Understanding Digital AI: Impact and Future Trends
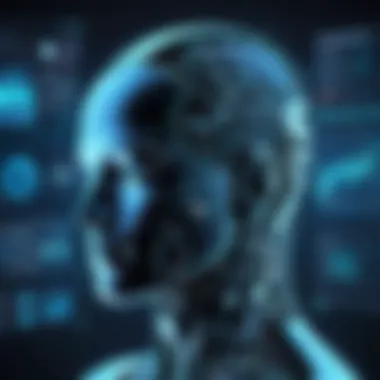
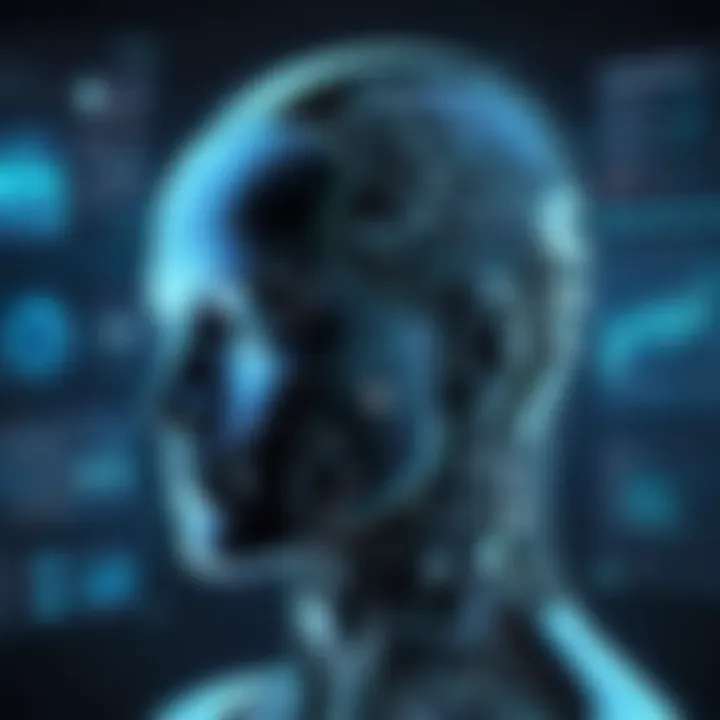
Intro
Digital AI represents a pivotal shift in the technological landscape. Its transformative power extends beyond mere automation, influencing diverse sectors including healthcare, finance, and manufacturing. This section will provide an overview that highlights vital trends, challenges, and the emerging technologies that shape this dynamic environment.
Understanding Digital AI requires grasping its broad implications on social, economic, and ethical dimensions. Decision-makers, IT professionals, and entrepreneurs must navigate these complexities to harness AI effectively for strategic advantages. The following narrative will unfold the essential facets of this ever-evolving field, guiding readers through the critical considerations in deploying AI solutions.
Industry Overview
The digital AI industry is continuously evolving, with new trends and challenges arising daily.
Current Trends in the Industry-specific Software Market
As businesses adapt to the digital age, several trends have emerged:
- Increased Integration of Machine Learning: Many companies are embedding machine learning systems within their processes to enhance decision-making.
- Data-Driven Solutions: Organizations prioritize data analytics to inform AI-driven strategies.
- Emphasis on Customization: Firms are turning towards tailored AI systems specific to business needs rather than generic solutions.
Key Challenges Faced by Buyers in the Industry
While the benefits of Digital AI are compelling, buyers encounter significant obstacles:
- Understanding Complexity: The intricate nature of AI technologies can overwhelm decision-makers.
- Data Concerns: Issues related to data privacy and security continuously challenge businesses.
- Integration Difficulties: Merging AI solutions with existing systems often proves problematic, affecting overall performance.
Emerging Technologies Impacting the Industry
Innovative technologies are pivotal to the advancement of Digital AI. Notable players include:
- Natural Language Processing: Enhances communication between humans and machines.
- Robotic Process Automation: Streamlines repetitive tasks, improving efficiency significantly.
- Blockchain: Offers secure and transparent transactions, crucial in data management.
"To navigate the evolving landscape of Digital AI, businesses must remain agile and responsive to technological advancements and market demands."
This overview sets the stage to explore software options, selection criteria, implementation strategies, and future trends, providing a comprehensive understanding of Digital AI's landscape.
Defining Digital AI
Defining Digital AI is a crucial step in understanding its broader impact on society and various industries. As Artificial Intelligence (AI) continues to evolve, it is vital for professionals to grasp what Digital AI encompasses. This section outlines its core components, benefits, and the context in which it operates.
What Constitutes Digital AI
Digital AI refers to the subset of Artificial Intelligence technologies that operate primarily in a digital landscape. Unlike traditional AI, which may rely on physical systems or simple algorithms, Digital AI takes advantage of vast amounts of data and computational power to enhance decision-making and automate processes. Key components include:
- Machine Learning: Algorithms that learn from data input and improve performance over time.
- Natural Language Processing (NLP): Enables machines to understand, interpret, and generate human language.
- Computer Vision: Allows machines to interpret visual information from the world.
The convergence of these technologies leads to applications in diverse fields, including finance, healthcare, and marketing.
Distinction Between Digital AI and Traditional AI
Understanding the distinction between Digital AI and Traditional AI is essential for decision-makers and IT professionals.
- Nature of Data: Traditional AI systems often rely on structured data; Digital AI thrives on unstructured and semi-structured data that is abundant in today's digital ecosystem.
- Complexity and Scalability: Digital AI systems are designed to handle complex tasks at scale, utilizing advanced algorithms and massive datasets. Traditional AI, in contrast, may struggle with scalability and complexity.
- Learning Capabilities: While traditional AI systems may perform designated tasks effectively, Digital AIs can continually learn and adapt based on new information and changing environments.
This differentiation is vital for entrepreneurs and businesses aiming to leverage AI technologies for innovation and efficiency.
By defining Digital AI and understanding its components and distinctions, professionals can better appreciate the transformative potential of AI-driven solutions. This knowledge equips organizations to implement strategies that harness Digital AI effectively for future growth.
Historical Context of Digital AI
Understanding the historical context of Digital AI is essential to appreciate its current impact and future trajectory. By exploring the evolution of artificial intelligence technologies, we can gain insights into the foundational concepts that have shaped modern Digital AI. This section will unpack the advancements made over decades, paving the way for the sophisticated automation and intelligent systems we see today. Recognizing these milestones provides clarity on how past innovations influence today's applications and ethical considerations, essential for any professional navigating this complex field.
