Unleashing the Potential: Power BI Predictive Analytics Decoded
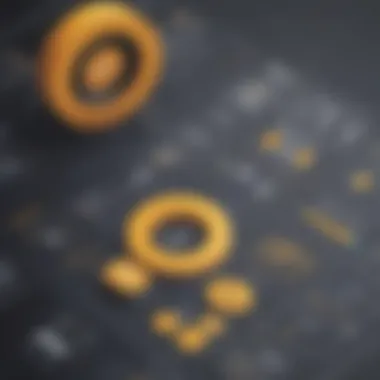
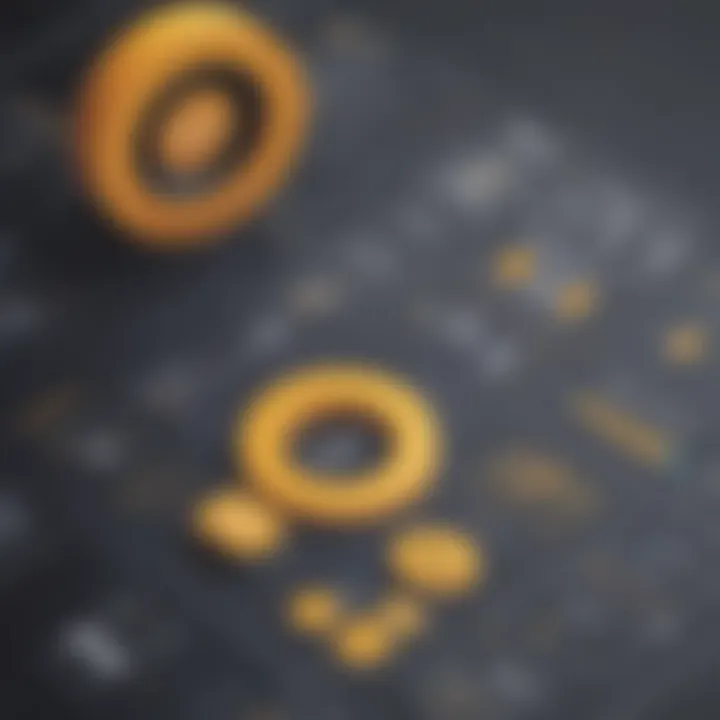
Industry Overview
The realm of predictive analytics within Power BI is witnessing a surge in interest and utilization from various industries π. As organizations delve deeper into the realm of data-driven decision-making, leveraging the power of predictive analytics has become a cornerstone for success. Understanding the current trends in industry-specific software markets is pivotal for harnessing the true potential of Power BI Predictive Analytics. Key challenges faced by buyers in this competitive landscape include data security, accuracy of predictions, and the integration of predictive insights into existing workflows. Emerging technologies like machine learning and artificial intelligence are leaving a significant impact on industry practices, paving the way for more efficient and proactive decision-making processes.
Top Software Options
When delving into the realm of Power BI Predictive Analytics, it is essential to review and compare leading software providers in the industry π. Conducting a thorough feature comparison of top software solutions allows businesses to identify the tools best suited to their unique needs and objectives. Analyzing the pricing structures of different software options is crucial in determining the most cost-effective and value-added solution for predictive analytics implementation.
Selection Criteria
Selecting the right software for predictive analytics implementation necessitates careful consideration of important factors π‘. Common mistakes during the selection process, such as overlooking scalability or compatibility issues, can hinder the effectiveness of predictive analytics initiatives. Determining the perfect fit for your business needs requires a strategic approach, focusing on factors like predictive accuracy, ease of integration, and scalability for future growth.
Implementation Strategies
Executing a seamless implementation strategy for Power BI Predictive Analytics is paramount for maximizing the benefits of the software solution π. Best practices entail aligning the implementation process with existing systems and processes, ensuring a smooth transition and minimal disruptions. Integration of predictive analytics tools with current workflows is essential for extracting meaningful insights and driving informed decision-making. Providing adequate training and support resources to employees facilitates successful deployment and adoption of predictive analytics within the organization.
