Unleashing the Power of Top Analytical Tools for Data Analysis Excellence
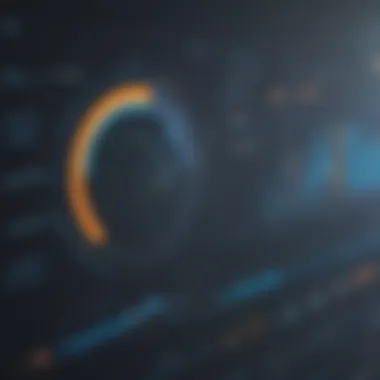
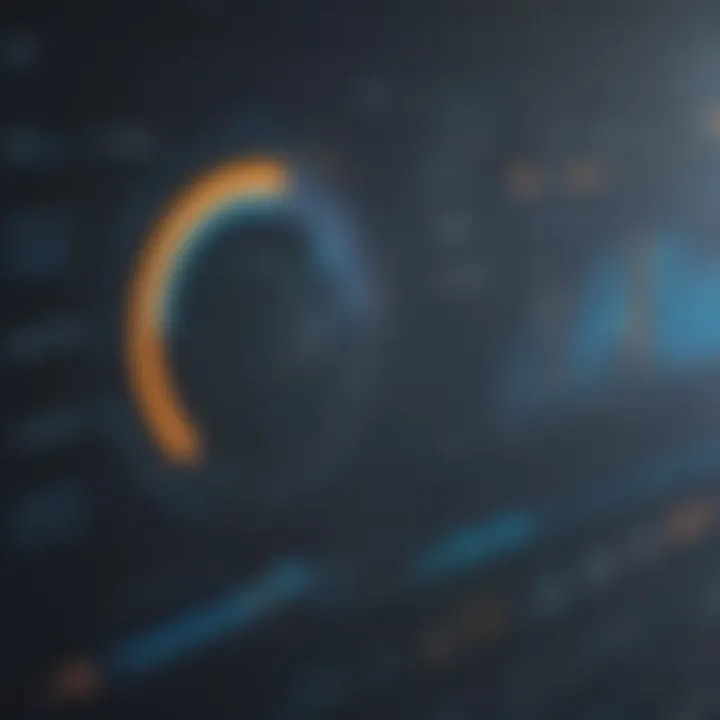
Industry Overview
When delving into the realm of data analysis, understanding the current trends in analytical tools is crucial for informed decision-making. The industry-specific software market is witnessing rapid advancements in data visualization and statistical analysis tools that cater to the evolving needs of businesses. Key challenges faced by buyers include the overwhelming options available and the complexity of choosing the right tool for their specific requirements. Additionally, emerging technologies like artificial intelligence and machine learning are revolutionizing the landscape, offering innovative solutions to complex data analysis problems.
Top Software Options
A thorough review of leading software providers in the industry reveals a diverse range of analytical tools designed to streamline data analysis processes. Conducting a feature comparison of these top software solutions showcases the unique strengths and capabilities of each platform, allowing buyers to make informed decisions based on their specific needs. Moreover, understanding the pricing structures of different software options is essential for budget planning and aligning investment with expected outcomes.
Selection Criteria
When selecting analytical tools for effective data analysis, several important factors must be considered to ensure optimal performance and functionality. Avoiding common mistakes during the selection process, such as overlooking compatibility with existing systems or underestimating training requirements, is paramount for successful implementation. Exploring how to determine the right fit for your business needs involves aligning software features with strategic objectives and long-term goals, ensuring a seamless integration within the organization.
Implementation Strategies
Deploying analytical software solutions requires meticulous planning and execution to avoid disruptions to existing workflows. Best practice guidelines for smooth implementation include thorough testing, stakeholder engagement, and a phased rollout approach. Integration with existing systems and processes is essential for seamless data flow and analysis, enhancing overall operational efficiency. Moreover, providing comprehensive training and support resources to end-users ensures successful deployment and adoption of the new software solutions.