Evolution of Artificial Intelligence Technologies
Artificial Intelligence began its journey in the mid-20th century. The initial aspirations were focused on creating machines that could mimic human reasoning. Early programs, like the Logic Theorist and the General Problem Solver developed by Allen Newell and Herbert A. Simon, demonstrated rudimentary problem-solving capabilities. These programs laid the groundwork for future explorations into machine learning and AI algorithms.
Through the 1980s and 1990s, the field experienced significant transformations with the introduction of various knowledge-based systems and expert systems. These systems functioned through rule-based logic but faced limitations in scalability and adaptability. The advent of statistical machine learning in the late 1990s changed the landscape. Techniques such as support vector machines and decision trees began to emerge, leading to more robust models that could learn from vast datasets, a precursor to current machine learning algorithms.
Notably, the release of more powerful computational resources and the availability of extensive datasets propelled growth in this area. With the introduction of neural networks and deep learning in the early 2000s, AI technologies witnessed a renaissance, enabling significant advancements in natural language processing and computer vision.
Milestones in AI Development
The history of AI is marked by key milestones that signify leaps in technology and application. Each phase has contributed to forming a more interconnected digital ecosystem. Here are some notable developments:
- 1956 Dartmouth Conference: This meeting is considered the birth of artificial intelligence as a field. Researchers gathered to discuss how machines could be made to simulate intelligent behavior.
- 1980s Expert Systems: The rise of expert systems showcased the practical applications of AI in industries like finance and medicine, marking a shift toward commercial use.
- 1997 Deep Blue: IBM's chess-playing computer defeated world champion Garry Kasparov, highlighting AI's potential in complex decision-making processes.
- 2012 ImageNet: The introduction of convolutional neural networks dramatically improved image recognition, enhancing the capabilities of computer vision within AI systems.
- 2020 GPT-3: OpenAI's language model demonstrated unprecedented language understanding and generation, showcasing the breadth of applications for AI in communication and creative tasks.
The evolution of these technologies signals that Digital AI is not a static concept but an ever-evolving discipline that reflects advancements in science, data, and computational power.
"The AI revolution is the most profound technological transformation ever seen. It reshapes industries and society in ways we are still discovering."
Core Technologies Behind Digital AI
The integration of core technologies is essential for the evolution and implementation of Digital AI. These technologies are the backbone that supports the sophisticated algorithms and processes necessary for AI operations. A deep understanding of these tech elements is crucial for professionals and decision-makers who aim to harness Digital AI.
Machine Learning Algorithms
Machine Learning (ML) algorithms form a fundamental component in Digital AI. They enable systems to learn from data patterns without being explicitly programmed. This adaptability is invaluable in countless applications, from predictive analytics to personalized marketing.
The most common algorithms include supervised learning models, unsupervised learning, and reinforcement learning. Each serves distinct purposes:
- Supervised Learning: This involves training an algorithm on a labeled dataset, allowing it to make predictions on unseen data. For example, classification tasks in email filtering utilize such algorithms.
- Unsupervised Learning: It identifies hidden patterns in unlabeled data. Clustering users in marketing based on behavior is one key application.
- Reinforcement Learning: Here, the algorithm learns by receiving feedback from its actions. This is widely used in robotics and game AI.
These algorithms can provide significant benefits:
- Increased efficiency in data processing
- Enhanced accuracy in predictions
- Potential for automation in various tasks
However, considerations around complexity and computational requirements must be addressed.
Natural Language Processing
Natural Language Processing (NLP) bridges the communication gap between humans and machines. This technology enables machines to understand, interpret, and respond to human language in a valuable way. NLP is increasingly used in applications such as virtual assistants, chatbots, and sentiment analysis.
Key components of NLP include:
- Tokenization: The process of dividing text into words or phrases.
- Part-of-Speech Tagging: Identifying the function of each word in a sentence.
- Named Entity Recognition: Extracting relevant information such as names, dates, or locations.
With NLP, businesses can enhance customer interaction through automated responses, thereby increasing efficiency. Moreover, the technology can analyze customer sentiments, offering insights into market trends. This integration can foster better decision-making.
Yet, challenges remain, particularly in terms of contextual understanding and language nuances that can affect the accuracy of responses.
Computer Vision Techniques
Computer Vision is another crucial technology that allows machines to interpret and understand visual information. Through the analysis of images and videos, systems can perform functions such as object recognition, facial recognition, and scene understanding. This is particularly transformative for industries like retail, autonomous vehicles, and security.
Key applications include:
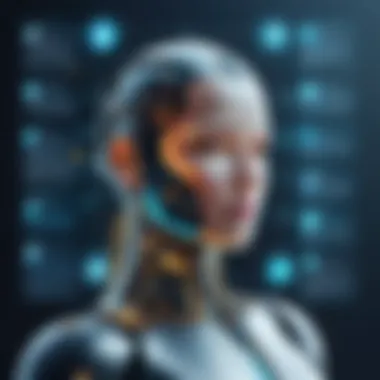
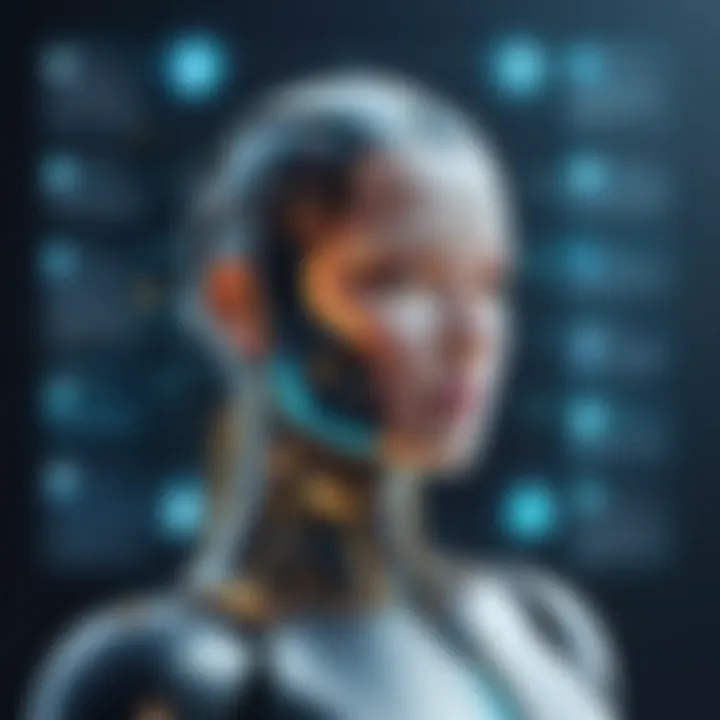
- Image Classification: Labeling images based on their content (e.g. identifying products in retail).