Future Trends
Looking ahead at the future of the industry-specific software landscape reveals a continuum of innovation and advancement π. Predictions foresee the integration of cutting-edge technologies into predictive analytics solutions, amplifying the accuracy and speed of insights generation. Upcoming technologies, such as advanced analytics algorithms and enhanced visualization capabilities, are set to redefine the industry's approach to data-driven decision-making. Recommendations for future-proofing your technology stack include staying abreast of emerging trends, fostering a culture of innovation, and proactively adopting new technologies to stay ahead in the competitive business landscape.
Introduction to Predictive Analytics
Predictive analytics sits at the crux of data-driven decision-making, propelling businesses towards informed strategies and actions. In this comprehensive guide on Power BI Predictive Analytics, we delve deep into the transformative capabilities of harnessing data to predict future trends and outcomes effectively. By understanding the essence of predictive analytics, businesses can uncover valuable insights that revolutionize their operational paradigms.
Understanding Predictive Analytics
Definition and Significance
The bedrock of predictive analytics lies in its ability to analyze historical data, extract patterns, and foresee potential outcomes with a significant degree of accuracy. This paramount feature enables organizations to make proactive decisions based on data-driven projections rather than mere speculations. The importance of this predictive model lies in its precision in anticipating trends, behavior, and events, providing a competitive edge in dynamic markets. By elucidating key concepts embedded in predictive analytics, businesses can fortify their decision-making processes and set a robust foundation for sustainable growth.
Key Concepts and Techniques
Navigating through the landscape of predictive analytics unveils a multitude of key concepts and techniques that underpin its efficacy. From regression analysis to time series forecasting, each technique offers distinct advantages in transforming raw data into actionable insights. Understanding these methodologies empowers analysts to uncover hidden patterns, correlations, and anomalies within datasets, thereby enhancing predictive accuracy and strategic planning. Leveraging these key concepts and techniques within Power BI equips organizations with the tools to optimize their predictive analytics workflows and elevate their business intelligence strategies.
Benefits of Predictive Analytics
Enhanced Decision-Making
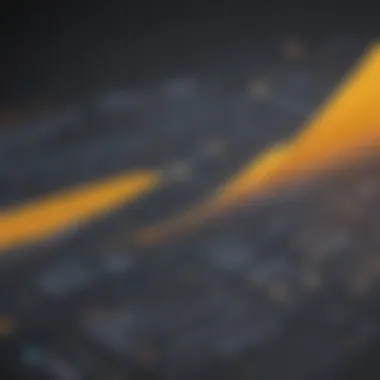
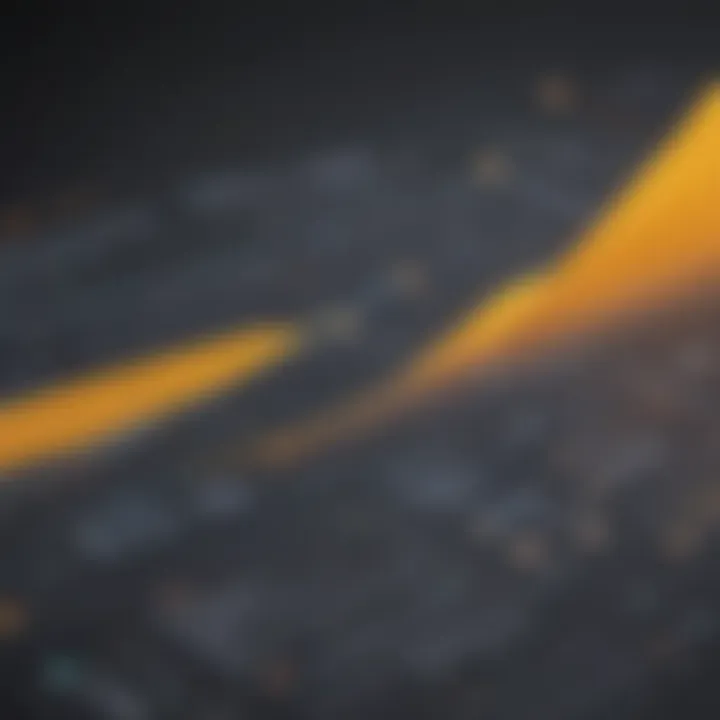
The integration of predictive analytics augments decision-making processes by providing a data-driven approach to problem-solving. By leveraging predictive models, organizations can forecast customer behavior, market trends, and operational outcomes, facilitating proactive and strategic decisions. This proactive stance not only mitigates risks but also maximizes opportunities, fostering a culture of informed decision-making across all business functions.
