, various aspects need attention, such as:
- Database Management System (DBMS) considerations: Understanding how the chosen DBMS handles data storage and retrieval is key. Common systems include Oracle, MySQL, and Microsoft SQL Server.
- Performance tuning: Implementing indexes and optimizing queries to enhance speed and efficiency in data retrieval will make a significant difference in usability.
- Security aspects: Addressing the security measures around data access and constraints to protect sensitive information.
"The effectiveness of a data model directly influences the quality of data-driven decisions. Therefore, choosing the right model ensures that organizations can manage and utilize their data efficiently."
Implementing Erwin Modeling in Organizations
Implementing Erwin Modeling is crucial for organizations looking to harness the power of their data assets. In today’s fast-paced business environment, having a clear data strategy isn’t just helpful, it’s necessary. Companies that turn data into actionable insights often find themselves ahead of the competition. Hence, Erwin Modeling presents a structured way to manage and visualize data, aligning it with business objectives.
With its comprehensive tools and frameworks, Erwin helps in mapping out data flows and understanding relationships across various data points. This understanding is fundamental for effective data governance, compliance, and strategic planning. After all, if you don't know where your data is coming from or how it's structured, how can you even begin to utilize it effectively?
Framework for Successful Implementation
A successful implementation of Erwin Modeling consists of several steps that ensure both immediate and long-term benefits. Here are some key elements to consider:
- Assessment of Current Data Practices: Before diving into Erwin, organizations should evaluate their existing data landscape. This assessment helps identify gaps and opportunities for improvement.
- Stakeholder Engagement: Involving key stakeholders from different departments fosters collaboration. It’s essential to gather insights from those who will interact with the data on a regular basis.
- Defining Clear Goals: Establishing clear, measurable goals is vital. Without direction, efforts may become scattered and lack focus.
- Training and Development: Investing in training ensures that team members are well-equipped to utilize Erwin's tools effectively. Training sessions should cover not just operational aspects, but also the strategic importance of data modeling.
- Iterative Process: Implementing Erwin should not be a one-off project. Instead, organizations should approach it as an iterative process—one that continuously evolves based on feedback and changing business needs.
Key Considerations and Best Practices
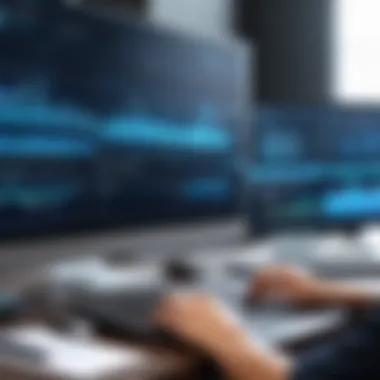
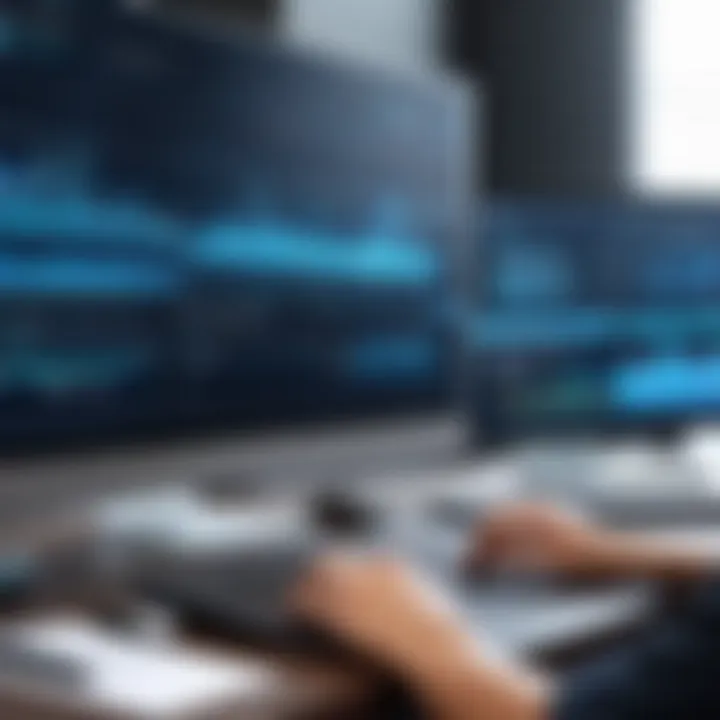
When implementing Erwin, several critical considerations and best practices should be kept in mind:
- Customization: The flexibility of Erwin allows users to tailor models according to specific business requirements, ensuring that data governance aligns perfectly with unique industry needs.