Future Trends
Predicting the future of the industry-specific software landscape involves analyzing upcoming technologies that will shape data analysis processes. As businesses strive to future-proof their technology stack, recommendations for staying ahead of trends and adopting innovative solutions become indispensable. By embracing emerging technologies and aligning software investments with strategic objectives, organizations can leverage data-driven insights for sustainable growth and competitive advantage.
Introduction to Analytical Tools
Analytical tools form the backbone of effective data analysis, serving as the linchpin for extracting valuable insights from vast and complex datasets. In this article, we embark on a comprehensive exploration of the top analytical tools that have revolutionized the landscape of data-driven decision-making. These tools are instrumental in converting raw data into actionable intelligence, facilitating informed decision-making processes across various industries.
Understanding the Significance of Analytical Tools
Evolution of Data Analysis
The evolution of data analysis marks a significant paradigm shift in how organizations harness the power of data. By delving into historical data patterns and trends, businesses can uncover valuable insights that inform strategic decision-making processes. The evolution of data analysis empowers organizations to make data-driven decisions based on concrete evidence rather than mere intuition. Its ability to track changes over time and predict future outcomes makes it a vital component in the analytical toolkit, enabling businesses to stay ahead in an increasingly competitive landscape.
Role of Analytics in Decision Making
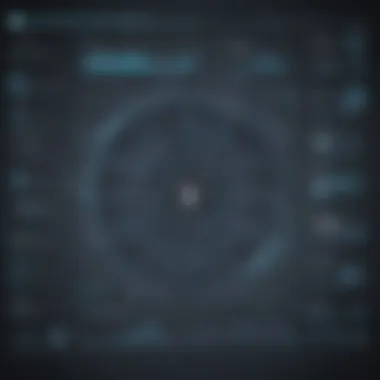
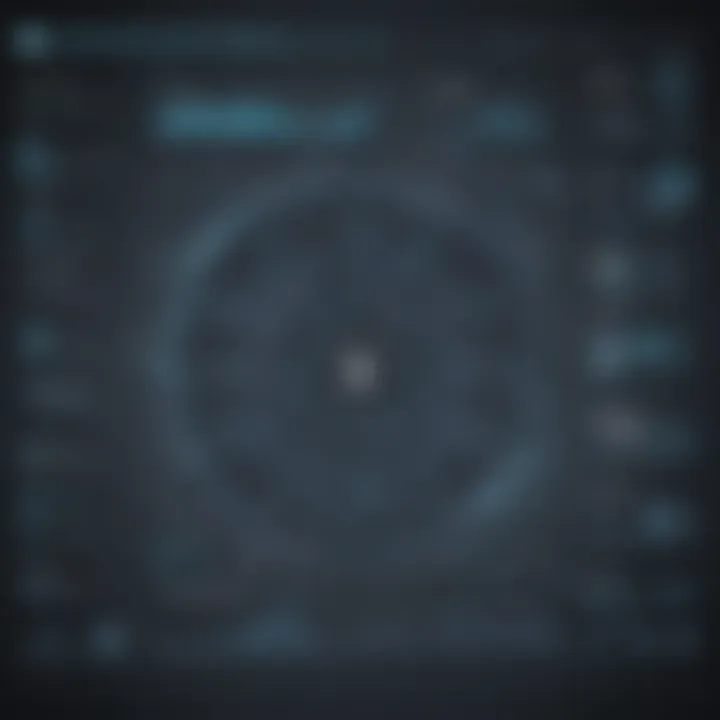
Analytics plays a pivotal role in shaping decision-making processes within organizations. By leveraging sophisticated algorithms and statistical models, analytics equips decision-makers with the tools needed to make informed choices. The role of analytics in decision making extends beyond mere number crunching; it enables executives to forecast trends, identify patterns, and mitigate risks effectively. The seamless integration of analytics into the decision-making framework empowers businesses to drive innovation, optimize processes, and gain a competitive edge in the market.