Improved Strategic Planning
In today's competitive landscape, strategic planning is imperative for sustainable growth and market relevance. Predictive analytics plays a pivotal role in shaping strategic initiatives by offering insights into future trends, potential risks, and optimal paths for growth. By incorporating predictive analytics into strategic planning frameworks, organizations can adapt swiftly to market dynamics, capitalize on emerging opportunities, and mitigate potential threats effectively. This proactive strategic approach enabled by Power BI Predictive Analytics propels businesses towards agility and resilience in volatile business environments.
Power BI Overview
Power BI plays a crucial role in the realm of predictive analytics, offering a robust platform for data analysis and visualization. In this article, we delve into the intricacies of Power BI, shedding light on its significance in revolutionizing data-driven decision-making processes.
Introduction to Power BI
Features and Capabilities
Power BI sets itself apart with its extensive range of features and capabilities that empower users to extract valuable insights from complex data sets. The interactive visualizations, drag-and-drop functionality, and real-time dashboards are key elements that contribute to the platform's effectiveness in data analysis. Its innate ability to handle large volumes of data while ensuring swift performance makes it a preferred choice for businesses looking to streamline their analytics processes.
Integration with Microsoft Ecosystem
One of Power BI's strengths lies in its seamless integration with the broader Microsoft ecosystem. This connectivity enables users to leverage existing Microsoft tools and services, such as Azure cloud services and Excel, enhancing the overall analytical experience. The cohesive integration not only facilitates data sharing across different platforms but also ensures compatibility with familiar tools, boosting productivity and reducing learning curves.
Power BI Predictive Analytics
Machine Learning in Power BI
Machine learning within Power BI opens new avenues for predictive analytics, allowing users to harness advanced algorithms to forecast trends and make informed decisions. The platform's machine learning capabilities empower users to build and deploy predictive models without the need for extensive coding knowledge. This accessibility to predictive analytics enhances data interpretation and drives actionable insights, making it a valuable asset for organizations seeking to stay ahead in a data-driven landscape.
Predictive Modeling Tools
Power BI's predictive modeling tools offer a user-friendly interface for creating and fine-tuning predictive models based on historical data patterns. From regression analysis to classification algorithms, these tools enable users to uncover hidden patterns within their datasets, facilitating accurate predictions and strategic planning. The intuitive nature of these tools simplifies the modeling process, enabling even non-experts to delve into the realm of predictive analytics with confidence.
Implementing Predictive Analytics with Power BI
In the realm of data-driven decision-making, implementing predictive analytics with Power BI holds paramount significance. This section delves into the essential steps and aspects involved in leveraging Power BI for predictive analytics. By utilizing Power BI tools for predictive analytics, organizations can unlock a wealth of benefits in terms of refined insights and informed strategic decisions. The process of implementing predictive analytics with Power BI entails meticulous data preparation and building robust predictive models to drive actionable outcomes.
Data Preparation
Data Cleaning and Transformation
Data cleaning and transformation play a pivotal role in the realm of predictive analytics with Power BI. This process involves refining raw data sets by identifying and rectifying inaccuracies, inconsistencies, and missing values. The concerted effort in cleaning and transforming data is crucial as it ensures the accuracy and reliability of the predictive models built upon it. The meticulous nature of data cleaning and transformation is imperative for enhancing the efficacy of predictive analytics processes within Power BI.