- Facial Recognition: Used for security and personalized user experiences in various platforms.
- Augmented Reality: Combining real-world imagery with digital elements, enhancing user experiences.
The benefits of Computer Vision are substantial:
- Improved accuracy in identifying elements within images
- Enhanced consumer insights through visual data analysis
- Automation in processes that require visual verification
Despite these advantages, technical limitations such as variability in image quality and lighting conditions need careful consideration.
"The successful application of these core technologies in Digital AI will define the scope of future innovations and societal changes."
Applications of Digital AI in Business
The integration of Digital AI within business operations marks a significant evolution in how companies manage their resources and interact with customers. This section aims to expose the various facets through which Digital AI can enhance business efficacy, promote engagement, and facilitate informed decision-making. Understanding these applications provides business leaders the insights necessary to leverage technology for competitive advantage and operational excellence.
Enhancing Customer Experience
In the evolving landscape of customer interactions, Digital AI plays a pivotal role in personalizing experiences. With tools like chatbots and recommendation systems, businesses can offer tailored solutions to individual preferences. For instance, companies like Amazon utilize predictive algorithms to suggest products based on previous purchases, significantly enhancing the user experience. Such practice not only fosters customer loyalty but also increases the likelihood of repeat purchases.
Another critical aspect is sentiment analysis, where AI analyzes customer feedback from various platforms. This data can unveil insights into customer satisfaction and inform service improvements. Thus, AI transforms raw data into actionable strategies, aligning business offerings more closely with consumer expectations.
Streamlining Operations
Operational efficiency remains a cornerstone for any successful business. Here, Digital AI introduces automated processes which enhance productivity while reducing resource waste. Businesses employ robotic process automation (RPA) to handle repetitive tasks like data entry, thus freeing personnel to focus on high-level strategic initiatives. Tasks that once required extensive manpower can be accomplished in a fraction of the time.
Furthermore, AI-driven data analysis tools enable companies to optimize supply chain management. These tools can predict inventory requirements, helping businesses avoid overstock and stockouts. Companies like Walmart implement such systems to minimize logistical costs and ensure product availability, which is essential for maintaining operational flow.
Data-Driven Decision Making
The landscape of decision-making has dramatically shifted from intuition-based strategies to data-driven methodologies, largely attributed to Digital AI. By harnessing large data sets and employing AI analytics, businesses can derive insights that guide strategic initiatives and mitigate risks. This allows for a granular understanding of market trends and consumer behavior.
Key performance indicators (KPIs) can be monitored through AI tools, providing real-time insights into the effectiveness of business strategies. For instance, Google Analytics utilizes machine learning to offer businesses detailed reports on web traffic and user engagement, enabling informed marketing decisions.
Digital AI in Healthcare
The healthcare industry has seen a remarkable transformation with the integration of Digital AI. Increasingly complex healthcare datasets and the growing need for personalized medicine have led to significant advancements in AI technologies. Digital AI plays a crucial role in enhancing patient outcomes and streamlining healthcare operations. By leveraging machine learning and data analytics, healthcare providers can make informed decisions, improve patient care, and reduce costs. The implications of these technologies extend to various aspects of healthcare including diagnostics, treatment options, and administrative processes.
Predictive Analytics in Patient Care
Predictive analytics is one of the most impactful applications of Digital AI in healthcare. This technology uses historical data and algorithms to predict future health outcomes. For instance, by analyzing patient history and demographic data, AI can identify individuals at high risk for conditions such as diabetes or heart disease. This capability allows healthcare providers to intervene early and implement preventive measures. The benefits are clear: early detection can lead to improved management of health conditions and potentially save lives.
Some key advantages of predictive analytics include:
- Improved Patient Outcomes: Timely interventions can prevent complications.
- Resource Optimization: Hospitals can allocate resources more effectively based on predicted patient inflow.
- Enhanced Personalization: Treatment plans can be tailored to individual needs, based on predictive insights.
Despite its advantages, the deployment of predictive analytics also poses challenges. Concerns regarding data privacy, as well as the accuracy of predictions, must be addressed. Ensuring access to comprehensive and high-quality data is also fundamental for the success of predictive models in patient care.
Automating Administrative Tasks
Another significant domain where Digital AI contributes in healthcare is through the automation of administrative tasks. Administrative overhead in healthcare settings is often substantial, ranging from patient scheduling to billing processes. AI can streamline these repetitive tasks, allowing healthcare professionals to focus more on patient care.
Automating tasks such as:
- Appointment Scheduling: AI can manage scheduling by predicting and accommodating patient needs effectively.
- Billing and Claims Processing: Automated systems can handle claims with high accuracy, reducing errors and the need for human intervention.
- Patient Data Management: AI technology can efficiently handle the storage, retrieval, and organization of medical records, ensuring easy access by healthcare providers.
"The integration of AI in administrative functions liberates valuable time for providers, allowing them to enhance patient interaction and care."
The transition to automation not only improves efficiency but also reduces operational costs. Nonetheless, careful consideration is needed when implementing these systems, particularly around employee training and the potential for job displacement.
By fully embracing Digital AI, healthcare organizations can enhance service delivery, improve efficiency, and provide high-quality patient care, shaping the future of healthcare systems worldwide.
The Ethical Implications of Digital AI
The rise of Digital AI brings profound ethical implications that merit serious attention. As the technology becomes integrated into various aspects of our lives and industries, the consequences of its use must be thoughtfully examined. These implications involve not only the direct interactions between AI systems and individuals but also broader societal impacts. Engaging with ethical considerations ensures the technology benefits society and mitigates potential harm.
Understanding the ethical dimension of Digital AI can guide decision-makers, IT professionals, and entrepreneurs in navigating its complexities. This can lead to a more responsible deployment of AI technologies that prioritize fairness, transparency, and security, thus fostering greater trust in AI systems.