Key Features to Look for in Analytical Tools
Scalability
Scalability stands as a cornerstone feature in analytical tools, allowing organizations to handle growing volumes of data seamlessly. A scalable tool adapts to increasing data loads without compromising performance, ensuring smooth operations even in the face of expanding datasets. The ability to scale horizontally and vertically makes scalability a sought-after trait in analytical tools, empowering organizations to maintain efficiency and productivity as data volumes increase.
Data Integration Capabilities
Data integration capabilities enable seamless data aggregation from disparate sources, offering a unified view for analysis. By consolidating data from various platforms and formats, analytical tools with robust integration features alleviate the challenges posed by siloed information. The seamless integration of data enhances data quality, reduces errors, and accelerates the decision-making process, underpinning the effectiveness of analytical solutions.
Visualization Options
Visualization options play a vital role in data analysis by transforming complex datasets into intuitive and actionable visual representations. An analytical tool's visualization capabilities determine its ability to communicate insights effectively. By presenting data through interactive charts, graphs, and dashboards, visualization options facilitate data interpretation and decision-making. The visual storytelling enabled by robust visualization features enhances comprehension and enables stakeholders to glean insights at a glance.
Impact of Analytical Tools on Business Performance
Enhanced Decision-Making
Analytical tools empower organizations to make data-driven decisions, fostering a culture of evidence-based reasoning and informed choices. The capacity to derive actionable insights from data enhances decision-making processes, enabling organizations to pivot swiftly in response to market dynamics. Enhanced decision-making catalyzes agility and flexibility, propelling businesses towards strategic goals and operational excellence.
Operational Efficiency
Operational efficiency is bolstered by the implementation of analytical tools that streamline processes and eliminate inefficiencies. By automating routine tasks, optimizing workflows, and identifying bottlenecks, analytical tools enhance operational effectiveness. The data-driven insights generated by these tools offer actionable recommendations for process enhancement, resource allocation, and performance optimization, contributing to overall operational efficiency.
Competitive Advantage
Analytical tools confer a competitive advantage upon organizations by fostering a culture of continuous improvement and innovation. By leveraging advanced analytics, businesses can uncover untapped opportunities, optimize growth strategies, and stay ahead of market trends. The insights derived from analytical tools enable organizations to respond proactively to industry changes, anticipate customer needs, and differentiate their offerings, solidifying their market position.
This meticulous exploration of the foundational elements and implications of analytical tools sets the stage for a deeper dive into the specifics of key tools and their applications. Stay tuned as we unravel the dynamic landscape of data analysis and its transformative impact on businesses.
Popular Analytical Tools in the Market
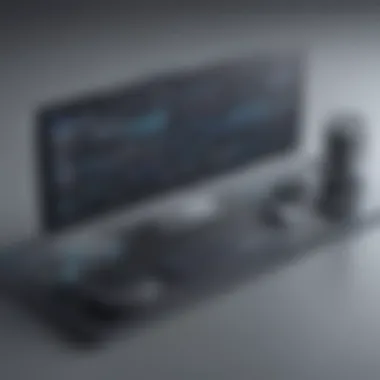
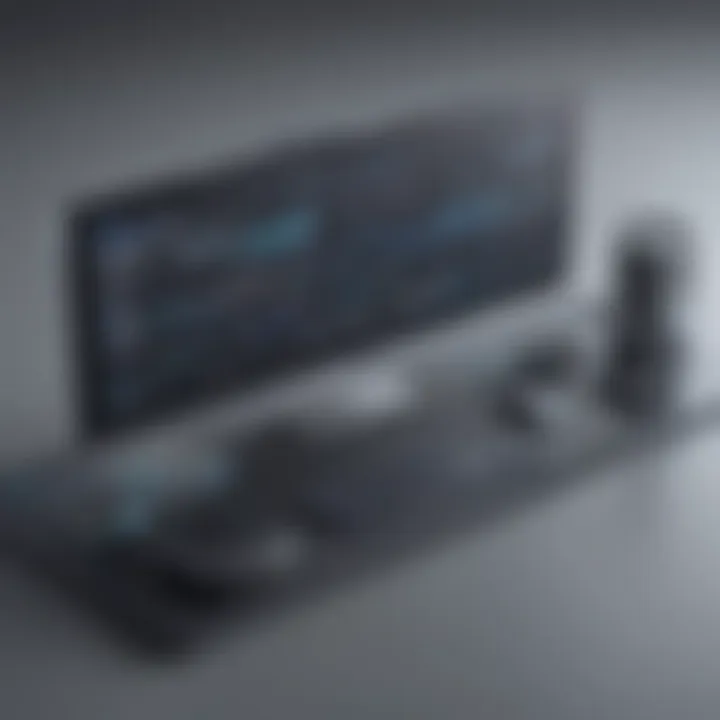