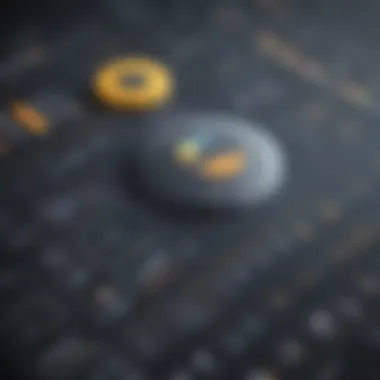
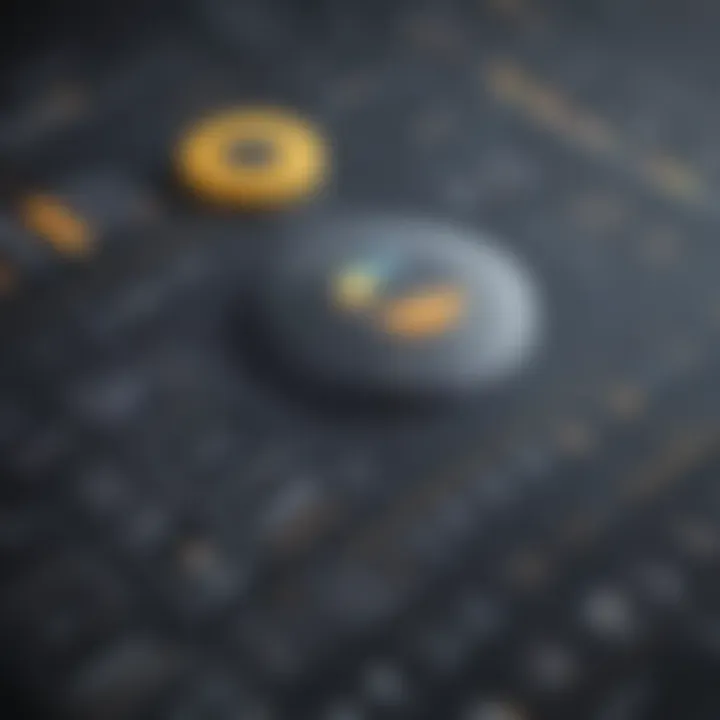
Feature Engineering
Feature engineering is a cornerstone of predictive analytics with Power BI, focusing on selecting and optimizing relevant data features for model development. This process involves extracting meaningful insights from the data through statistical analyses and domain expertise. By strategically engineering features, organizations can enhance the predictive capabilities of their models and improve the accuracy of forecasts. Feature engineering stands as a fundamental practice in optimizing the performance of predictive analytics workflows within Power BI.
Building Predictive Models
Regression Analysis
Regression analysis serves as a fundamental predictive modeling technique within Power BI, aiming to establish relationships between variables and predict outcomes based on historical data patterns. This method leverages statistical algorithms to estimate the impact of one or more variables on the target variable, facilitating precise decision-making processes. Regression analysis empowers organizations to forecast trends, understand correlations, and make informed predictions, thereby augmenting the predictive analytics capabilities of Power BI.
Classification Algorithms
Classification algorithms are instrumental in predictive modeling with Power BI, enabling organizations to categorize data points into distinct classes based on predefined parameters. These algorithms, including decision trees, support vector machines, and neural networks, classify data efficiently to facilitate predictive insights and pattern recognition. By leveraging classification algorithms, organizations can streamline data classification tasks, enhance data interpretation, and optimize decision-making processes within Power BI.
Optimizing Predictive Analytics Workflows
In exploring the realm of Optimizing Predictive Analytics Workflows, this article delves into the critical strategies that can drive efficiencies and effectiveness in data analysis processes. Optimization is a key aspect in ensuring that predictive analytics models deliver accurate and actionable insights. By streamlining workflows, organizations can enhance their decision-making capabilities, identify trends more efficiently, and gain a competitive edge in their respective industries. The importance of optimizing these workflows cannot be overstated, as it directly impacts the quality and reliability of the predictive models generated within Power BI.
Visualization and Interpretation
Interactive Dashboards:
Interactive Dashboards play a pivotal role in providing users with real-time and customizable data visualizations. These dashboards enable seamless exploration of complex datasets, empowering users to interact with the data and extract meaningful insights. The interactive nature of these dashboards fosters data-driven decision-making by allowing users to drill down into specific data points and trends. This feature enhances user engagement and facilitates a deeper understanding of the underlying data. While Interactive Dashboards offer unparalleled flexibility and interactivity, they require careful design and structuring to ensure that the information presented aligns with the user's objectives and preferences.