- Documentation: Maintaining thorough documentation of data models is essential. This practice not only aids in compliance but also serves as a valuable reference for future projects.
- Regular Audits: Conducting regular audits of the data models helps to ensure accuracy and relevancy. As business environments are dynamic, static data models can quickly become obsolete.
"An effective model is like a detailed map; it should guide decisions and actions, not become a mere artifact."
- Integration with Existing Systems: Ensuring that Erwin integrates seamlessly with current data management systems can prevent silos and enhance data flow.
- Fostering a Data-Driven Culture: For Erwin Modeling to truly succeed, organizations should promote a culture that values data-driven decisions at every level. This cultural shift can take time but is crucial for maximizing the benefits of any data strategy.
Implementing Erwin Modeling is not merely a technical endeavor; it represents a shift toward utilizing data as a strategic asset. By carefully considering the framework for implementation and following best practices, organizations can position themselves for success in an industry that increasingly relies on accurate, actionable data.
Case Studies: Erwin in Action
The significance of delving into case studies involving Erwin Modeling cannot be overstated. These real-world examples not only illuminate the theoretical aspects discussed earlier in the article but also showcase how organizations effectively apply Erwin tools to tackle their data challenges. By investigating these instances, decision-makers and IT professionals can gain insights into practical implementations, allowing them to envision similar strategies in their own contexts.
Industry Applications of Erwin Modeling
Erwin Modeling finds its footing across diverse industries, each harnessing its potential to streamline operations and enhance decision-making. Here are some notable examples:
- Finance: In the financial sector, data integrity is paramount. A bank utilized Erwin to develop a unified data model, integrating various sources such as customer accounts, transactions, and regulatory requirements. This streamlined reporting processes, enabling compliance and enhancing customer service through better data visibility.
- Healthcare: A hospital network deployed Erwin to manage the complex interrelations of patient records, treatment histories, and clinical outcomes. They could better support data-sharing across departments. This unity improved care delivery and reduced redundancy in data collection.
- Retail: A leading retail chain adopted Erwin Modeling to optimize inventory management. By mapping out data relationships between suppliers, inventory levels, and customer demand, they achieved significant reductions in stockouts and improved sales forecasting accuracy.
Each of these cases demonstrates not just the versatility of Erwin tools, but their tangible impact on operational efficiency and organizational success.
Measuring Success: Outcomes and Benefits
Evaluating the returns on investment from Erwin Modeling is essential for organizations to justify their efforts. Here’s what several businesses have realized post-implementation:
- Enhanced Data Quality: Many organizations reported a notable improvement in data accuracy and consistency. As they structured their data more effectively, the likelihood of errors diminished.
- Operational Efficiency: Streamlined processes led to faster decision-making and less time wasted on data retrieval and analysis. For instance, the hospital case study revealed a 30% reduction in the time taken for data reporting.
- Improved Compliance and Risk Management: By consistently applying data governance standards, companies not only mitigated risks associated with data breaches but also aligned their operations with regulatory requirements. This application was particularly crucial in finance and healthcare sectors.
"Organizations that effectively utilize data management tools can expect to see enhanced performance metrics, including operational speed and compliance rates."
While these outcomes can vary based on specific applications, the overarching theme remains clear: the integration of Erwin Modeling translates into measurable improvements. Organizations are not just adopting a tool; they are committing to a transformative journey in their data management processes.
These case studies serve as touchstones to drive home the point: successful implementation of Erwin tools can revolutionize how organizations manage their data, leading to strategic advantages in an increasingly data-driven world.
The Future of Erwin Modeling
The landscape of data management is ever-evolving, and with that, the tools we rely on must keep pace. Erwin Modeling is no exception. As organizations move towards more agile frameworks, adapting to new demands from both a technology and business perspective, the future of Erwin Modeling stands to reflect those changes. This section delves into what lies ahead for Erwin, highlighting the emerging trends and innovations that will shape its trajectory.