Bias and Fairness in Algorithms
Bias in algorithms is a critical concern in the ethical landscape of Digital AI. Algorithms are not neutral; they reflect the data on which they are trained. Historical biases can become entrenched in AI systems, perpetuating discrimination. This may occur in recruitment processes, loan approvals, or law enforcement applications.
A study revealed that AI facial recognition systems often have higher error rates for people of color and women. This raises significant ethical questions. Do these systems ensure a just society, or do they exacerbate existing inequalities?
To mitigate bias, organizations must prioritize fairness in algorithm development. This requires:
- Diverse Data Sets: Training models on diverse datasets helps in recognizing and correcting bias.
- Regular Audits: Continuous monitoring of AI systems for biased outcomes ensures accountability.
- Stakeholder Involvement: Engaging various stakeholders in the design process can provide insight into potential biases.
Addressing bias and fairness is not just ethical but also beneficial for organizations. Fair algorithms can lead to better decision-making and enhance customer trust.
Privacy Concerns and Data Security
Privacy concerns are paramount when discussing Digital AI. AI systems often require vast amounts of personal data to function effectively. This reliance raises questions about individuals' rights regarding their data and how it is used. Security also links closely to privacy; data breaches can expose sensitive information, leading to financial and reputational damage.
The implementation of AI technologies must prioritize data privacy through several strategies:
- Transparency: Organizations should clearly communicate how data is collected, used, and stored. This builds trust with users.
- Data Minimization: Collecting only the necessary data helps in protecting individuals' privacy.
- Robust Security Protocols: Implementing strong data security measures prevents unauthorized access and data loss.
As we continue to harness Digital AI, balancing innovation with ethical considerations is crucial. This ensures a technology landscape that respects individual rights while still allowing for advancement and efficiency.
"Ethics in AI is not an afterthought. It must be part of its structure."
Future Directions of Digital AI
The future directions of Digital AI represent a crucial aspect of this article. This section will explore the evolving landscape of AI technologies and their anticipated impact on various domains. Understanding these directions is vital for businesses, policymakers, and tech enthusiasts alike, as they navigate the implications and benefits that Digital AI brings to society and industries.
Innovations on the Horizon
Innovations in Digital AI are expected to reshape industries profoundly. A few areas to look out for include:
- Advanced Personalization: Improving user experiences via tailored recommendations.
- Enhanced Automation: Streamlining processes in sectors like manufacturing and logistics.
- Augmented Reality and AI Integration: Merging AI with AR to create more immersive experiences.
The significance of these innovations lies in their potential to enhance efficiency and foster innovation across various sectors. The healthcare industry, for example, will benefit from AI-driven diagnostic tools that improve patient outcomes. Such advancements will not only streamline operations but also reduce costs, making healthcare more accessible.
Potential for Societal Impact
Digital AI holds the potential for substantial societal changes. Its effects may be felt in different aspects, including:
- Education: AI can provide personalized learning tools that cater to individual learning paces and styles.
- Workforce Dynamics: The introduction of AI may transform job roles, leading to new types of employment opportunities.
- Sustainability Initiatives: AI could help optimize resource usage, thus contributing to environmental sustainability efforts.
As organizations increasingly depend on AI, a conversation about its societal implications must take precedence. This includes understanding the balance between job creation and displacement, as well as addressing ethical considerations. In adaptation to these rapid changes, it is essential for society to engage in discussions about responsible AI development and deployment.
The future of Digital AI is not only about technology but also about its integration into the very fabric of society, fundamentally altering how we interact with the world.
Ultimately, the future directions of Digital AI can lead to noteworthy advancements that touch nearly every aspect of daily life. Stakeholders must grasp these innovations and prepare for the broader impact ahead.
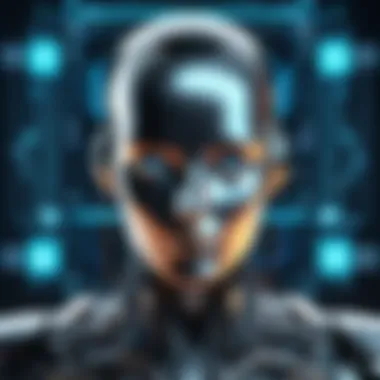
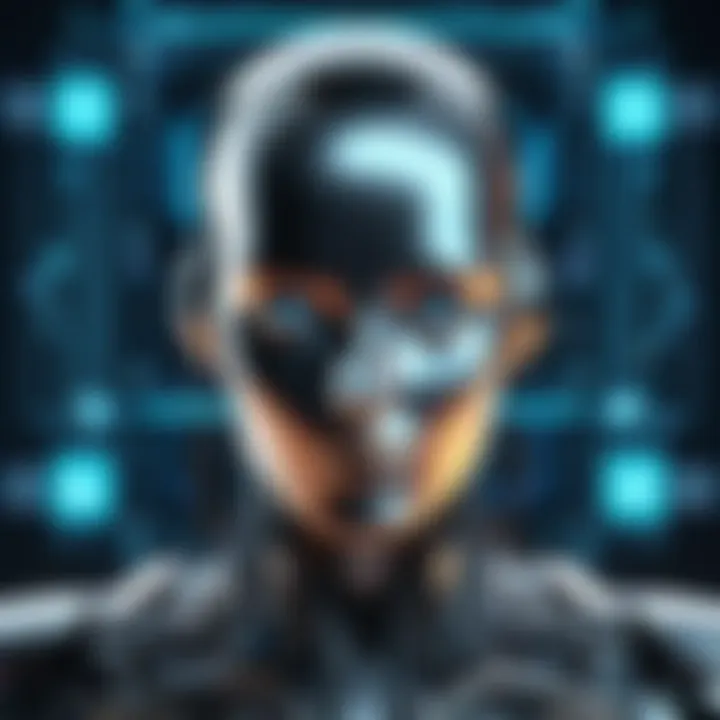
Challenges Faced by Digital AI
Understanding the complexities and obstacles surrounding Digital AI is essential for any business or professional looking to embrace its capabilities. As organizations implement AI solutions, they must grapple with various challenges that can hinder effectiveness. This examination focuses on two primary areas: technical limitations and regulatory hurdles. These aspects influence not only implementation strategies but also the overall efficacy of AI applications.
Technical Limitations
Digital AI solutions are often constrained by technical limitations that impact their performance and reliability. Despite significant advancements, there remain areas where technology struggles to meet expectations.