Insights Generation:
Insights Generation encapsulates the process of deriving actionable and valuable insights from the data visualizations and analyses generated within Power BI. This aspect focuses on identifying patterns, trends, and anomalies within the data that can inform strategic decision-making. By harnessing advanced analytics tools and algorithms, businesses can uncover hidden insights that may not be apparent through traditional data analysis methods. Insights Generation plays a crucial role in transforming raw data into actionable intelligence, enabling organizations to uncover new opportunities, mitigate risks, and optimize their operations. While this process is instrumental in driving informed decision-making, it requires a nuanced approach to ensure that the generated insights are accurate, relevant, and actionable.
Performance Monitoring
Model Evaluation Metrics:
Model Evaluation Metrics serve as the yardstick for assessing the performance and accuracy of predictive models developed within Power BI. These metrics provide quantifiable measures of a model's predictive capabilities, including accuracy, precision, recall, and F1 score. By evaluating these metrics, data scientists and analysts can gauge the efficacy of their models and identify areas for improvement. Model Evaluation Metrics play a crucial role in validating the reliability of predictive models, ensuring that they meet the desired objectives and deliver actionable insights. While these metrics offer valuable insights into model performance, it is essential to interpret them judiciously and consider them in conjunction with the business context and domain expertise.
Continuous Improvement Strategies:
Continuous Improvement Strategies encompass a set of iterative processes aimed at refining and enhancing predictive analytics workflows over time. These strategies revolve around leveraging feedback, performance metrics, and emerging technologies to fine-tune models and methodologies. By adopting a continuous improvement mindset, organizations can adapt to evolving business dynamics, seize new opportunities, and mitigate potential risks. Continuous Improvement Strategies play a pivotal role in driving innovation and excellence in predictive analytics, ensuring that organizations stay ahead of the curve in a rapidly changing landscape. While embracing these strategies is essential for staying competitive, organizations must strike a balance between innovation and stability to maximize the value derived from their predictive analytics initiatives.

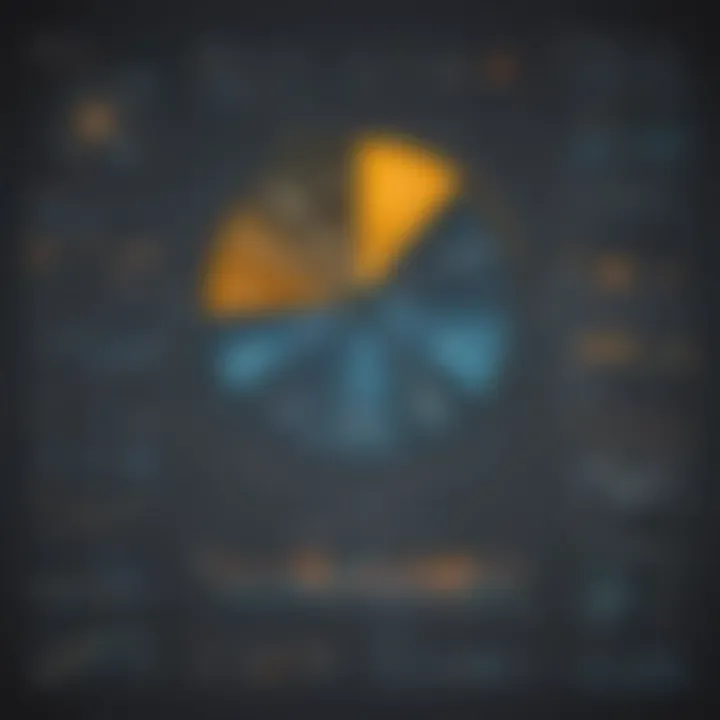
Real-World Applications and Case Studies
Real-world applications and case studies play a pivotal role in understanding the practical implications of predictive analytics. They serve as tangible examples of how businesses can leverage Power BI Predictive Analytics to drive informed decision-making and enhance operational efficiency. By examining real-world scenarios, readers can grasp the relevance and significance of integrating predictive analytics tools like Power BI into their organizational processes. Through in-depth analysis of industry use cases, this section delves into the diverse applications of predictive analytics across different sectors, illustrating the transformational impact of data-driven insights on business outcomes.