Analytical tools are indispensable in today's data-driven world, enabling organizations to extract valuable insights from complex datasets. Among the myriad of analytical tools available, some stand out for their efficiency and effectiveness. These tools streamline the data analysis process, enhance decision-making, and ultimately drive business success. Understanding the landscape of popular analytical tools in the market is crucial for organizations looking to leverage data effectively.
Tableau
Tableau is a powerhouse in the realm of data analysis, renowned for its interactive data visualization capabilities. With Tableau, users can create visually appealing and insightful dashboards that aid in understanding data trends quickly and intuitively. The tool's strength lies in its ability to transform raw data into comprehensible visuals, empowering users to uncover patterns and derive meaningful conclusions. Interactive data visualization not only simplifies complex data sets but also fosters data-driven decision-making by presenting information in a digestible format.
Python
Python, as a versatile programming language, plays a pivotal role in data analytics. Its rich ecosystem of libraries offers a wide range of functionalities, making Python a go-to choice for data manipulation, analysis, and visualization. The language's flexibility and simplicity allow users to perform various data tasks efficiently, from cleaning and preprocessing datasets to building sophisticated models. The diverse applications of Python, coupled with its scalability and ease of use, make it a highly preferred tool for data analysis across industries.
Power BI
Power BI is synonymous with intuitive reporting, providing organizations with dynamic and interactive reporting capabilities. The tool empowers users to create visually compelling reports and dashboards that convey information effectively. Its seamless integration with Microsoft technologies enhances the reporting process, enabling users to access and analyze data from multiple sources effortlessly. By offering real-time insights and fostering collaboration through data sharing, Power BI simplifies the data analysis journey for users of all levels.
R Programming
R Programming is renowned for its statistical analysis capabilities, making it a vital tool for data scientists and statisticians. With R, users can conduct in-depth statistical analyses, generate advanced visualizations, and build predictive models with ease. The tool's extensive library of packages amplifies its capabilities, enabling users to explore complex data sets and extract valuable insights. R's robust data modeling features facilitate accurate predictions and enable data-driven decision-making, contributing significantly to the field of data analysis.
Advanced Analytical Tools for Specialized Functions
dvanced analytical tools play a pivotal role in enhancing data analysis capabilities, particularly when dealing with complex datasets. These tools are designed to tackle specialized functions that traditional analytical tools may struggle to address effectively. By leveraging advanced tools, organizations can delve deeper into their data and extract valuable insights that can drive impactful decision-making processes and improve overall operational efficiency. In this section, we will explore the significance of advanced analytical tools and the specific elements, benefits, and considerations associated with them.
Utilizing Machine Learning with TensorFlow
hen it comes to harnessing the power of machine learning for advanced analytics, TensorFlow stands out as a popular and versatile tool. TensorFlow empowers users to implement deep learning techniques effectively, offering sophisticated capabilities for model training and deployment. Let's delve into two key aspects of TensorFlow that contribute significantly to its value proposition.