Emerging Trends in Data Management
The data management sector is witnessing several trends that are set to redefine how modeling tools, including Erwin, operate. Here are some key trends:
- Cloud Data Management: As organizations migrate to the cloud, there's a pressing need for tools that can facilitate and streamline this transition. Erwin is likely to enhance its cloud functionalities, enabling users to model and manage their data seamlessly in cloud environments.
- Data Governance: With compliance issues becoming a hot topic, companies are increasingly turning their eyes towards data governance. Erwin can tap into this trend by integrating stronger governance frameworks that help users track how data is utilized and ensure adherence to various regulations.
- Artificial Intelligence Integration: AI’s growing influence on data analysis hints at a future where Erwin could incorporate AI to enhance decision-making. This would enable predictive analytics and smarter data patterns that can lead to stronger insights.
- Automation in Data Management: Automation is no longer a luxury; it's a necessity. Day-to-day operations can be streamlined further through intelligent automation, making the data lifecycle more efficient. A focus on automating repetitive tasks in the Erwin suite could lead to significant time savings for data professionals.
These trends illustrate how dynamic the data management environment is, and Erwin Modeling will need to adjust to remain relevant and effective.
Innovations in Erwin Tools
Looking ahead, innovation will be paramount for Erwin to maintain its competitive edge. Here’s a sneak peek into potential innovations:
- Enhanced User Experience: Usability remains a cornerstone in tool adoption. Future updates to Erwin's interface are expected to make the tools even more user-friendly, accommodating both seasoned and novice users alike. Simplified navigation and intuitive visuals can help users get the most out of the software without extensive training.
- Collaborative Features: The future of work calls for collaboration across departments. Erwin may leverage this by integrating tools that facilitate group work, such as shared dashboards and real-time data updates, supporting decision-making across teams.
- Integration with Emerging Technologies: As new technologies, like IoT and blockchain, become integral to data management, Erwin will need to embrace these innovations. This might include creating specific templates or models tailored to these technologies that allow businesses to adapt more quickly.
- Advanced Data Analytics: As data volumes explode, it is imperative for modelling tools to offer sophisticated analytics capabilities. Erwin could work towards embedding advanced reporting functions that provide real-time insights and predictive data analysis.
"Adapting to new data realities will be key for Erwin's future growth, as businesses must understand and utilize their data better than ever."
These innovations not only set the stage for Erwin's competitive positioning but also ensure that it meets the emerging needs of businesses navigating the increasingly complex data landscape.
Epilogue: The Strategic Advantage of Erwin Modeling
In the ever-evolving landscape of data management, Erwin Modeling stands out as a pivotal tool for businesses striving to maintain a competitive edge. Its significance cannot be understated, as it delivers a structured approach to data governance, making substantial contributions towards enhancing organizational efficiency and decision-making processes.
One of the primary benefits of utilizing Erwin Modeling is its ability to enhance clarity in data representation. By providing a visual depiction of complex data relationships and structures, it allows IT professionals and decision-makers to grasp critical insights without the fog of ambiguity that often accompanies data management tasks. Clarity leads to informed decisions, which in turn fuel strategic initiatives and drive organizational growth.
Additionally, the integration capabilities of Erwin Modeling with various technologies promote a seamless flow of information across departments. This synergy reduces data silos—a common pain point that many organizations face—thereby facilitating a more agile and responsive data environment. Just as a well-oiled machine operates efficiently, so too does a well-integrated data model enable organizations to adapt swiftly to changing market conditions.
Another advantage lies in its robust compliance features. As regulations around data continue to tighten, having a reliable tool like Erwin Modeling ensures that organizations remain compliant without sacrificing operational efficiency. It empowers companies to keep their data practices transparent and accountable, which is becoming increasingly essential in the eyes of consumers and stakeholders alike.
Key Considerations
Here are several key elements to ponder when reflecting on the strategic advantage of Erwin Modeling:
- Data Quality Improvement: The rigorous frameworks included in Erwin help identify and rectify data integrity issues before they escalate.
- Scalability: As businesses grow, so too does their data. Erwin’s modeling tools are designed to scale, ensuring future needs are anticipated and met.
- User-Friendly Features: Simplifying complex data into manageable visual models allows various team members, regardless of technical expertise, to participate in data discussions.
- Cross-Functional Collaboration: By offering a common platform that can be used by different stakeholders, it fosters a collaborative environment necessary for comprehensive data governance.
"In data management, clarity is king; only then can the true potential of data be realized."