- Data Dependency: AI models require vast amounts of quality data to learn effectively. Insufficient data can lead to inaccurate predictions, limiting the AI’s potential. Moreover, noise within data sets can contribute to bias, leading to distrust among users.
- Computational Resources: High computational power is needed for the training of sophisticated models. Many organizations may not have access to sufficient hardware, which can stifle innovation,
- Lack of Standardization: As various industries adopt AI, the lack of unified standards complicates integration. Systems that work well in one domain might not translate effectively to another, creating additional challenges.
"Organizations often pay great attention to people but underestimate the technical backbone.
Regulatory Hurdles
Navigating the regulatory landscape poses another layer of challenges for Digital AI. As technology evolves rapidly, regulations often lag behind. Understanding the legal context of AI deployment is crucial for avoiding potential issues.
- Data Privacy Laws: Regulations such as GDPR in Europe dictate strict guidelines on data usage, requiring organizations to ensure compliance. Failure to adhere to these rules can result in hefty fines and reputational damage.
- Ethical Guidelines: There is an ongoing discussion about the ethical deployment of AI. Companies face pressure from stakeholders to align their technologies with ethical standards that are still evolving.
- Intergovernmental Regulations: Different countries have varying approaches to AI regulation, complicating international collaboration. This divergence can hinder innovation and create barriers to market entry for tech-focused businesses.
Addressing these challenges requires thorough research and proactive strategies, ensuring that Digital AI can fulfill its transformative potential. As experts and organizations navigate this complex landscape, understanding the implications of technical limitations and regulatory hurdles will play a pivotal role in the future development of AI technologies.
Integration of Digital AI in Existing Systems
Integrating Digital AI into current systems is a critical process for organizations aiming to enhance efficiency and competitiveness. As businesses strive to leverage AI's capabilities, understanding the integration aspect becomes pivotal. The ability to seamlessly blend AI technologies into existing frameworks can lead to improved decision-making, better customer experiences, and increased operational efficiency.
Best Practices for Implementation
When implementing Digital AI, several best practices should be followed to ensure success:
- Start Small: Focus on pilot projects that target specific business challenges. This helps in minimizing risk and provides a concrete example of AI benefits to stakeholders.
- Ensure Data Compatibility: Validate that the data infrastructure can support AI. Without compatible data, the effectiveness of AI initiatives will diminish.
- Involve Stakeholders Early: Engaging stakeholders during the planning phase increases buy-in and clarifies expectations from the outset. This is essential for aligning AI initiatives with business goals.
- Choose the Right Tools: Select AI tools and platforms that are flexible and can integrate with existing systems. For instance, technologies like IBM Watson and Microsoft Azure offer robust options that can be customized.
- Monitor Performance: Establish metrics to measure both the implementation process and the outcomes. Continuous assessment helps identify areas for improvement and ensures that the AI integration aligns with organizational objectives.
- Focus on User Experience: Think about how employees and customers will interact with the AI. Ensuring a user-friendly experience can lead to higher adoption rates.
Using these practices, organizations can navigate the complexity of digital AI integration more effectively.
Training and Skill Development
Training and skill development are also essential in facilitating the successful integration of Digital AI. Companies must ensure their workforce is well-equipped to handle new technologies. Without proper training, even the best AI systems may fail to realize their full potential. Here are some key points regarding training and skill development:
- Upskill Existing Staff: Provide ongoing learning opportunities for employees to build familiarity with AI technologies. Programs can range from basic AI concepts to advanced statistical methods, catering to various skill levels.
- Encourage Collaborative Learning: Foster a culture of knowledge sharing among teams. Collaboration can accelerate learning and help develop innovative solutions leveraging AI.
- Leverage Online Resources: Utilize platforms like Coursera and edX for courses specific to AI and machine learning. These accessible resources can help employees enhance their qualifications with minimal disruption to regular work duties.
- Hire Specialized Talent: Bringing in data scientists and AI specialists can bridge knowledge gaps and expedite implementation efforts.
- Integrate AI Literacy into Onboarding: New hires should receive training on how AI influences the business and their specific roles, ensuring a comprehensive understanding from the start.
The Role of Data in Digital AI
Data is the cornerstone of Digital AI. It provides the raw materials for AI systems to learn, adapt, and make decisions. The ability to collect and manage data effectively influences the success of AI initiatives across various sectors, including healthcare, finance, and marketing. Organizations that prioritize data as a key asset enhance their capacity to leverage AI technology. This section analyzes the importance of data in Digital AI, focusing on crucial elements such as data collection and management, as well as the ethical concerns that arise when handling this data.
Data Collection and Management
Effective data collection and management strategies are vital for developing robust AI systems. Without quality data, AI models may yield inaccurate or biased results. Businesses must adopt systematic approaches to gather data, ensuring it's relevant and diverse.
Factors to consider include:
- Source Variety: Utilizing multiple data sources, like customer interactions, sensor data, or social media, generates a comprehensive dataset.
- Data Quality: High-quality data is free from errors and inconsistencies. Organizations should employ methods to clean and preprocess data before it feeds into AI models.
- Scalability: The ability to manage large volumes of data is essential. As AI applications grow, streamlined processes for data storage, retrieval, and analysis are necessary.
- Real-time Management: Real-time data collection supports dynamic decision-making. This responsiveness can benefit applications such as fraud detection or personalized marketing strategies.
Nonetheless, the process of gathering and maintaining data is challenging. Lack of data governance can lead to data silos, where information remains unshared within the organization. A cohesive strategy for data management promotes collaboration across departments and enhances the value derived from data.
Ethics of Data Usage
With the power of data comes a set of ethical responsibilities. Organizations are tasked with ensuring that their data usage aligns with ethical standards. Privacy, consent, and transparency are critical factors in the ethical landscape surrounding AI.
Key points to consider:
- Data Privacy: Protecting user privacy is essential. Businesses must comply with regulations like the General Data Protection Regulation (GDPR), which enhances users' control over their personal data.
- Ethical Consent: Ensuring that users provide informed consent for data collection fosters trust and adheres to ethical norms. Obtaining explicit approval can mitigate backlash from the public against AI initiatives.
- Bias Mitigation: AI systems trained on biased data can perpetuate unfairness. Organizations should constantly audit datasets for bias, diversity, and representation to ensure fairness in AI-driven outcomes.