Deep Neural Network Training
eep Neural Network Training is a fundamental aspect of machine learning that enables the creation of complex models capable of understanding and analyzing intricate patterns within data. TensorFlow excels in this area by providing a robust framework for building and training deep neural networks, ensuring high model accuracy and performance. The unique feature of TensorFlow's Deep Neural Network Training lies in its ability to handle massive datasets with efficiency, making it a preferred choice for data-intensive projects.
High-performance Prediction Models
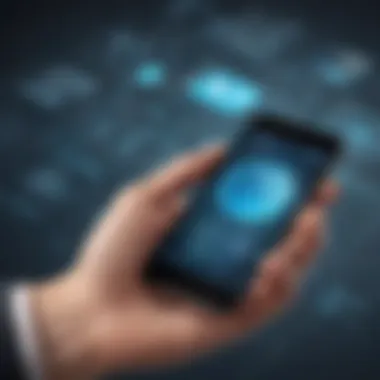
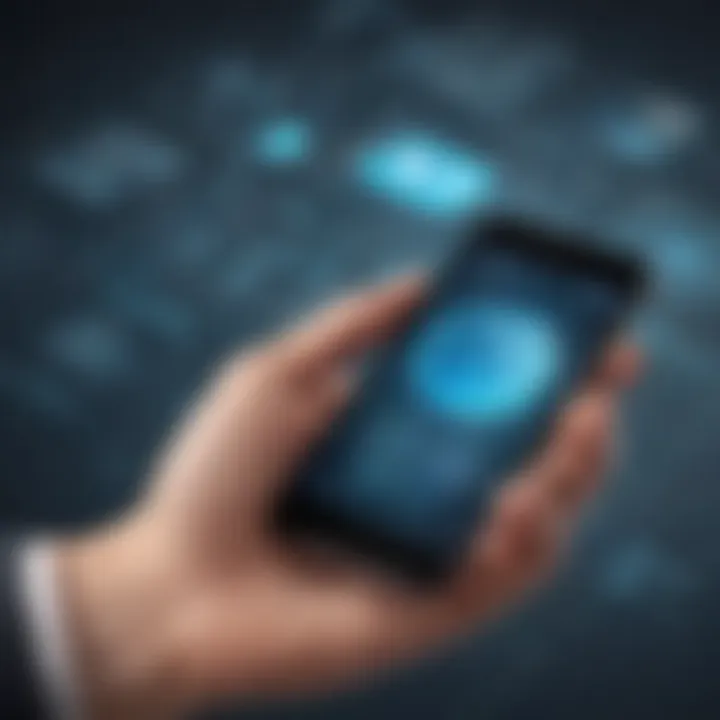
nother compelling feature of TensorFlow is its proficiency in developing high-performance prediction models that deliver accurate forecasts and insights. By leveraging advanced algorithms and optimization techniques, TensorFlow empowers data scientists to build models that excel in predictive accuracy and scalability. The key characteristic of TensorFlow's High-performance Prediction Models lies in their ability to adapt to diverse data scenarios, making them a versatile and reliable choice for a wide range of analytical tasks.
Enhancing Data Security with Apache Spark
nsuring robust data security is paramount in today's data-driven landscape, and Apache Spark plays a crucial role in enhancing security measures while enabling real-time data analytics. In this section, we will explore two key aspects of Apache Spark that make it indispensable for safeguarding sensitive data.
Distributed Processing Capabilities
pache Spark's Distributed Processing Capabilities empower organizations to process large-scale data sets across distributed computing clusters efficiently. By parallelizing data processing tasks, Apache Spark enhances data processing speed and scalability, enabling real-time analytics and rapid decision-making. The key characteristic of Apache Spark's Distributed Processing Capabilities lies in its ability to handle massive volumes of data seamlessly, making it an ideal choice for high-throughput data processing.