Industry Examples
Retail and E-Commerce
Finance and Banking
Finance and banking institutions benefit greatly from predictive analytics embedded within Power BI. By utilizing predictive models, these sectors can enhance risk management, detect fraudulent activities, and tailor financial products to customer needs. The key characteristic of finance and banking lies in the precision and speed required for decision-making, where predictive analytics algorithms provide invaluable insights for minimizing uncertainties and optimizing operational processes. Despite its advantages, the sector faces concerns regarding data privacy and regulatory compliance when implementing predictive analytics solutions.
Success Stories
Increased Revenue Streams
The focus on increasing revenue streams through predictive analytics signifies a strategic shift towards data-driven revenue optimization strategies. By employing Power BI for revenue forecasting and identifying growth opportunities, businesses can make informed decisions that amplify profitability and market competitiveness. This unique feature emphasizes the role of predictive analytics in empowering businesses to capitalize on market trends and customer behavior patterns, enabling proactive decision-making based on predictive insights. However, businesses must ensure the scalability and sustainability of revenue generation models to realize long-term financial gains.
Enhanced Customer Experiences
Enhancing customer experiences through predictive analytics revolutionizes how businesses interact with their clientele, tailoring products and services to meet evolving expectations. Power BI facilitates the analysis of customer insights, allowing organizations to personalize offerings, improve service quality, and foster lasting customer relationships. The significant characteristic of enhanced customer experiences lies in the ability to predict customer needs and preferences accurately, leading to higher satisfaction levels and brand loyalty. Despite its advantages, organizations need to address data security concerns and ethical considerations when personalizing customer experiences through predictive analytics.
Future Trends and Innovations
In this pivotal section on Future Trends and Innovations, we delve into the cutting-edge advancements shaping predictive analytics through Power BI. By exploring how technology evolves, we gain insights into the disruptive potential of AI integration and enhanced automation. These trends are instrumental in driving organizations towards data-driven excellence, propelling decision-makers to embrace innovative strategies and tools.
Advancements in AI Integration
AI-Powered Predictive Analytics
Embarking on the realm of AI-Powered Predictive Analytics, we unlock a realm of predictive capabilities that redefine data analysis. The distinctive feature of leveraging artificial intelligence to enhance predictive analytics offers a revolutionary approach to understanding trends and making proactive decisions. The efficiency and accuracy bolster decision-making processes, positioning AI-Powered Predictive Analytics as a cornerstone in the digital transformation journey of enterprises.
Deep Learning Integration
Delving deeper, Deep Learning Integration brings forth a sophisticated layer of predictive insights into the data analytics landscape. Its core characteristic lies in its ability to decipher complex patterns and correlations within large datasets, fostering a deeper understanding of business dynamics. The unique feature of self-learning enables the system to adapt and improve autonomously, contributing to the agility and precision of predictive analytics. However, challenges may arise in the intensive computational requirements and the need for specialized skill sets to maximize its potential.
Enhanced Automation
Streamlined Processes
Within the realm of Streamlined Processes, the focus shifts towards optimizing and automating data workflows to streamline operations. The key characteristic of seamless automation lies in its ability to enhance efficiency, reduce errors, and accelerate decision-making processes. By automating repetitive tasks and standardizing processes, organizations can free up resources for strategic initiatives, driving productivity and innovation.
Predictive Maintenance Solutions
Exploring Predictive Maintenance Solutions illuminates a paradigm shift in how organizations approach asset management and operational sustainability. The pivotal feature of predictive maintenance lies in its proactive approach to identifying potential issues before they escalate, minimizing downtime and optimizing resource allocation. Implementing predictive maintenance empowers organizations to move from reactive to proactive maintenance strategies, ensuring equipment reliability and operational continuity.