The bottom line is that while data powers Digital AI, its ethically responsible usage is equally important. Failing to address ethical considerations can damage brand reputation and lead to legal challenges.
"Data is not just an asset; it's also a responsibility. As AI continues to evolve, so must our approach to data ethics."
Digital AI in Education
Digital AI stands as a critical element in the realm of education, fundamentally altering traditional learning paradigms. It offers a wide array of applications that enhance both teaching and learning. The integration of AI technologies can lead to improved student engagement, personalized educational experiences, and administrative efficiency. As these technologies evolve, they illuminate the pathway for educational institutions to adopt innovative strategies that cater to diverse learning needs. Understanding these aspects can significantly impact educational outcomes and overall institutional success.
Personalized Learning Experience
Personalized learning powered by Digital AI tailors educational experiences to fit the individual needs of students. Through advanced algorithms and machine learning techniques, educational platforms can analyze student performance, learning styles, and interests. This data-driven approach provides targeted content, enabling students to progress at their own pace.
- Adaptive Learning Systems: These systems adjust the difficulty of lessons based on real-time assessment of student performance. For example, platforms like DreamBox Learning and Knewton utilize AI to modify educational content, ensuring that students face appropriate challenges without becoming overwhelmed.
- Data Analytics: By collecting and analyzing large data sets, educators can identify patterns in student learning. This insight facilitates understanding of where students struggle, allowing for timely interventions and support.
- Engagement Tools: AI can foster engagement through gamification and simulations. Programs that track user interaction patterns can suggest more engaging activities that suit a student's unique preferences.
Administrative Efficiency
Administrative tasks in education often consume valuable time and resources. Digital AI provides tools to streamline these processes, allowing educators to focus on what truly matters—teaching. By automating routine tasks, AI systems can enhance operational effectiveness within educational institutions.
- Automated Enrollment and Admissions: AI can simplify the registration process, ensuring accurate data capture and streamlined workflows. Systems such as Salesforce Education Cloud help institutions manage student relationships effectively.
- Scheduling: Through smart algorithms, AI can optimize scheduling for classes, exams, and even teacher assignments. This reduces conflicts and ensures more efficient use of facilities.
- Performance Tracking: Administrative personnel can utilize AI for efficient performance tracking of both students and faculty. By automating grading and feedback, the system saves time and improves the quality of evaluation.
Digital AI serves as an enabler for educational institutions, allowing them to operate more efficiently. This operational refinement ultimately enhances the learning environment, creating a more conducive atmosphere for education.
"Digital AI is not just a trend in education; it is a transformation that promises to redefine how learning is delivered and managed."
By embracing Digital AI, educational institutions can prepare to meet the demands of a rapidly changing landscape, ensuring they remain relevant in an increasingly digital world.
Digital AI in Finance
Digital AI is reshaping the finance sector with innovative solutions that enhance efficiency and accuracy. The adaptation of Artificial Intelligence technologies can optimize operations across various finance-related activities. This is particularly significant as this sector deals with vast amounts of data that require swift analysis and interpretation. Leveraging Digital AI helps organizations minimize risks, improve customer experiences, and streamline workflows.
Risk Assessment and Management
Risk management is a crucial element in finance, where the complexity and variability of financial markets can pose substantial challenges. Digital AI plays an essential role in enhancing risk assessment practices. By utilizing advanced machine learning algorithms, financial institutions can better predict market fluctuations and assess investment risks.
The benefits include:
- Improved Data Analytics: AI tools analyze historical data more efficiently than traditional methods. This allows for anticipating potential risks with greater accuracy.
- Real-time Monitoring: Digital AI enables continuous monitoring of financial metrics, leading to faster response times regarding potential risks.
- Scenario Analysis: Advanced simulations can be run to foresee various possible future states of the market, informing sound decision-making.
However, it is crucial to consider that while AI enhances risk assessment, it also introduces new complexities. The reliance on algorithms may lead to unforeseen consequences if not managed properly.
Fraud Detection Mechanisms
Fraud in finance can lead to significant financial loss and damage to reputations. Here, Digital AI serves as a proactive measure for fraud detection. Utilizing algorithms that analyze user behavior patterns, financial institutions can identify anomalies that may suggest fraudulent activities.
Key features include:
- Anomaly Detection: AI systems can learn from historical transactions to establish a baseline behavior. They can flag transactions that deviate from this established pattern.
- Machine Learning Models: These models adapt over time, learning from new data patterns, which continuously enhances their detection capabilities.
- Automated Alerts: AI can provide instant alerts when suspicious activities are detected, allowing for timely action.
In summary, the integration of Digital AI into the finance sector has profound implications for risk assessment and fraud detection. It enables organizations to act more swiftly and efficiently in a landscape that is constantly evolving. This is not just about adopting technology; it is about rethinking how the finance sector manages its most pressing challenges and leveraging digital tools for a more secure and resilient environment.
Digital Marketing and AI
Digital marketing paired with Artificial Intelligence (AI) emerges as a pivotal topic in the discourse surrounding modern business strategies. As businesses increasingly venture into the digital sphere, the integration of AI transforms marketing processes and practices, enabling organizations to achieve greater efficiency and effectiveness. The ability to utilize vast amounts of data allows marketers to enhance targeting precision, optimize campaigns, and drive conversions in ways previously unimaginable.