Real-time Data Analytics
eal-time Data Analytics is a critical feature of Apache Spark that enables organizations to analyze and derive insights from streaming data in real-time. By supporting continuous data processing, Apache Spark allows businesses to gain immediate visibility into changing trends and patterns, facilitating agile decision-making and proactive responses. The unique feature of Apache Spark's Real-time Data Analytics is its capability to process and analyze data streams without delays, ensuring timely and actionable insights.
Optimizing Data Warehousing with Snowflake
ata warehousing plays a crucial role in storing and managing vast amounts of structured and unstructured data. Snowflake, a cloud-based data warehousing solution, offers advanced features for optimizing data storage and accessibility. In this section, we will delve into two key aspects of Snowflake that enhance data warehousing capabilities.
Elastic Data Scaling
nowflake's Elastic Data Scaling feature enables organizations to scale their data storage and processing resources dynamically based on demand. By automatically adjusting computing resources to accommodate varying workloads, Snowflake ensures optimal performance and cost efficiency. The key characteristic of Snowflake's Elastic Data Scaling lies in its ability to provide seamless scalability without the need for manual intervention, making it a versatile and cost-effective solution for data warehousing.
Efficient Data Sharing
fficient Data Sharing is another key strength of Snowflake, allowing users to share data securely and collaborate with partners and stakeholders effortlessly. By providing granular control over data access and permissions, Snowflake enables organizations to maintain data integrity while facilitating seamless data sharing and exchange. The unique feature of Snowflake's Efficient Data Sharing is its ability to streamline data collaboration processes and enhance overall productivity, making it a valuable asset for organizations seeking efficient data management solutions.
Trends Shaping the Future of Analytical Tools
Tackling the subject of Trends Shaping the Future of Analytical Tools is paramount within this article. As the landscape of data-driven decision-making continues to evolve, staying ahead of emerging trends becomes integral to maintaining a competitive edge. In the ever-expanding realm of analytics, AI-Powered analytics is paving the way for transformative capabilities that harness the power of artificial intelligence to drive decision-making processes. The integration of IoT data is another crucial trend, allowing real-time sensor data analysis to provide invaluable insights for predictive maintenance solutions. Furthermore, the focus on data governance and privacy underscores the importance of compliance with regulatory standards and transparent data handling practices, signaling a shift towards more responsible and accountable data management strategies.
AI-Powered Analytics
Cognitive Insights Generation
Delving into the specifics of Cognitive Insights Generation sheds light on its pivotal role within the overarching theme of AI-Powered Analytics. This facet emphasizes the generation of cognitive insights through advanced algorithms and machine learning techniques, enabling organizations to extract deeper, more nuanced insights from their datasets. The key characteristic of Cognitive Insights Generation lies in its ability to uncover patterns and trends that may not be immediately apparent to human analysts, thereby enhancing the decision-making process significantly. The unique feature of Cognitive Insights Generation lies in its capacity to augment human intelligence with machine-driven analysis, leading to more informed and data-driven decisions. While its advantages include enhanced predictive capabilities and increased efficiency, potential disadvantages may stem from over-reliance on automated insights, overlooking subtle contextual nuances that human judgment may catch.
Automated Data Preparation
Exploring Automated Data Preparation within the realm of AI-Powered Analytics reveals its crucial contribution to the efficiency and accuracy of data processing workflows. Automated Data Preparation streamlines the preparation phase of data analysis by automating tasks such as data cleansing, normalization, and structuring, reducing the time and effort required for data pre-processing significantly. The key characteristic of Automated Data Preparation lies in its ability to accelerate data readiness for analysis, enabling organizations to derive insights rapidly and effectively from complex datasets. The appeal of Automated Data Preparation in this article stems from its reputation as a time-saving and error-reducing solution in the data analysis pipeline. Its unique feature lies in its capacity to handle large volumes of data efficiently while minimizing human errors typically associated with manual data preparation. Despite its advantages in boosting analytical efficiency and scalability, potential disadvantages may arise from algorithmic biases leading to inaccuracies in data processing.