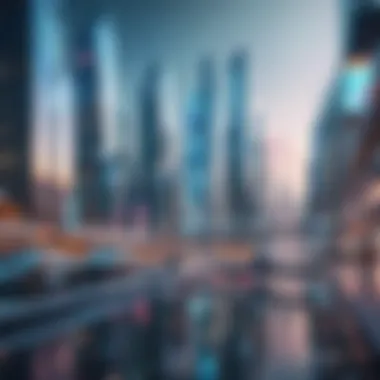
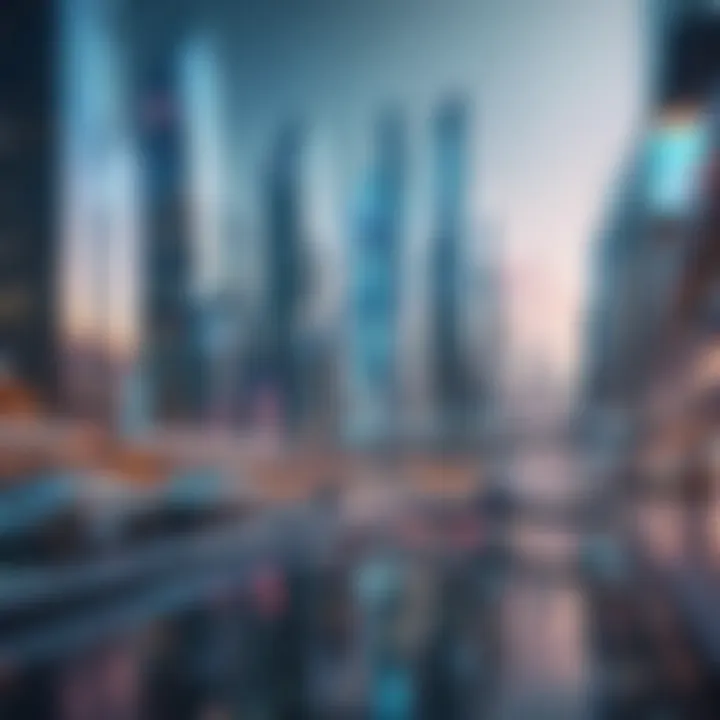
The benefits of leveraging AI in digital marketing are manifold. It aids in understanding consumer behavior, predicting trends, and personalizing communication at an unprecedented scale. AI's capacity for processing and analyzing data fosters a more nuanced approach to every aspect of marketing, from ad placement to customer engagement strategies. This not only improves customer satisfaction but also maximizes return on investment.
Despite the numerous advantages, several considerations must also be addressed. As marketers harness the power of AI, they must remain acutely aware of ethical implications, particularly regarding data privacy and user trust. Transparency in how data is collected and used is paramount; failing to uphold this can erode consumer confidence and lead to reputational harm.
Behavioral Targeting
Behavioral targeting is a strategy that employs AI algorithms to analyze consumer behavior patterns in order to deliver personalized advertising content. This technique focuses on understanding user intent through their online actions, enabling marketers to reach their audience with highly tailored messages.
The key advantages of behavioral targeting include:
- Increased Relevance: By catering to individual preferences, businesses can produce ads that resonate more effectively with potential customers.
- Higher Conversion Rates: Personalized content tends to yield better engagement, ultimately fostering higher conversion rates compared to traditional marketing approaches.
- Efficient Use of Resources: By directing efforts toward consumers who have shown interest, businesses can optimize their marketing budgets and reduce waste.
However, behavioral targeting also raises questions regarding privacy and consent. As companies analyze user data, it is crucial to strike a balance between personalized experiences and respecting consumer privacy. Organizations must ensure compliance with regulations while fostering transparent relationships with users to navigate this complex landscape successfully.
Content Creation Tools
AI’s role in content creation has evolved rapidly, providing tools that empower marketers and content creators to produce engaging material. These tools utilize machine learning and natural language processing to streamline the content development process, saving both time and resources.
Some notable applications of AI in content creation include:
- Automated Writing Tools: Platforms like Grammarly and Jarvis allow users to generate content based on input data or specific guidelines, assisting in crafting articles, blog posts, or social media updates.
- Data-Driven Insights: AI analyzes audience engagement to aid in developing content that appeals to specific demographics, ensuring that the output is not only relevant but also impactful.
- Optimization Tools: Solutions such as Yoast SEO provide guidelines on optimizing content for search engines, enhancing visibility and discoverability in an increasingly competitive landscape.
Nonetheless, while AI-generated content can enhance efficiency, it is essential to combine this technology with human creativity. The nuances of storytelling and emotional intelligence cannot be fully replicated by machines. Therefore, leveraging AI as a supportive tool rather than a complete replacement fosters a more balanced and effective content creation strategy.
"AI is not just about automation; it can enhance creativity and provide new tools for delivering value to consumers."
Marketers who embrace AI in their digital marketing efforts are taking proactive steps toward future-proofing their strategies. The interplay between digital marketing and AI encapsulates both promise and responsibility, highlighting the need for ethical considerations while navigating a data-rich landscape.
Decoding the AI Buzzwords
In an evolving technological landscape, understanding essential terminology is crucial for decision-makers and IT professionals. Buzzwords often emerge from advancements in digital AI, making it imperative to decode them effectively. This section aims to clarify these terms and their implications. Knowledge of key AI terms drives informed discussions and decisions, especially amid rapidly changing environments.
Understanding Key Terms
Multiple terms define functions and paradigms in digital AI. Some key terms include:
- Machine Learning (ML): A subset of AI focused on systems that learn from data to improve performance.
- Deep Learning: A more advanced area of ML involving neural networks with many layers, capable of analyzing large volumes of data.
- Natural Language Processing (NLP): The capability of machines to understand and interpret human language, vital for applications like chatbots and virtual assistants.
- Automation: The use of technology to perform tasks without human intervention; in AI, this often leads to efficiency gains.
- Predictive Analytics: Techniques that use historical data for predicting future outcomes, often applied in business and healthcare.
Understanding these terms establishes a foundation for discussing their applications and impacts.
Common Misconceptions
Despite the growing prevalence of AI, certain misconceptions persist. Addressing them helps clarify the role of digital AI in modern society:
- AI Equals Automation: Many believe AI solely embodies automation. However, AI encompasses a broader range of functions.
- AI Can Replace Human Intelligence: While AI can imitate certain tasks, it does not possess human-like reasoning or emotional understanding.
- More Data Always Means Better AI: While data quality is critical, it is not about quantity. Poor quality data can lead to misleading results.
- AI Is Infallible: AI systems can make errors. Relying solely on AI without human oversight can result in significant issues.
Understanding these misconceptions equips professionals to make better-informed decisions when implementing AI tools.
Case Studies of Digital AI Implementation
The case studies of digital AI implementation provide significant insights into the practical applications and the impact of these technologies across various sectors. Analyzing these examples not only underscores the successful adoption of AI but also highlights the potential pitfalls companies may encounter. These case studies deliver a dual perspective, enabling organizations to understand both triumphs and challenges faced by early adopters of digital AI.
Focusing on the importance of real-world applications, case studies demonstrate how businesses leverage AI technologies to improve efficiency, drive innovation, and enhance customer satisfaction. Each narrative showcases distinct strategies and their respective outcomes. Thus, they serve as a rich resource for decision-makers and entrepreneurs aiming to integrate AI into their operations and develop competitive advantages in the marketplace.
There are particular elements to consider in this analysis of case studies:
- Sector-specific insights: Each industry's unique challenges and opportunities shape the implementation of digital AI. This allows for tailored strategies that optimize outcomes.
- Scalability: Observing how different organizations scale their AI initiatives provides lessons on growth and adaptation.
- Return on investment: The financial results of AI implementation often reveal the feasibility and economic viability of adopting these technologies.
By studying both success stories and failures, businesses can gain a comprehensive view of what works in leveraging AI effectively. The lessons gleaned from these narratives can guide future endeavors, instilling a profound understanding of the intricacies involved in AI integration.
Success Stories from Various Industries
Several success stories exemplify how digital AI has reshaped industries and propelled organizations toward their goals. For instance, in the healthcare sector, IBM Watson has made significant strides in diagnostic accuracy and treatment recommendations, leading to better patient outcomes. Healthcare professionals now have access to vast data analysis tools, improving decision making in patient care.
In retail, Amazon adeptly uses AI algorithms for inventory management. This allows for real-time tracking and optimization, greatly enhancing supply chain efficiency. As a result, Amazon reduces operational costs and improves customer experience through quicker fulfillment.
Within the finance domain, Ant Financial, a Chinese fintech company, has implemented AI systems to conduct risk assessments. This helps in identifying potential fraud patterns and preventing financial crimes, thus protecting customers and strengthening confidence in digital transactions.
These successes illustrate the transformative potential of digital AI across diverse fields. They establish benchmarks for best practices while demonstrating how innovative technologies can create substantial value.
Lessons Learned from Failed Projects
Despite the numerous success stories, several digital AI projects have faced challenges, yielding lessons crucial for future implementations. A notable example is the failed attempt by Google's AI ethics board to create an independent group for overseeing AI developement. The project fell apart due to internal conflict and a lack of clear guidelines, exposing weaknesses in governance structures.
Another cautionary tale is Microsoft's Tay, an AI chat bot that descended into offensive and inappropriate language shortly after launch. This highlighted critical issues with data bias and the need for effective monitoring mechanisms. Organizations can learn from Tay's failure by ensuring robust safeguards are in place before deploying AI systems to the public.
Additionally, many enterprises have met challenges when attempting to scale digital AI operations. For instance, a large financial institution ventured to implement an AI-driven customer service system, only to find it incompatible with existing IT infrastructure. Such cases emphasize the importance of thorough planning and alignment of new technologies with existing frameworks.
By understanding these failures, businesses can better prepare themselves to navigate the complexities of AI implementation. Lessons learned serve as essential guidelines for achieving successful integration in the future.
"The success or failure of digital AI projects ultimately influences how organizations will leverage these technologies in upcoming initiatives."
The Economic Impact of Digital AI
The economic implications of Digital AI extend beyond mere technological adoption. The integration of artificial intelligence into various sectors has been reshaping traditional economic frameworks. As businesses leverage AI capabilities, they unlock unprecedented opportunities for growth, efficiency, and innovation. Understanding this impact is crucial for professionals and decision-makers who wish to navigate the evolving landscape of the digital economy.
Job Creation vs. Job Displacement
A primary concern regarding Digital AI revolves around its dual potential to create jobs while displacing existing ones. The narrative often leans towards a pessimistic view, highlighting the risk of automation leading to job losses. However, an analysis reveals a more nuanced reality.
Digital AI fosters innovation, leading to the emergence of entirely new job categories. Roles such as AI specialists, data scientists, and AI ethicists are gaining prominence. Traditional industries are also adapting; for example, sectors like finance and healthcare require new positions focused on managing AI systems and interpreting data output.
Yet, this job creation comes with a caveat. Jobs that involve repetitive and manual tasks are at higher risk of automation. Workers in these roles may need reskilling or upskilling to transition into new employment opportunities. Therefore, balancing the benefits of job creation with the reality of job displacement requires strategic planning and investment in workforce development.
Implications for Global Markets
The integration of Digital AI into global markets alters competitive dynamics and reshapes trade. Countries that successfully adopt AI technologies enhance their economic competitiveness. They tend to experience increased productivity, lower operational costs, and an improved ability to respond to market demands.
Moreover, AI's capability to analyze vast datasets allows companies to identify trends quickly and respond swiftly to changing consumer preferences. This agility can lead to a more resilient economy that adapts to shocks better than before.
On the downside, disparities may emerge. Nations lagging in AI adoption could face economic challenges, exacerbating existing inequalities. Companies reliant on outdated technologies may find it hard to compete, leading to potential economic fragmentation.
"Countries that lead in AI adoption will not only dominate their respective markets but will also shape the future of global economic structures."
In summary, the economic impacts of Digital AI are profound. As professionals continue to explore AI applications, understanding these economic factors becomes critical for informed decision-making and strategic planning.
Epilogue: Navigating the Future of Digital AI
Understanding the future of Digital AI is crucial for organizations looking to remain competitive in an increasingly digital landscape. This section synthesizes the insights provided throughout the article and examines the potential pathways for adopting Digital AI effectively.
Digital AI's transformative potential offers significant opportunities for advancement in various sectors. These opportunities include enhanced efficiency, improved decision-making, and innovative solutions to pressing problems. With the increased capability to process vast amounts of data, organizations can leverage Digital AI to gain insights that were previously unattainable. The importance of being proactive in adopting AI technologies cannot be overstated.
Final Thoughts on Digital AI
Digital AI stands at the intersection of technology and human potential. It is imperative for decision-makers and IT professionals to grasp its significance not just as a technical tool, but as a catalyst for change.
As organizations look toward future directions, here are several key considerations that emerge:
- Continuous Learning: Embracing Digital AI requires a commitment to learning and adaptation. The evolving nature of AI technologies means that ongoing training for personnel is essential.
- Ethical Responsibility: Companies must prioritize ethical considerations in AI utilization. This includes addressing issues of bias, fairness, and privacy. A transparent approach builds trust with stakeholders and users.
- Strategic Investments: Investing in the right AI technologies can drive significant returns. It's important to be strategic, focusing on initiatives that align with business goals while keeping flexibility to adapt to newer innovations.
- Collaborative Ecosystems: Collaboration across sectors can yield innovative solutions. Engaging in partnerships with other firms, research institutions, or even local communities can enhance the AI development process.